DOWNLOAD
14 min read •
Risk: Strengthening business resilience after COVID-19
Building a “sense and respond” approach using new predictive analytics tools
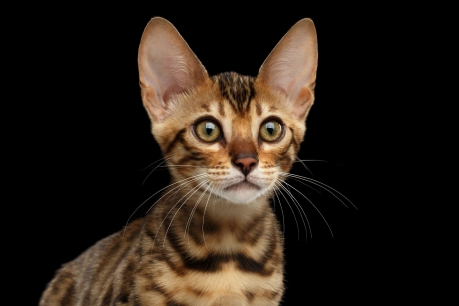
Despite that they had invested in comprehensive crisis management planning, the spread of COVID-19 found many companies ill-prepared, even though it was a known risk with extreme consequences and a reasonable likelihood of occurring. Looking at the evidence, the authors consider the underlying causes of this poor preparedness and set out the key elements of a new business resilience approach suitable for the post- COVID-19 world.
When COVID-19 suddenly escalated from a regional crisis in China to a pandemic, in companies around the world executives rapidly started double-clicking on their crisis management and emergency-response plans. For some, especially those with significant Asian operations, there was already a plan to respond, while for others, the term “pandemic” returned a blank. So began an intensive period of almost continuous back-to-back virtual meetings as leadership teams attempted to regain control of their business operations. Their immediate priorities were securing employee and customer safety and health, followed by maintaining operational continuity, managing cash, helping suppliers, coordinating with governments, engaging with communities, looking towards the recovery phase and, through all of this, continuous intensive communications.
Most companies, as well as governments, quickly realized that they were not well prepared. The breathtaking speed with which the crisis unfolded meant companies had to improvise, because the processes set out in their crisisresponse plans were simply too rigid and slow.
Many found that their plans had not considered the challenges of having to make rapid decisions with incomplete information, and many had underestimated the efforts needed to coordinate across complex external partner ecosystems. Initially, there were huge shortages of basic provisions such as appropriate protective equipment.
Yet, a virus pandemic such as COVID-19 was not at all unexpected. The world had already had a stark warning during the SARS outbreak in the early 2000s, and the risk of similar events was discussed in numerous conferences and panels, including two pandemic tabletop exercises by the John Hopkins Center for Health Security in 2018 and 2019. Bill Gates famously warned about the potential consequences of a virus pandemic in a TED Talk in 2015.
So, if we had a known risk with extreme consequences and a reasonable likelihood of occurring in the medium term, why did governments and companies do so little to invest in the necessary control and response measures? What does this tell us about how companies should go about improving their business resilience? In this article we consider some of the underlying causes of the poor preparedness, and set out the key elements of a new business-resilience approach suitable for the post-COVID-19 world.
Why were we not better prepared?
Major crises with global impact occur regularly. For example, in the last 20 years we have seen, among many others, 9/11, Deepwater Horizon, Fukushima and the 2008/9 financial crash. After every major crisis event there has been an in-depth investigation and analysis. In nearly all cases the conclusion was that there had been weaknesses or errors in how emerging issues had been recognized and dealt with. New controls and/or regulations were then put in place to prevent similar events from happening again – think of changes to air travel after 9/11, changes to banking regulations after the financial crash, and nuclear power policies after Fukushima. Of course, we can expect similar, and probably even greater, changes in the wake of COVID-19. This means next time we have a global crisis that looks like COVID-19, we will be a lot better prepared.
The problem is, of course, that the next global crisis probably won’t look exactly like COVID-19. It could be a different sort of pandemic or an entirely different sort of crisis altogether, such as a cyber-security or environmental crisis, both of which are already well recognized on corporate risk registers.
So why are companies in general still reluctant to invest in controls for catastrophic events that are already recognized but may or may not happen in the medium term? We can identify some common underlying reasons:
- The need to feel it to believe it: It is sometimes said that people do not learn from history. It is perhaps more accurate to say people learn from their own history, but not so well from someone else’s. The countries that were prepared for COVID-19 were the ones that had been through SARS. After a catastrophe, it’s usually not so difficult, with the benefit of hindsight, to find at least one or two prior pieces of evidence or warnings that could have been better heeded. However, in the period before the catastrophe these warnings are often lost in the noise with all the other warnings about potential catastrophes that may or may not happen. So, it is perhaps no wonder leaders do not always take action. Hindsight can be a deceptive tool.
- The “boiling frog” problem: This metaphor (a fable that suggests a frog may fail to jump out of a pan of water as it slowly heats up) refers to the poor ability of humans to take action in the case of threats that build gradually rather than appear suddenly. It is often used in connection with the threat of climate change, but could equally apply to the early phases of COVID-19. The problem here is that most organizations have not done enough to develop clear thresholds for risk tolerance (part of a wider concept sometimes referred to as “risk appetite”). In other words, there are no clear criteria to trigger action in the case of a gradually deteriorating situation that causes a risk to reach a predetermined threshold. Without these clear risk tolerance thresholds, organizations tend, like the frog, to do nothing until it’s too late.
- The pressures of the short term: Governments and business leaders alike tend to be judged over timescales of a few years at most. The average tenure of a CEO has been falling steadily over the last 20 years to no more than five or six years, and governments stand or fall based on their performances between elections. Catastrophic risks tend to be infrequent (high impact, low likelihood), and it is therefore often attractive to park or postpone preparations for them, especially given more pressing short-term priorities and the demands of shareholders or the electorate.
- The difficulties of investment prioritization: In theory, prioritizing investments in risk management is straightforward: for each risk, calculate the expected loss over an agreed period by multiplying its impact by its likelihood of occurring. The value of the “averted loss” through investing in risk-control measures is then compared to the costs of those measures. In practice, however, this is often not enough to prompt boards to invest large sums of money to control major catastrophic risks. Firstly, the calculation usually involves a series of modeled assumptions which are often easy to challenge. Secondly, the sums of money involved in major risk control are often significant, so the intervention may get deprioritized when compared to other risks which may be lower impact but more likely to occur.
- The “can-do” mentality trap: Management cultures typically value leadership traits such as positivity, dynamism, ambition and entrepreneurship. Indeed, all these qualities are important for good leaders. However, in many organizations the corollary of this is that traits such as caution, attention to detail, and concern for what could go wrong are not valued, or even sometimes discouraged, in top leaders. Although consideration of what could go wrong and how to respond should be an integral part of any strategy, in practice these are often perceived as negative or pessimistic topics. Consequently, they are often passed down to risk management functions and treated more as unavoidable red tape and overhead than as value-adding activities for the business.
Moving towards a more resilient business
These causes of poor resilience to major crises are fundamental and rooted in basic human behavior. Although some may berate leaders for their short-sightedness and lack of vision, shouting more loudly and introducing more controls and procedures is unlikely to be the solution.
To make matters worse, the vulnerability of the world to global crises has increased significantly due to increased global connectivity. For example, Nassim Nicholas Taleb, the originator of the Black Swan concept, is quoted in a recent interview with the New Yorker as saying, “The great danger has always been too much connectivity.” The interview goes on to highlight that “proliferating global networks, both physical and virtual, inevitably incorporate more fat-tail risks into a more interdependent and ‘fragile’ system…” The COVID-19 crisis is an all-too-real illustration of the problem. So, in the post-COVID-19 world it is also reasonable to assume that there will be:
- Greater likelihood of local risks escalating globally.
- Higher velocity of escalation of those risks.
- More interconnections between risks – for example, COVID-19 has already led to an increase in cyber-attacks due to the numbers of people working at home.
If we accept that we can’t predict the future just by reviewing the past, and if the fragility of our global systems has increased, the key thing we need from our business resilience system is to sense what is happening in real time, constantly update our predictions, and allow us to take early action before a major risk escalates.
This requires a much more dynamic and adaptive approach than has been traditionally used in conventional static enterprise risk management (ERM) systems. A major shift in philosophy is needed, as shown in Figure 1:
To achieve this shift towards a “sense and respond” philosophy, organizations need to evolve beyond conventional risk-register-based ERMs. Three aspects are key to making this evolution (see Figure 2):
Forward-facing practices: In a sense-and-respond business resilience system the emphasis is changed from rear-facing monitoring and review (such as incidents and losses) towards forward-facing prediction. Using a mix of lagging and leading risk indicators is nothing new, but usually the chosen leading risk indicators (for example, proportion of audits passed successfully or risk training provided) say very little about emerging risks or increasing threats.
The key capability needed for an effective forward-facing approach is the ability to develop realistic and robust causeeffect models. This can be challenging in practice for a complex global operation, especially in view of the high degree of connectedness organizations have within their partner ecosystems, but it is possible and worth spending time on. Once the cause-effect models have been developed, it becomes possible to establish customized and aggregated leading key risk indicators (KRIs). KRIs need to be calibrated to provide a “red flag” prior to a risk event occurring, with this calibration directly related to the organization’s risk tolerance levels.
An example could be a composite KRI relating to supplier defaults in one part of a complex global supply chain, which could provide early warnings of major disruptions further down the line. An effective forward-facing approach also requires an effective horizon-scanning or foresighting capability to identify emerging risks. Often these capabilities are present in companies, but focused on innovation or new product development, and therefore disconnected from corporate risk or business resilience functions. Fortunately, new data-analytics approaches and artificial intelligence (AI) and machine learning (ML) technologies are now becoming available to enable much easier cause-effect analysis, horizon scanning, detection of weak signals, and real-time KRI monitoring.
Dynamic prioritization: Being able to regularly “retune” risk-control priorities to take account of emerging risks is the essence of being dynamic. For this, understanding risk velocity – how quickly an organization will feel the impact of a risk event occurring – is key. Modern data-analytics tools now enable potentially high-velocity emerging risks to be identified more easily and monitored in real time through KRIs.
Adaptive response: Finally, the business resilience system needs to be adaptive in how it supports decision-making. In practice, this means moving away from formulaic management responses based on static risk registers, towards an active decision-making regime based on constantly refreshed KRI data. A key enabler for this is for decision-makers to have up-to-date, tailored dashboards suitable for both operational and leadership levels at their fingertips. Crisis-response and business-recovery plans also need to be adapted regularly, whenever there is a change in the operating model. One of the most commonly reported weaknesses in crisis response – also encountered during corporate responses to the initial COVID-19 outbreak – is that the plans have not been kept updated as the operating model has changed over time.
In terms of organization, one the most important features is to put in place a single, integrated framework that includes risk management, insurance management and crisis recovery. This ensures that there is only one source of truth for data analysis, response plans are updated as risk profiles evolve, and there is a proper balance between risk retention, risk mitigation and risk transfer strategies. Senior executives need to understand that this approach will drive improvement in business performance in the long run.
Operating a dynamic business resilience system of this sort in a large, complex organization is only practical if supported by suitable digital tools. These are needed particularly for:
- Ingesting and constantly analyzing large quantities of data, including hard data from governments, intelligence agencies, etc., as well as soft data from other sources such as Google searches, to provide early indications of emerging trends, risks and weak signals. Indeed, when dealing with catastrophic risks, gathering data from governments and the wider partner ecosystem to which the organization belongs is essential to ensure that risk models are realistic.
- Aggregating external and internal data and providing customized, context-specific analysis and interpretation to support decision-making, including user-driven dashboard graphics that can be tailored for different user personas.
- What-if simulation modeling to assess scenarios and stress-test responses.
- Continuously learning and adapting to improve responses and resilience. AI and ML technologies are especially valuable in this respect, as they enable continuous increases in resilience as the system “learns” and adapts from each iteration.
New predictive data and analytics methodologies also create significant opportunities to drive change in the insurance market:
- Better insight for brokers and underwriters to ensure they can provide more effective services to their policy holders and reduce the overall number of claims.
- More relevant and fit-for-purpose specialty insurance products through reflecting realistic scenarios and associated risk triggers in policy wording.
This also means by implementing data-driven sense-andrespond approaches, companies can reduce insurance costs. Case studies have demonstrated companies have saved up to 15–18 percent on insurance premiums by demonstrating more dynamic approaches to risk management and thereby ensuring that the premiums are more reflective of the actual risks they face.
In the following box we have included two examples of specific use cases of digital tools in risk and resilience management in a large corporate enterprise.
Use case 1 – Proof of concept (POC) for rail service disruption risk management
One of the world’s leading railways implemented a novel approach to improving risk management of service disruptions caused by tree falls. Tree falls, often resulting from adverse weather conditions, are a significant cause of disruption and delay. With support from Arthur D. Little, an ML-based analytical tool was developed to help operations predict where tree falls were most likely to occur. A digital model was created of the railway line and surrounding topography and surface data, including number and proximity of trees, as well as historical data on service interruptions. Machine learning was used to understand past weather patterns and their impact on tree falls and service disruption. By continuously ingesting real-time data on weather conditions, including day forecasts, the tool presented a detailed visual map to indicate dynamic risks where disruption was most likely. This has provided the ability for the company to minimize service disruptions and reduce maintenance costs. The system was delivered in eight weeks and provided a web-based dashboard for the client to use. Further application of the approach more broadly across other risk management domains is under consideration.
Use case 2 – Improving the resilience of clinical-trial planning for a pharma company during COVID-19
A mid-sized pharma company needed to rapidly develop an integrated, global approach for managing the continuity of active and planned clinical trials in light of disruption caused by COVID-19. Using Arthur D. Little’s healthcare and digital experts, a new ML-powered approach was developed to collect and integrate data (internal and external), aggregate and correlate it into focused dashboards, and set up a framework to make or recommend relevant clinical operations decisions, including which locations to prioritize or avoid. The model is constantly updated and refreshed as the work continues, including data on changing government policies and regulations. Further work is continuing to apply the approach more broadly to improve the resilience of future clinical-trial planning.
Insight for the executive
Even when the global economy eventually manages to recover, it will be vulnerable to further shocks. Organizations will need to adopt better strategies and tactics to become what Taleb called “anti-fragile”. Undoubtedly, these will include measures such as reducing supply-chain vulnerability, ensuring adequate backup systems and reducing the dependence of operational continuity on people physically working together. Moving towards an integrated sense-andrespond business resilience system should be a key part of the response. Making this happen requires more than just deploying new digital tools. Organizations should take a true “transformational” approach, for which there are some key priorities:
- Readiness for change: Reinforce the need to embrace, and commit to, new ways of working around risk. This means, for example, recognizing that the past is not a good playbook for the future, adopting agile work methods, and being willing to experiment and learn.
- Data strategy: Regard data as the “new currency” and invest in strong data governance to secure a robust single source of truth, both external and internal.
- Capabilities: Get access to the required capabilities you need to build a dynamic business resilience system, including the best capabilities you can find in data analytics and AI/ML. These may not be in-house.
- Start with a proof of concept: Start with a “stand back” executive-level workshop to take a fresh look at key risk areas and risk drivers, without being constrained by the current corporate risk register. Consider the whole ecosystem, including suppliers, partners, government, regulators, employees and customers. Following this, select a specific, but strategically important, use case on which to conduct an initial proof of concept before moving towards broader implementation.
Unfortunately, our world is one where catastrophes do happen periodically, and we cannot expect that their frequency or severity is necessarily going to diminish in the foreseeable future – on the contrary. We end with another quote from Nassim Taleb: “Prediction, not narration, is the real test of our understanding of the world.”
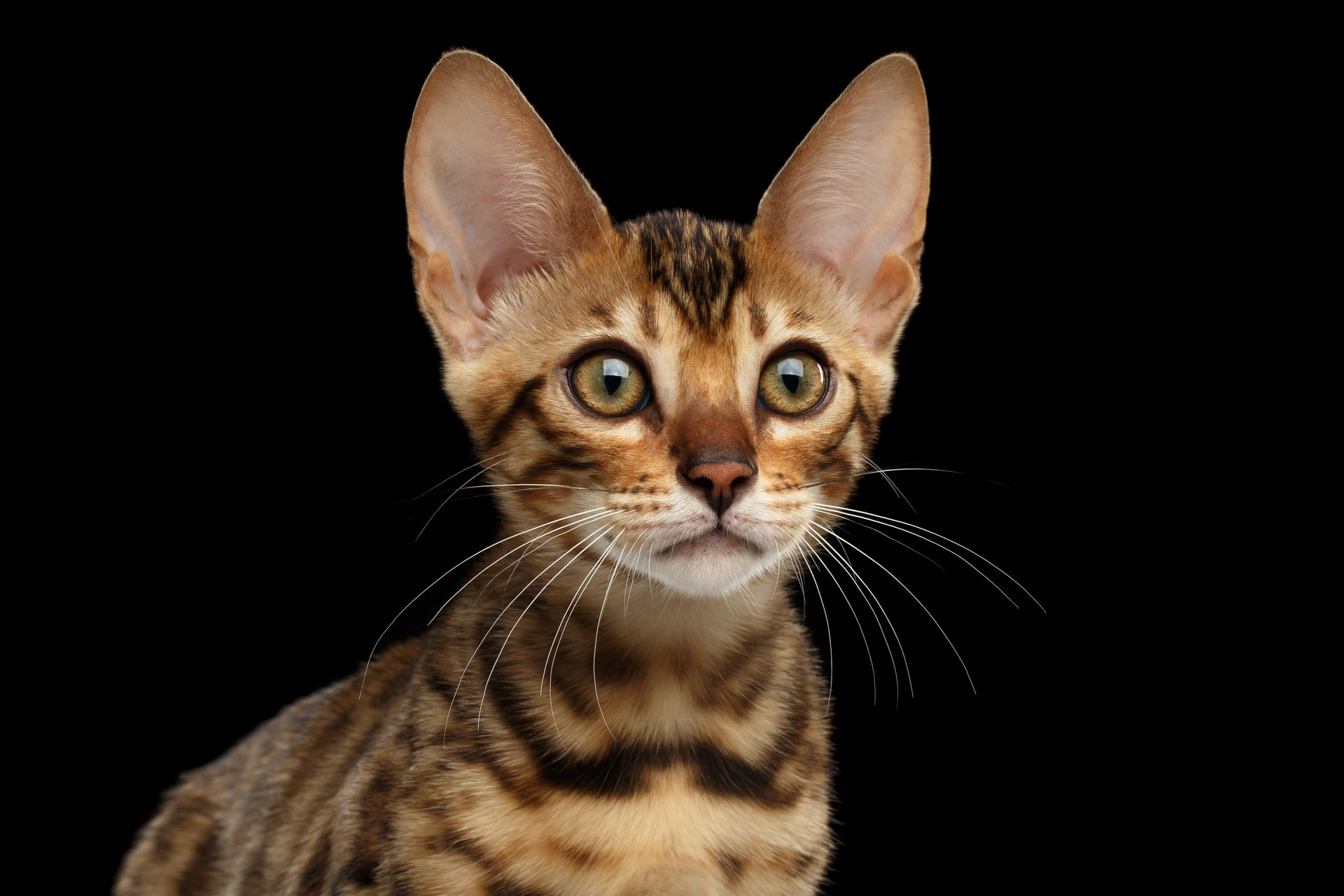
14 min read •
Risk: Strengthening business resilience after COVID-19
Building a “sense and respond” approach using new predictive analytics tools
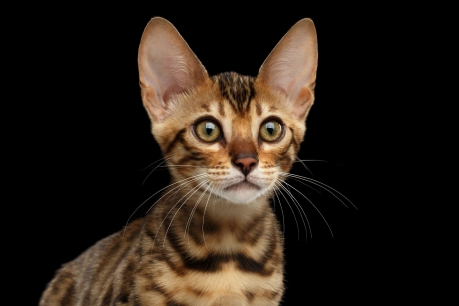
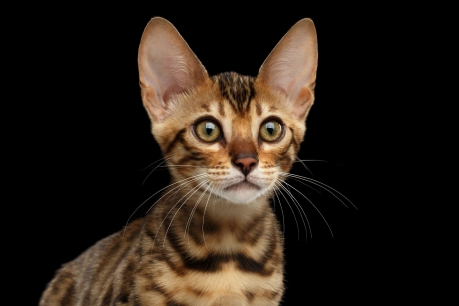
Despite that they had invested in comprehensive crisis management planning, the spread of COVID-19 found many companies ill-prepared, even though it was a known risk with extreme consequences and a reasonable likelihood of occurring. Looking at the evidence, the authors consider the underlying causes of this poor preparedness and set out the key elements of a new business resilience approach suitable for the post- COVID-19 world.
When COVID-19 suddenly escalated from a regional crisis in China to a pandemic, in companies around the world executives rapidly started double-clicking on their crisis management and emergency-response plans. For some, especially those with significant Asian operations, there was already a plan to respond, while for others, the term “pandemic” returned a blank. So began an intensive period of almost continuous back-to-back virtual meetings as leadership teams attempted to regain control of their business operations. Their immediate priorities were securing employee and customer safety and health, followed by maintaining operational continuity, managing cash, helping suppliers, coordinating with governments, engaging with communities, looking towards the recovery phase and, through all of this, continuous intensive communications.
Most companies, as well as governments, quickly realized that they were not well prepared. The breathtaking speed with which the crisis unfolded meant companies had to improvise, because the processes set out in their crisisresponse plans were simply too rigid and slow.
Many found that their plans had not considered the challenges of having to make rapid decisions with incomplete information, and many had underestimated the efforts needed to coordinate across complex external partner ecosystems. Initially, there were huge shortages of basic provisions such as appropriate protective equipment.
Yet, a virus pandemic such as COVID-19 was not at all unexpected. The world had already had a stark warning during the SARS outbreak in the early 2000s, and the risk of similar events was discussed in numerous conferences and panels, including two pandemic tabletop exercises by the John Hopkins Center for Health Security in 2018 and 2019. Bill Gates famously warned about the potential consequences of a virus pandemic in a TED Talk in 2015.
So, if we had a known risk with extreme consequences and a reasonable likelihood of occurring in the medium term, why did governments and companies do so little to invest in the necessary control and response measures? What does this tell us about how companies should go about improving their business resilience? In this article we consider some of the underlying causes of the poor preparedness, and set out the key elements of a new business-resilience approach suitable for the post-COVID-19 world.
Why were we not better prepared?
Major crises with global impact occur regularly. For example, in the last 20 years we have seen, among many others, 9/11, Deepwater Horizon, Fukushima and the 2008/9 financial crash. After every major crisis event there has been an in-depth investigation and analysis. In nearly all cases the conclusion was that there had been weaknesses or errors in how emerging issues had been recognized and dealt with. New controls and/or regulations were then put in place to prevent similar events from happening again – think of changes to air travel after 9/11, changes to banking regulations after the financial crash, and nuclear power policies after Fukushima. Of course, we can expect similar, and probably even greater, changes in the wake of COVID-19. This means next time we have a global crisis that looks like COVID-19, we will be a lot better prepared.
The problem is, of course, that the next global crisis probably won’t look exactly like COVID-19. It could be a different sort of pandemic or an entirely different sort of crisis altogether, such as a cyber-security or environmental crisis, both of which are already well recognized on corporate risk registers.
So why are companies in general still reluctant to invest in controls for catastrophic events that are already recognized but may or may not happen in the medium term? We can identify some common underlying reasons:
- The need to feel it to believe it: It is sometimes said that people do not learn from history. It is perhaps more accurate to say people learn from their own history, but not so well from someone else’s. The countries that were prepared for COVID-19 were the ones that had been through SARS. After a catastrophe, it’s usually not so difficult, with the benefit of hindsight, to find at least one or two prior pieces of evidence or warnings that could have been better heeded. However, in the period before the catastrophe these warnings are often lost in the noise with all the other warnings about potential catastrophes that may or may not happen. So, it is perhaps no wonder leaders do not always take action. Hindsight can be a deceptive tool.
- The “boiling frog” problem: This metaphor (a fable that suggests a frog may fail to jump out of a pan of water as it slowly heats up) refers to the poor ability of humans to take action in the case of threats that build gradually rather than appear suddenly. It is often used in connection with the threat of climate change, but could equally apply to the early phases of COVID-19. The problem here is that most organizations have not done enough to develop clear thresholds for risk tolerance (part of a wider concept sometimes referred to as “risk appetite”). In other words, there are no clear criteria to trigger action in the case of a gradually deteriorating situation that causes a risk to reach a predetermined threshold. Without these clear risk tolerance thresholds, organizations tend, like the frog, to do nothing until it’s too late.
- The pressures of the short term: Governments and business leaders alike tend to be judged over timescales of a few years at most. The average tenure of a CEO has been falling steadily over the last 20 years to no more than five or six years, and governments stand or fall based on their performances between elections. Catastrophic risks tend to be infrequent (high impact, low likelihood), and it is therefore often attractive to park or postpone preparations for them, especially given more pressing short-term priorities and the demands of shareholders or the electorate.
- The difficulties of investment prioritization: In theory, prioritizing investments in risk management is straightforward: for each risk, calculate the expected loss over an agreed period by multiplying its impact by its likelihood of occurring. The value of the “averted loss” through investing in risk-control measures is then compared to the costs of those measures. In practice, however, this is often not enough to prompt boards to invest large sums of money to control major catastrophic risks. Firstly, the calculation usually involves a series of modeled assumptions which are often easy to challenge. Secondly, the sums of money involved in major risk control are often significant, so the intervention may get deprioritized when compared to other risks which may be lower impact but more likely to occur.
- The “can-do” mentality trap: Management cultures typically value leadership traits such as positivity, dynamism, ambition and entrepreneurship. Indeed, all these qualities are important for good leaders. However, in many organizations the corollary of this is that traits such as caution, attention to detail, and concern for what could go wrong are not valued, or even sometimes discouraged, in top leaders. Although consideration of what could go wrong and how to respond should be an integral part of any strategy, in practice these are often perceived as negative or pessimistic topics. Consequently, they are often passed down to risk management functions and treated more as unavoidable red tape and overhead than as value-adding activities for the business.
Moving towards a more resilient business
These causes of poor resilience to major crises are fundamental and rooted in basic human behavior. Although some may berate leaders for their short-sightedness and lack of vision, shouting more loudly and introducing more controls and procedures is unlikely to be the solution.
To make matters worse, the vulnerability of the world to global crises has increased significantly due to increased global connectivity. For example, Nassim Nicholas Taleb, the originator of the Black Swan concept, is quoted in a recent interview with the New Yorker as saying, “The great danger has always been too much connectivity.” The interview goes on to highlight that “proliferating global networks, both physical and virtual, inevitably incorporate more fat-tail risks into a more interdependent and ‘fragile’ system…” The COVID-19 crisis is an all-too-real illustration of the problem. So, in the post-COVID-19 world it is also reasonable to assume that there will be:
- Greater likelihood of local risks escalating globally.
- Higher velocity of escalation of those risks.
- More interconnections between risks – for example, COVID-19 has already led to an increase in cyber-attacks due to the numbers of people working at home.
If we accept that we can’t predict the future just by reviewing the past, and if the fragility of our global systems has increased, the key thing we need from our business resilience system is to sense what is happening in real time, constantly update our predictions, and allow us to take early action before a major risk escalates.
This requires a much more dynamic and adaptive approach than has been traditionally used in conventional static enterprise risk management (ERM) systems. A major shift in philosophy is needed, as shown in Figure 1:
To achieve this shift towards a “sense and respond” philosophy, organizations need to evolve beyond conventional risk-register-based ERMs. Three aspects are key to making this evolution (see Figure 2):
Forward-facing practices: In a sense-and-respond business resilience system the emphasis is changed from rear-facing monitoring and review (such as incidents and losses) towards forward-facing prediction. Using a mix of lagging and leading risk indicators is nothing new, but usually the chosen leading risk indicators (for example, proportion of audits passed successfully or risk training provided) say very little about emerging risks or increasing threats.
The key capability needed for an effective forward-facing approach is the ability to develop realistic and robust causeeffect models. This can be challenging in practice for a complex global operation, especially in view of the high degree of connectedness organizations have within their partner ecosystems, but it is possible and worth spending time on. Once the cause-effect models have been developed, it becomes possible to establish customized and aggregated leading key risk indicators (KRIs). KRIs need to be calibrated to provide a “red flag” prior to a risk event occurring, with this calibration directly related to the organization’s risk tolerance levels.
An example could be a composite KRI relating to supplier defaults in one part of a complex global supply chain, which could provide early warnings of major disruptions further down the line. An effective forward-facing approach also requires an effective horizon-scanning or foresighting capability to identify emerging risks. Often these capabilities are present in companies, but focused on innovation or new product development, and therefore disconnected from corporate risk or business resilience functions. Fortunately, new data-analytics approaches and artificial intelligence (AI) and machine learning (ML) technologies are now becoming available to enable much easier cause-effect analysis, horizon scanning, detection of weak signals, and real-time KRI monitoring.
Dynamic prioritization: Being able to regularly “retune” risk-control priorities to take account of emerging risks is the essence of being dynamic. For this, understanding risk velocity – how quickly an organization will feel the impact of a risk event occurring – is key. Modern data-analytics tools now enable potentially high-velocity emerging risks to be identified more easily and monitored in real time through KRIs.
Adaptive response: Finally, the business resilience system needs to be adaptive in how it supports decision-making. In practice, this means moving away from formulaic management responses based on static risk registers, towards an active decision-making regime based on constantly refreshed KRI data. A key enabler for this is for decision-makers to have up-to-date, tailored dashboards suitable for both operational and leadership levels at their fingertips. Crisis-response and business-recovery plans also need to be adapted regularly, whenever there is a change in the operating model. One of the most commonly reported weaknesses in crisis response – also encountered during corporate responses to the initial COVID-19 outbreak – is that the plans have not been kept updated as the operating model has changed over time.
In terms of organization, one the most important features is to put in place a single, integrated framework that includes risk management, insurance management and crisis recovery. This ensures that there is only one source of truth for data analysis, response plans are updated as risk profiles evolve, and there is a proper balance between risk retention, risk mitigation and risk transfer strategies. Senior executives need to understand that this approach will drive improvement in business performance in the long run.
Operating a dynamic business resilience system of this sort in a large, complex organization is only practical if supported by suitable digital tools. These are needed particularly for:
- Ingesting and constantly analyzing large quantities of data, including hard data from governments, intelligence agencies, etc., as well as soft data from other sources such as Google searches, to provide early indications of emerging trends, risks and weak signals. Indeed, when dealing with catastrophic risks, gathering data from governments and the wider partner ecosystem to which the organization belongs is essential to ensure that risk models are realistic.
- Aggregating external and internal data and providing customized, context-specific analysis and interpretation to support decision-making, including user-driven dashboard graphics that can be tailored for different user personas.
- What-if simulation modeling to assess scenarios and stress-test responses.
- Continuously learning and adapting to improve responses and resilience. AI and ML technologies are especially valuable in this respect, as they enable continuous increases in resilience as the system “learns” and adapts from each iteration.
New predictive data and analytics methodologies also create significant opportunities to drive change in the insurance market:
- Better insight for brokers and underwriters to ensure they can provide more effective services to their policy holders and reduce the overall number of claims.
- More relevant and fit-for-purpose specialty insurance products through reflecting realistic scenarios and associated risk triggers in policy wording.
This also means by implementing data-driven sense-andrespond approaches, companies can reduce insurance costs. Case studies have demonstrated companies have saved up to 15–18 percent on insurance premiums by demonstrating more dynamic approaches to risk management and thereby ensuring that the premiums are more reflective of the actual risks they face.
In the following box we have included two examples of specific use cases of digital tools in risk and resilience management in a large corporate enterprise.
Use case 1 – Proof of concept (POC) for rail service disruption risk management
One of the world’s leading railways implemented a novel approach to improving risk management of service disruptions caused by tree falls. Tree falls, often resulting from adverse weather conditions, are a significant cause of disruption and delay. With support from Arthur D. Little, an ML-based analytical tool was developed to help operations predict where tree falls were most likely to occur. A digital model was created of the railway line and surrounding topography and surface data, including number and proximity of trees, as well as historical data on service interruptions. Machine learning was used to understand past weather patterns and their impact on tree falls and service disruption. By continuously ingesting real-time data on weather conditions, including day forecasts, the tool presented a detailed visual map to indicate dynamic risks where disruption was most likely. This has provided the ability for the company to minimize service disruptions and reduce maintenance costs. The system was delivered in eight weeks and provided a web-based dashboard for the client to use. Further application of the approach more broadly across other risk management domains is under consideration.
Use case 2 – Improving the resilience of clinical-trial planning for a pharma company during COVID-19
A mid-sized pharma company needed to rapidly develop an integrated, global approach for managing the continuity of active and planned clinical trials in light of disruption caused by COVID-19. Using Arthur D. Little’s healthcare and digital experts, a new ML-powered approach was developed to collect and integrate data (internal and external), aggregate and correlate it into focused dashboards, and set up a framework to make or recommend relevant clinical operations decisions, including which locations to prioritize or avoid. The model is constantly updated and refreshed as the work continues, including data on changing government policies and regulations. Further work is continuing to apply the approach more broadly to improve the resilience of future clinical-trial planning.
Insight for the executive
Even when the global economy eventually manages to recover, it will be vulnerable to further shocks. Organizations will need to adopt better strategies and tactics to become what Taleb called “anti-fragile”. Undoubtedly, these will include measures such as reducing supply-chain vulnerability, ensuring adequate backup systems and reducing the dependence of operational continuity on people physically working together. Moving towards an integrated sense-andrespond business resilience system should be a key part of the response. Making this happen requires more than just deploying new digital tools. Organizations should take a true “transformational” approach, for which there are some key priorities:
- Readiness for change: Reinforce the need to embrace, and commit to, new ways of working around risk. This means, for example, recognizing that the past is not a good playbook for the future, adopting agile work methods, and being willing to experiment and learn.
- Data strategy: Regard data as the “new currency” and invest in strong data governance to secure a robust single source of truth, both external and internal.
- Capabilities: Get access to the required capabilities you need to build a dynamic business resilience system, including the best capabilities you can find in data analytics and AI/ML. These may not be in-house.
- Start with a proof of concept: Start with a “stand back” executive-level workshop to take a fresh look at key risk areas and risk drivers, without being constrained by the current corporate risk register. Consider the whole ecosystem, including suppliers, partners, government, regulators, employees and customers. Following this, select a specific, but strategically important, use case on which to conduct an initial proof of concept before moving towards broader implementation.
Unfortunately, our world is one where catastrophes do happen periodically, and we cannot expect that their frequency or severity is necessarily going to diminish in the foreseeable future – on the contrary. We end with another quote from Nassim Taleb: “Prediction, not narration, is the real test of our understanding of the world.”