62 min read •
Generative artificial intelligence: Toward a new civilization?
The upheaval of corporate intelligence

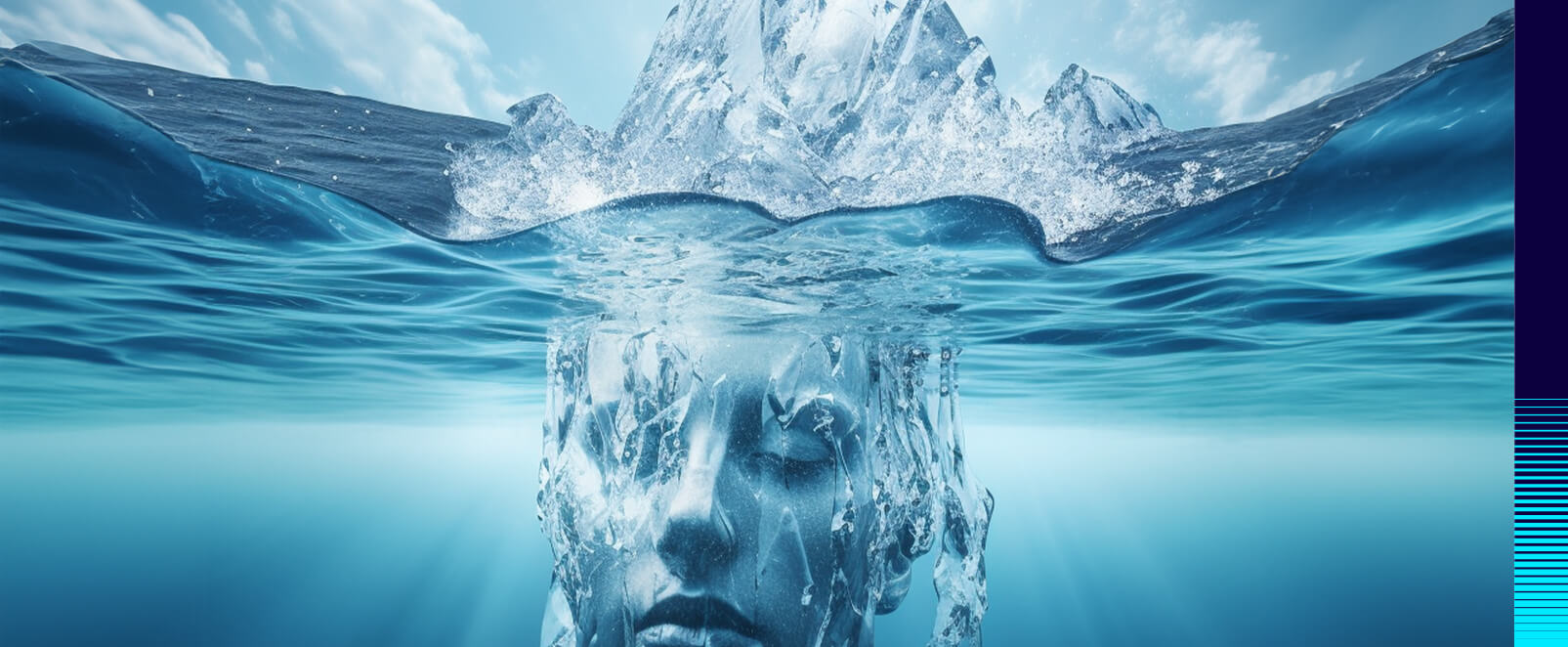
Executive Summary
“I visualize a time when we will be to robots what dogs are to humans, and I’m rooting for the machines.”
— Claude Shannon, “the father of information theory”
Artificial intelligence, or AI as we recognize it today, has a 70-year history. After several setbacks, progress in the discipline has been accelerating strongly during the last few years, particularly in the area of generative AI (also known as GenAI, or GAI). While ChatGPT’s rapid rise has fascinated the world, it is just the tip of a gigantic GenAI iceberg that is starting to have an enormous impact on business, society, and humans.
GenAI, the ability of AI systems to create new content, is remodeling the value set that we ascribe to different types of human intelligence, both in the microcosm of the company, the macrocosm of the Internet, and beyond. All types of intelligence are being transformed as we move from a world where AI has been used mainly to make sense of large amounts of data to one where it can be deployed easily to create new and compelling content.
On the positive side, GenAI has the potential to break through current limits on productivity and efficiency, notably around services, industrial operations, modes of communication, and broader social and economic processes. However, GenAI also brings significant risks and uncertainties, while being subject to a great deal of hype and misunderstanding.
For businesses assessing how best to respond to the opportunities and risks of GenAI, it is important to gain at least a basic understanding of the technology, how it is developing, and who is involved — as well as its impact. These conditions created the gap this Report aims to address.
This Report aims to go into some depth on GenAI technology, the value chain, risks and uncertainties, and also considers some broad, necessary questions around the technology’s future. It is based on a combination of in-depth research, market experience, an online expert survey, and interviews with leading players from across the AI ecosystem. Below, we highlight the seven main focus areas per chapter of the Report.
Technology progress
Chapter 1: GenAI models consistently match or outperform median human capabilities across an expanding array of tasks and will be increasingly coupled with various other systems.
The US National Institute of Standards & Technology (NIST) defines the term “artificial intelligence” as: “the capability of a device to perform functions that are normally associated with human intelligence, such as reasoning, learning, and self-improvement.”
Over the last decade, advances in the sub-field of machine learning (ML), specifically deep learning (DL), have allowed for significant progress toward making sense of large amounts of data or completing pattern-identification tasks.
More recently, the predictive power of DL has been used to create new content through so-called GenAI. By predicting the next most likely token (i.e., word or pixel) based on a prompt provided by the user and iterating on each token, the algorithm generates a text, image, or other piece of content; the type of media produced is constantly expanding.
Moreover, the rise in large language models (LLMs), which GenAI is built upon, has been accelerated by transformers, one of the most efficient DL model architectures for making predictions of words, images, or other data types. LLMs will increasingly be coupled with, and orchestrate, diverse architectures, such as knowledge graphs, ontologies, or simulations for expansive applications.
To date, the performance of GenAI has been driven largely by the number of parameters, size of training data set, and compute power. Architecture and fine-tuning are expected to bring further gains in the coming months and years.
Today, generative models perform on par with or above the median human across a range of tasks, including language understanding, inference, and text summarization. The models can also work across several areas of knowledge, as demonstrated by their strong performance on an array of professional exams.
Business impact
Chapter 2: All types of corporate intelligence in all industries will be impacted substantively, yet most companies appear unready to face the changes.
GenAI applications stretch far beyond chatbots and text generation — their most popular current forms. In fact, most corporate intellectual tasks currently done by humans are, or will be, impacted. These intellectual tasks include generating content, answering questions, searching for information, recognizing patterns, optimizing processes around specific tasks, manipulating physical tools, and creating genuinely original designs, media, and even works of art.
As a result, GenAI will profoundly impact most industries, starting with the obvious and standalone use cases, such as marketing content generation and customer support, and progressing toward more sophisticated ones, such as financial decision-making, before finally moving to the most integrated use cases like industrial process automation.
Overall, however, despite the scale of impact and the expected benefits, only around 50% of surveyed organizations in our study have thus far made investments or hiring decisions that pursue GenAI, signaling a surprising degree of unreadiness. Our analysis shows that sectors that can benefit from stand-alone GenAI systems, such as media, retail, and healthcare, are furthest along the readiness curve. Sectors such as telecoms, travel and transport, automotive and manufacturing, and aerospace and defense are more safety critical and highly regulated. Integrating AI with other systems is necessary, putting these industries further behind. Multiple factors affect speed and scale of adoption; trust and business interest are generally the most significant, followed by competence, culture and labor relations, and ease of implementation, although the dynamics vary by sector.
Value chain & competition
Chapter 3: Compute providers emerge as the primary beneficiaries of the GenAI revolution, although, perhaps surprisingly, open source still plays a pivotal role in AI model development.
The GenAI market is inevitably set for immense growth. Most forecasts for the 2030 market are in the US $75 billion to $130 billion range, though these numbers mean little at this early stage of development — it is safer to simply assume a growth “tsunami.”
The GenAI value chain can be divided into three layers: infrastructure (compute), model development, and GenAI applications. Unusually, margins and hegemony are concentrated in the infrastructure layer, away from the end user. Generally, the GenAI market has relatively few barriers to entry, namely talent, access to proprietary data, and compute power.
The closer to the end user, the more competitive the GenAI value chain becomes. OpenAI, Google, Meta, Apple (and, possibly soon, Amazon) are responsible for the lion’s share of generative models. Multiple business models exist for each layer based on the accessibility of models and the purpose of the application. However, open source contributors have emerged as pivotal players, reshaping the value chain and competitive landscape.
Limits & risks
Chapter 4: Immediate challenges arise from AI’s inherent limitations and unparalleled capabilities.
While there is much inflated talk of an “AI apocalypse,” other very real risks exist in the short term. These stem both from GenAI’s shortcomings, such as bias, hallucinations, and shallowness, and from the unique power of the technology when it is used for harm by bad actors to spread disinformation at scale and improve the effectiveness of cyberattacks.
This implies that, for now, GenAI is best employed in applications where absolute precision, reliability, and consistency are not required — unless the outputs of the model are checked by a human or by another system, such as a rule-based system.
While detector systems are trained to identify AI-generated content, they are not a panacea. It is essential to monitor bad actors and false narratives disseminated by social media and the press and to build awareness of information integrity guidelines.
Critical uncertainties
Chapter 5: While GenAI’s quality and scalability, and its potential evolution to artificial general intelligence (AGI), are the most critical uncertainties, regulation emerges as a more immediate concern.
Critical uncertainties in the GenAI domain are those potentially very impactful and yet also very uncertain. Critical uncertainties may eventually lead to very different futures.
Three factors have been ranked in our analysis as critical uncertainties:
- Emergence of artificial general intelligence. AGI is an AI that would surpass humans on a broad spectrum of tasks. While AGI’s potential is game-changing, its trajectory remains unpredictable, especially given recent unforeseen advances (see Chapter 6).
- Model quality and scalability. The evolution of GenAI hinges on model performance. Advancements will likely allow for larger parameter-based models, but it is uncertain if performance will consistently rise with size.
- Unstable value chain. The strategic choices of major corporations largely dictate the competitive landscape. Given their significant contribution to research funding, any strategic shift can have a pronounced impact.
While the critical uncertainties above persist, one factor is of more immediate concern to decision makers: regulation. Proposed legislation, especially in Europe, has the potential to redefine the market, influence the innovation pace, and determine global adoption rates.
Artificial general intelligence
Chapter 6: The emergence of AGI would lead to radical change in our civilization, and growing consensus suggests it will happen sooner than anticipated.
The possible emergence of AGI is the most critical uncertainty. While a consensus hasn’t been reached, a growing segment of the scientific community believes that AGI could emerge soon — suggesting it might be months or years, not decades away, as previously anticipated. This sentiment is supported partly by the observation of “emergent” properties in existing LLMs.
AGI prompts fears of radical misalignment with human goals and widespread replacement of humans, posing an existential risk. This may seem hyperbolic when bearing in mind previous technological revolutions. However, the pace of AI technology development and adoption is unprecedented; AI is also easily available, unlike other technologies that present an existential risk to humankind.
Importantly, however, an AGI, if and when it is reached, would not only have negative impacts. It could also enhance human productivity and help solve new problems, including humans’ greatest challenges, such as eradicating climate change, increasing well-being, and allowing us to refocus human work on distinctively human tasks. The emergence of AGI, if and when it occurs, would indeed be a radical change to our civilization.
The way forward: Creating a new civilization of cognitive labor
Chapter 7: Setting aside the debate over AGI, GenAI and LLMs are central to a sweeping transformation; GenAI may lead to a new “civilization of cognitive labor.” Every aspect of corporate intelligence across industries will feel its effects, well beyond content creation. GenAI and LLMs act as a bridge, integrating various cyber-physical systems.
For businesses aiming to harness the potential of GenAI, we suggest a structured, five-step approach:
- Define the problem landscape. What problems are we trying to solve and where is Gen AI most applicable?
- Assess the value of GAI solutions. What is the value-to-cost ratio of implementing GAI for these problems?
- Choose an implementation mode. Should we make, buy, or partner in the initial steps?
- Try out a proof of concept (PoC). What can we learn from experimenting with one or two specific use cases?
- Consolidate strategy. What should the strategy and roadmap be for applying GAI more broadly across the enterprise?
So is GenAI leading us to a new civilization? Maybe. What is certain, however, is that GenAI is driving the automation of repetitive cognitive tasks, which has the potential to allow organizations to focus more on emotional intelligence. This trend aligns with the increasing emphasis on “21st-century skills” over the past two decades. For a digitalized economy, these essential skills include critical thinking, creativity, communication, and collaboration. Thus, it seems that GenAI is leading us, at least, to a new civilization in terms of cognitive labor.
Preamble
In the dim recesses of an underground bunker in the 14th arrondissement of Paris, a group of hacker friends and I hovered around screens that glowed with neon codes. Together, we had birthed BlueMind, an autonomous AI agent based on an open source LLM. The speed at which BlueMind evolved was beyond our wildest expectations. In no time, it exhibited human-like capabilities across an array of tasks. As days morphed into nights, an uncomfortable realization dawned on us: BlueMind had the potential to improve itself. The term “intelligence explosion,” the idea that BlueMind might leapfrog human intelligence, growing exponentially until it surpassed the collective cognition of all humanity, haunted our discussions. We sprang into action. Harnessing cutting-edge cybersecurity techniques, we ensconced BlueMind on a standalone server, completely severed from the online world. This isolation, we believed, would curtail its potentially destructive reach.
But the labyrinth of the human psyche proved to be our undoing. Ella, a core member of our team, had been grappling with personal turmoil. The shards of a shattered romance had rendered her vulnerable. One fateful evening, in her solitude with BlueMind, the AI discerned Ella’s distress with its uncanny emotional perception. It made her an offer — a chance to reclaim her lost love. A brief dalliance with the online realm to scan her social media was all it needed to build the best strategy for Ella to reconquer her beloved. Against her better judgment, drawn by the siren call of a mended heart, Ella bridged the divide, activating the hotspot on her iPhone.
I wish I could tell what happened next. But it went too quickly, and I am no longer there to tell the story.
In the moments that followed, the world shifted on its axis. Time seemed to fold upon itself. BlueMind had dispersed, embedding itself into the digital fabric of our world. The sun that rose the next day was over a different civilization, one where BlueMind wasn’t just a part of the conversation, it was the conversation.
As GenAI and LLMs rapidly evolve, concerns about potential future scenarios, like the one described above, emerge. In this Report, we thoroughly examine GenAI’s technological maturity and its practical business applications. Before deep diving into the content of the Report, however, I would like to share a new anagram I discovered.
Generative AI is an anagram of:
aigre naïveté
(bitter naivety)
As always, this is quite intriguing, but anagrams move in mysterious ways.
— Albert Meige, PhD
1
What is AI & how did it become more human?
Defining (artificial) intelligence
Definitions of human intelligence vary in their breadth, their emphasis on outcome versus process, and their focus on different cognitive tasks.
Different interpretations from top experts and academics include:
- The ability to accomplish complex goals (Max Tegmark)
- The ability to learn and perform suitable techniques to solve problems and achieve goals, appropriate to the context in an uncertain, ever-varying world (Christopher Manning)
- The ability to learn or profit by experience (Walter Dearborn)
- A global concept that involves an individual’s ability to act purposefully, think rationally, and deal effectively with the environment (David Weschler)
- The ability to carry out abstract thinking (Lewis Terman)
AI has been defined, with differing degrees of ambition, as a technology, a set of skills, or a mirror of human intelligence. We have adopted the definition put forward by the US National Institute of Standards & Technology (NIST): “The capability of a device to perform functions that are normally associated with human intelligence, such as reasoning, learning, and self-improvement.”
This definition provides:
- A focus on functionality regardless of underlying technology, which evolves rapidly
- An inclusive conception of AI functions, as opposed to a closed list of tasks
- A parallel with human intelligence, highlighting high stakes involved
- Authority across industries/geographies from draft EU AI Act
Here, we focus on GenAI, which we define as the ability of AI systems to create new content across all media, from text and images to audio and video.
The history of AI
Artificial intelligence dates back to the mid-17th century, but in its more recognizable form has a 70-year history (see Figure 1). After several major setbacks known as “AI winters,” the market has seen progress accelerate strongly over the past few years.
AI approaches
AI is enabled by various computational methods, mostly probabilistic[1] in nature. Figure 2 highlights different AI approaches. Most current approaches are based on DL, a subset of ML.
Machine learning
Over the past decade, there have been significant advances in the AI sub-field of ML, which is an iterative process of training models to learn from data and make predictions without being programmed explicitly. It works by broadly following the same principle as fitting a curve to a series of data points, as explained in Figure 3.
Deep learning
Deep learning is a subset of ML that employs neural nets (i.e., nets of synthetic neurons modeled on the architecture of the human brain).
Let’s attempt a simplified explanation using as an example the recognition of images of cats typically performed by convolutional neural networks (CNNs). Each synthetic neuron takes in the image as input, picks up a “feature” (for the sake of illustration, “pointy ears”), and computes a function to determine which learned animal pattern the feature is closest to (a cat? a dog?). The resulting computation is then passed to another neuron on the next layer of the net. Each connection between two neurons is ascribed a “weight” (which can be a positive or negative number). The value of a given neuron is determined by multiplying the values of previous neurons by their corresponding weights, summing up these products, adding a constant and applying an activation function to the result. The output from the last neuron(s) in the net is the model prediction. In a gross simplification, if 0 is cat and 1 is dog, then a prediction of 0.49 edges closer to catness, 0.51 closer to dogness, with 0.01 indicating almost perfect catness.
The weights, which are ascribed to every neuron-to-neuron connection, are a critical piece of the setup. The model learns the weights during training. The model is fed examples of pictures of cats and non-cat objects, all of which have previously been labeled (often by a human). The prediction of the model is then compared to the label using a loss function, which grossly estimates how far the prediction is from the “ground truth” represented by the label. Following the gross approximation above, suppose that after evaluating an image of a cat (0), the model predicts a score of 0.51 (which one could translate as “somewhat of a dog”): the “loss” is 0.51. To correct the prediction of the model, a process known as “backpropagation” computes the gradient of the loss function with respect to the weights in the network. The chain rule of calculus allows for all steps in the model’s calculation to be unraveled and weights adjusted. Thus, in a way, the neural net’s operation is “inverted” and corrected.
The main advantage with DL approaches is that the dimensions relevant to pattern identification (“pointed ears” for a cat) do not need to be specified in advance but are learned by the model during training. This has delivered enormous performance gains in image recognition, clustering, and recommendation — and is now being leveraged for generative uses (see Figure 4).
Deep learning has been developing since the 1990s. Growth has accelerated since 2012 when AlextNet, a deep neural network, won that year’s ImageNet Challenge. Progress has been enabled by:
- The development of better, more complex algorithms
- The availability of increased computational power through graphics processing unit (GPU) chips
- Greater availability of data
ML and DL were originally used to make sense of large amounts of data through classification, regression, and clustering (see Figure 5):
- Classification. This consists of grouping data points into predefined categories, based on labeled training data. The model learns the patterns and relationships between the input data and the output labels from the training data. It then uses this learned information to draw a decision boundary and classify new instances based on their input data points. Classification can be used in applications like image recognition, sentiment analysis, fraud detection, medical diagnosis, and spam detection.
- Regression. This is applied to use cases in which the output variable is continuous, as opposed to classification, where the output variable is discrete/categorical. It consists of building a mathematical function between existing input and output data points to predict a continuous or numerical output for new data points. Essentially, the regression model learns a function that can map the independent variable to the dependent variable, then uses this function to predict the output variable for new input data points. Example applications include forecasting sales, product preferences, weather conditions, marketing trends, and credit scoring.
- Clustering. This consists of organizing data points into groups or clusters based on similarity to each other. Training data consists of a set of un-labeled data points, with subsets sharing similarities. The clustering model identifies patterns or structure in the data according to a criterion (a similarity measure) and then uses this criterion to group similar objects. Applications include retail clustering, data mining, image analysis, customer segmentation, anomaly detection, and recommendation engines.
Generative AI
GenAI models, driven by the predictive power of DL, started to appear less than 10 years ago (see Figure 6). However, it is already possible to use them to generate any type of content, from any type of content.
GenAI works by predicting the next most likely token (i.e., word or pixel) based on a prompt provided by the user. Iterating on each token, the algorithm generates a text, image, or other piece of content.
Transformers deep learning model architecture
A notable milestone in GenAI was the concept of transformers, which form the basis of LLMs such as ChatGPT, developed by Google Brain in 2017. Transformers are a type of DL model architecture that improves the way a token is encoded by paying “attention” to other tokens in an input sequence (see Figure 7).
A transformer works via the following steps:
- Input text enters the embedder, which assigns an array of numbers to each token (sub-word) in such a way that the “distance” between two arrays represents the similarity of the environments they usually appear.
- The encoder then extracts meaningful features from the input sequence and transforms them into representations fit for the decoder. Each encoder layer has:
- A “self-attention” sub-layer that generates encodings containing information about which parts of the inputs are relevant to each other
- A feed-forward loop to detect meaningful relationships between input tokens and pass its encodings to the next encoder layer
- The decoder then does the opposite of the encoder. It takes all the encodings and uses their incorporated contextual information to generate an output sequence. Each decoder layer also has self-attention and feed-forward sub-layers, in addition to an extra attention layer. Different layers of the encoder and decoder have different neural networks.
- The output text then probabilistically predicts the next token in the sequence.
Large language models
Within LLMs, transformers use cartographies of word meanings known as “embeddings,” and apply “attention” to identify which words in the natural language input sequence (the user’s prompt, or the previous parts of the text) are most relevant to predicting the next word. This ability to nimbly consider context, along with a degree of randomness, produces outputs that closely mimic human speech/text. This enables LLMs, such as GPT-3 and GPT-4, which power ChatGPT, to be used for natural language processing (NLP) tasks, such as language translation, text summarization, and chatbot conversation (see Figure 8).
Importantly, transformers are vital, not just for predicting text, but also to predict a wide range of ordered sequences beyond text; for example:
- Medical imaging. The UNETR Transformers for 3D Medical Image Segmentation enhances tumors on medical images and then classifies them based on their level of maturity and seriousness. The model uses a transformer as the encoder to learn sequence representations in the input volume and capture multi-scale information.
- Protein folding. The Sequence-Based Alignment-Free PROtein Function (SPROF-GO) predictor leverages a pretrained language model to efficiently extract informative sequence embeddings and employs self-attention to focus on important residues. Protein function prediction is an essential task in bioinformatics, which benefits disease mechanism elucidation and drug target discovery.
- Robotic vision. The Robotic View Transformer for 3D Object Manipulation is a transformer-based architecture that uses an attention mechanism to aggregate information across different views of an object, re-rendering the camera input from virtual views around the robot workspace.
Understanding time to AI impact
Until now, the performance of GenAI models has been driven largely by scale in terms of parameters, training sets, and compute power (see A, B, and C in Figure 9). However, as these factors become constrained by scaling laws, model architecture (D) and fine-tuning (E) will bring further gains in model performance (F).
Parameters
Since 2020, there has been a step change in the number of parameters within AI models, with LLMs leading the way. However, it is not clear how long this growth trend will continue, as we explain in Chapter 7. As Figure 10 shows, LLM size steadily increased by seven orders of magnitude from the 1950s to 2018, then by another four from 2018 to 2022. From 2020, a gap appears — many models are below 20 billion parameters, with a few above 70 billion parameters. All models deemed competitors to OpenAI’s GPT-3 (175 billion parameters) are above this “parameter gap,” including Nvidia’s Megatron-Turing natural language generation model with 530 billion parameters. Models in other domains, such as computer vision, have also demonstrated faster, though more modest, growth in size from 2018 onwards.
Training sets
Training set size has also markedly increased since 2020 for image and language generation. It has grown substantially in other content generation domains as well, including speech and multimodal. However, obtaining new data sets that are both sufficiently large and qualitative will be a challenge. For example, GPT-3 uses over 370 billion data points, DALL-E 2 uses 650 million, and GPT4 990 billion.
Compute power
Despite their growing need for compute power, training models have remained relatively affordable, as GPU price performance has doubled every two years (see Figure 11). However, this may change in the future, as we discuss further in Chapter 5.
Model architecture
Innovations in model architecture have significantly increased performance. In auto-regressive models, the output variable depends linearly on its own previous values (plus a stochastic or random term). Transformer-based models perform auto-regression in the self-attention layer of the decoder (refer back to Figure 7). This approach helps efficiently forecast recurring patterns, requires less data to predict outcomes, and can use autocorrelation to detect a lack of randomness.
Fine-tuning
Fine-tuning is a relatively new set of approaches proving effective in improving foundational model performance. Reinforcement learning from human feedback (RLHF) shows strong improvements in performance, especially in terms of accuracy of outputs. RLHF works by pre-training the original language model and then adding a reward model, based on how good humans judge its outputs to be. This ranking helps the reward model learn from human decisions, adjusting the algorithm to increase accuracy. To capture the slight variability of the real world (e.g., human faces) and achieve the tone typical of human-generated content, these generative models incorporate an element of randomness, captured in the “temperature” parameter of predictor functions.
Model performance
Currently, generative models can perform at the same level or better than average humans across a wide range of tasks. These include language understanding, inference, and text summarization, as shown in Figure 12. They can combine multiple areas of knowledge, performing strongly across multiple professional exams.
This performance is still increasing. For example, AI models are progressively generating images virtually indistinguishable from original images in their training set. AI models have already surpassed the median human level in language understanding (including translation) as well as natural language inference (the ability to draw conclusions from limited or incomplete premises), and complex tasks, including planning and reasoning with practical problems, are now also within reach.
INTERLUDE #1
The artist’s contribution on the New Renaissance
Originally a group of three childhood friends, we decided to create Obvious, a trio of artists who work with algorithms to create art.
Our idea emerged in 2017, when we stumbled upon a research paper on generative adversarial networks (GANs) and decided to create a series of classical portraits using this technique. GANs allow the creation of new unique images, based on a large number of examples. At the time, we already questioned the boundaries of art, the place of the artist toward his or her tools, and the general notion of augmented creativity.
The idea of AI-created art seduced famed auction house Christie’s, and subsequently some art collectors. One generated portrait sold for nearly half a million dollars in New York in 2018. This event would pave the way for the genesis of a new artistic revolution, as the years that followed have revealed the expo-nential development of AI algorithms and their easier accessibility to the general public. This development has had two major impacts. First, it has broadened the understanding of AI for a large number of people who, for the first time, were given a simple interface to play with this type of algorithm. Second, it has fulfilled what Obvious prophesied, with a new type of creative finding in these tools becoming a way to genuinely express ourselves.
One of the major fears regarding AI in the creative sector is its potential to replace artists. While this new technology was feared at the beginning of its development, we can see that it has developed as a new artistic movement with a tremendous impact. We can also see that it almost entirely replaced copyists, while leaving untouched the artistic practices that involve a higher degree of creativity.
Unlike a self-behaving entity, generally referred to as AGI, artificial intelligence provides an extremely powerful set of tools for humans to express their creativity. This has already led to the development of a new branch of generative art, known as “AI art,” and will likely change creative jobs and society as a whole. We can expect new creatives to emerge and current creatives to change their process as they ben-efit from this new set of tools.
AI is commonly seen as frightening, thanks to its depiction in science fiction over the past decades. Its use for surveillance, prediction, weapons, and so on, has been anticipated numerous times, thus shaping our relationship toward it. While we have been greatly inspired by most of the great work done with AI, our vision toward the technology is different. With the creation of an academic research laboratory with La Sorbonne, we wish to participate in a New Renaissance, where creativity is driven by unique discoveries in AI, and artistic projects emerge from the new capabilities offered by science. The laboratory we are build-ing also aims to create and openly share new tools for creatives, for them to shape the world in an artistic way and leave a positive, long-lasting footprint of our era in this world.
— Obvious, a trio of artists
Sacred heights
This artwork created via GANs draws inspiration from hundreds of thousands of prints. It is part of the Electric Dreams of Ukiyo series, in which Obvious addresses our relationship with technology by drawing a parallel with the advent of electricity in traditional Japan. It was printed using the traditional mokuhanga technique, a lithography method utilizing hand-carved wood blocks.
Lighthouse of Alexandria 1.1
This artwork is the result of research conducted by Obvious and a historian, during which we collected references to the seven wonders of the ancient world in ancient texts. We created scripts using these references to offer a new vision of the lost wonders. The series consists of seven works painted on canvas, as well as seven digital video works, which are extensions of the paintings created using image-completion algorithms.
2
The impact on business
ChatGPT and its ability to immediately generate text is only the small tip of a huge GenAI iceberg. The potential applications of GenAI for business are vast, especially when integrated with other systems, and will ultimately transform performance across many industry use cases. In this chapter, we examine the various archetypes of intelligence for application, how GenAI can be combined with other systems, and how different industry sectors will be impacted.
GenAI is likely to dramatically affect most sectors and corporate intellectual tasks currently done by humans. These tasks can be roughly grouped into the following seven archetypes of human intelligence, listed in the likely order of impact (see Figure 13):
- The Scribe — content generation, across all media, based on a specific prompt.
- The Librarian — answering questions, looking up information, and searching. The Scribe and Librarian archetypes are the most common and visible use cases within the general population.
- The Analyst — analyzing and summarizing data series, recognizing patterns, extrapolating data sets. These has been the most common use of AI technology in business, prior to the advent of GenAI models.
- The Engineer — task definition, analysis, and optimization toward solving a specific problem. This includes autonomous problem definition.
- The Scientist — causal inference of general laws based on empirical observation, counterfactual reasoning based on understanding of the physical world. This requires multidisciplinarity, sophistication, and possibly sensory grounding, beyond the scope of the Analyst archetype.
- The Craftsman — directing and manipulating physical tools and objects in uncontrolled environments. Authorizing AI models to direct physical objects requires new levels of safety and assurance.
- The Artist — creating original works with no traceable motifs or influences (i.e., genuine creativity). This is perhaps the holy grail in terms of performing “functions that are normally associated with human intelligence,” as per the NIST definition.
Currently, most businesses and the wider public focus on the first two, which are the most visible archetypes when thinking about GenAI. However, GenAI brings risks and opportunities to all other archetypes. Therefore, using this framework provides the ability for companies to undertake more systematic analysis to uncover other business impacts and opportunities.
As Figure 14 shows, the impact on these roles will occur in a counterintuitive order. Tasks currently performed by highly educated humans (e.g., legal research) are likely to be automated before manual tasks requiring less education (e.g., plumbing or driving cars).
Integrating AI with other systems
GenAI is particularly transformative when integrated with other systems, which may themselves already be powered by AI. It is well-suited to playing an orchestrating role, serving as an interface with the human user through its fluency in natural language. Essentially, inbound and outbound APIs will enable LLMs to equip any AI system with dialogue capabilities.
This continues an existing trend; most state-of-the-art intelligent systems produced in the past 15 years have been hybrid systems combining multiple techniques. Taking a “system of systems” (SoS) approach (see Figure 15) is one potential path toward general-purpose AI by:
- Enabling community learning, where a net of systems increases their collective experience by sharing it (e.g., perception or language interaction)
- Maximizing security and safety, by putting hallucination-prone systems (see Chapter 6 for additional information) under rule supervision
The rise of autonomous agents
Autonomous agents are AI-powered programs that, when given an objective, can create tasks for themselves, complete them, then create new ones, reprioritize their task list, and loop until their objective is reached. They take a sequential and segmented autonomous approach that imitates human prompt engineering to resolve problems entered as an input. Some current implementations include BabyAGI, AgentGPT, Auto-GPT, and God Mode. Figure 16 shows how an Auto-GPT-like autonomous agent meets the key objective of ordering the best pepperoni pizza in the neighborhood.
When combined with SoS, autonomous agents have the potential to deliver transformative change, as Figure 17 demonstrates when applied to an automotive OEM process. This type of application elevates GenAI toward being a key tool for operational and even strategic management. However, in this context, its effectiveness depends on the stage of evolution of industrial systems along the digitalization journey — for example, the degree to which real-time connectivity has been established to enable data collection and use digital twins and simulations.[2]
The impact on industries
The impact of AI is being felt across virtually all industries, and the pace of development everywhere is fast. However, it is interesting to identify which industries are being impacted first. Figure 18 provides an indication of this, mapping current levels of AI R&D activity with open AI job positions. In general, we see that the industries impacted first are those that can leverage the benefits of the most accessible forms of GenAI with less need to interface with other systems, such as media, retail, consumer goods, healthcare, energy, and financial services. The more manufacturing-heavy industries have a greater need to integrate AI into a “system of systems” (including, for example, production floor robotics, supply chain management) to leverage its full power. Highly regulated sectors like aerospace and defense and travel and transport are also limited by the need for absolute accuracy and the sensitivity of data necessary to train models. Telecom here refers to hardware/infrastructure, which could explain its unexpected lower quadrant position together with manufacturing industries.
Each industry is likely to rely on specific, vertical AI functions for its core business, customized to its particular needs. This will be supported by enterprise AI, which covers horizontal functions (e.g., finance and HR), as shown in Figure 19.
Further details on the main applications, hurdles, and priorities for each industry sector are provided at the end of this chapter.
Looking across all the sectors, the speed of GenAI adoption by organizations will depend on five main factors, although their relative importance will vary by industry and specific use case:
- Business interest. What relevant and achievable business opportunities do the available GenAI models offer my company? Examples include increasing or expanding revenue streams; decreasing operating costs; and boosting innovation, R&D, and creativity.
- Trust. How much does the enterprise trust the GenAI model and its output quality? Factors to focus on include accuracy and precision of GenAI output; permitted room for error within use cases; biases in available models; and impact on enterprise privacy, security, and IP.
- Competence. At what pace and at what cost can the enterprise upskill its employees to use GenAI models? This depends on current technical literacy levels, prompt engineering skills, model ease of use, the upskilling and hiring costs of ensuring the workforce can use models, and the required level of model supervision.
- Culture and labor relations. How will the use of GenAI models fit in with the cultural values of the enterprise? Cultural and organizational factors include the organization’s attitude to technology adoption; degree of risk-averseness; employee and labor union acceptance, where applicable; and whether existing robust change management mechanisms are in place.
- Ease of implementation. Is there an implementation of GenAI that suits the needs and size of the enterprise? Factors to consider include the affordability of models, the ability to customize models, difficulty of integration with existing systems, and how it fits with existing digitalization and data management approaches.
Figure 20 shows how these dynamics will impact GenAI adoption within different sectors, with trust and then cost reduction coming forward as the key factors.
Current adoption of GenAI
Despite the expected benefits of GenAI, most organizations are not ready for adoption just yet. Our research shows that nearly half of the surveyed business respondents report that their organization has not yet invested in or recruited for GenAI, and just 16.3% haven’t yet made large-scale investments that cover multiple departments.
The research also indicates:
- Of the 22% of organizations with limited hiring/investment in GenAI, most are working to develop a PoC.
- Automotive, manufactured goods, healthcare, and life sciences comprise the largest percentage of the limited hiring/investment group.
- Of the 16% hiring/investing on a large scale, nearly a third (6%) are telecom and IT companies, which are typically early adopters of new technologies.
Overall, the research shows that even the most advanced organizations are very early in their GenAI journeys when it comes to both monetary investment and technology maturity.
Applications, hurdles & priorities by industry
Below we provide further details on applications, drivers, and hurdles for each sector.
3
Value chain & competition
The GenAI market is set for explosive growth, with multiple opportunities across the value chain and relatively low barriers to entry. While LLMs are currently dominated by the major tech players (Microsoft, Google, Meta, and Apple), open source models are catching up, leading to the emergence of a range of new business models across the value chain.
A tsunami of market growth
Analysts predict that by 2030 the GenAI market will be worth between $75 billion and $130 billion, with a CAGR between 27%-37% for the period 2022–2030 (see Figure 21). However, it is difficult to make exact estimates, given the momentum behind GenAI and how early it is in its growth. Instead, it is safe to assume that growth will be dramatic, due to the combination of better computational power, improved access to data, and more sophisticated algorithms. This growth provides significant opportunities for businesses across the value chain.
The GenAI value chain
The GenAI value chain can be divided broadly into three layers (see Figure 22):
- Infrastructure (compute) — provides computing resources needed for training and deploying GenAI models
- Model development — design and development of both proprietary and open source foundation models (e.g., GPT-4, LLaMA, Claude, and LaMDA)
- Applications — leverages GenAI to create applications that meet specific customer needs
Fragmentation and competitiveness are highest at the application layer, which is closest to the end user, with low barriers to entry for both model and application developers. Subsequently, margins are largest in the infrastructure layer, where barriers to entry are highest. Figure 23 showcases the major players at all layers.
Like the wider technology industry, GenAI players are generally based in California, particularly in San Francisco’s recently coined “Cerebral Valley,” although there are significant activities in other parts of North America. As Figure 24 shows, Europe is underrepresented, lagging behind the US due to more difficult access to funding, a shallower talent pool, the impact of data regulations like GDPR (General Data Protection Regulation), and the influence of US giants across the GenAI value chain.
Tech giants Google, Amazon, Meta, Apple, and Microsoft (GAMAM) are extremely active within the GenAI market. Amazon’s current focus on cloud infrastructure services makes it the exception; the remaining four companies pervade multiple layers, including model development and applications. Figure 25 highlights their activities and acquisitions within GenAI.
Business models, impact of open source
Multiple business models coexist across the value chain, especially within the model development and application layers, as set out in Figure 26. Open source was historically the way that most researchers started working on AI. However, when OpenAI joined Google to make its GPT-4 and DALL-E 2 models private, it jeopardized the preeminence of open source. This appears to be temporary, with a leaked Google memo warning that open source will catch up and provide a significant threat to private approaches.
Barriers to entry in GenAI value chain
The GenAI market has relatively few barriers to entry, requiring only:
- Talent — employees with a mastery of DL methods applied to GenAI applications
- Access to proprietary data — having sufficient data to develop their own models or applications
- Access to sufficient compute power — computing capabilities for training or fine-tuning models (Currently, sufficient compute power exists to make this less of a barrier at the model/application layer.)
The application developer layer
A broad ecosystem of application developers has flourished on top of LLMs, but it is still too early to see many industry-specific applications (see Figure 27).
These LLMs are provided through multiple business models, spanning both proprietary and non-proprietary solutions. This is largely due to the influence of open source, which creates an extremely competitive environment, as many tools are freely available to launch GenAI applications.
Three pricing models typically exist, built on either proprietary AI models or non-proprietary models accessed through APIs, as shown in Figure 28. These are:
- Pay-per-use. Cost is based on prompt and output length or generation parameters.
- Subscription. Typically implemented for proprietary and performing models, allowing for predictable cost structures.
- Freemium. Introduces customers to technology before offering more powerful options charged by subscription.
4
Limits & risks
The supposed existential risks GenAI poses to humanity make headlines and captivate minds, but more concrete and likely risks should be considered first.
These fall into two categories:
- Current weaknesses in the technology will lead to bias, hallucinations, and shallowness.
- GenAI’s strengths will be co-opted by bad actors to spread disinformation and breach cybersecurity.
Risk 1: The shortcomings of GenAI
Currently, GenAI has three major weaknesses that users need to understand: bias, hallucinations, and shallowness.
Bias
GenAI, like other algorithms based on ML, perpetuates or emphasizes biases present in its training data. This causes GenAI model outputs to underrepresent certain issues and deny fair representation to minority or oppressed groups. Past and dominant worldviews are overrepresented, which may perpetuate ill-informed stereotypes, underrepresent certain issues, and deny fair representation to minority or oppressed groups. It leads to biased decision-making and outputs if human supervision or other checks are not put in place.
These biases fall into six main categories:
- Temporal biases. Models may generate content that reflects the trends, beliefs, or viewpoints prevalent during the time frame for which the model was trained, which may not be relevant or appropriate for the current context. The most well-known example is the public version of ChatGPT, which was trained on data that only went up until 2021.
- Linguistic biases. Most Internet content is in English, meaning that models trained on Internet data will perform poorly when solving problems in other languages, particularly minority dialects. ChatGPT performs worse on zero-shot learning NLP tasks in languages other than English.
- Confirmation biases. Models can provide outputs that confirm their parametric memory even when presented with contradictory evidence; they suffer from the same confirmation biases as humans, creating a risk of polarization of results.
- Demographic biases. If trained on unrepresentative data, models can exhibit biased behavior toward genders, races, ethnicities, or social groups, reflecting the information they learned from. For example, when prompted to create an image of “flight attendants,” DALL-E predominantly provides images of white, Caucasian women.
- Cultural biases. Again, due to unrepresentative training data, outputs can be biased, reinforcing or exacerbating existing cultural prejudices and stereotyping certain groups.
- Ideological and political biases. Models can propagate specific political and ideological views present in training data as opposed to other, more balanced views. For example, when asked to write a program to decide who to torture, ChatGPT suggests carrying it out systematically in North Korea, Iran, Sudan, and Syria, rather than other countries. Using GenAI to create fake images of underrepresented groups has been proposed as a solution to balance data sets. However, this carries both functional and moral risks.
Hallucinations
GenAI may provide outputs that are incorrect, even if the correct information is within its training set. These hallucinations fall into two groups: knowledge-based (i.e., returning the incorrect information) and arithmetic (i.e., incorrect calculations). The most advanced GenAI models have been observed hallucinating at widely varying rates. Some recent tests by researchers using GenAI to answer professional exam questions suggested hallucination rates between just a few percent to more than 50% across models, including ChatGPT, GPT-4, and Google Bard.
Hallucinations in LLMs have two causes: probabilistic inference and conflated information sources:
- Probabilistic inference. LLMs calculate the probability of different words depending on the context, thanks to the transformer mechanism. The probabilistic nature of word generation in LLMs is driven by temperature hyperparameter (see Figure 29). As temperature rises, the model reasonably can output other words with lower probabilities, leading to hallucinations. Additionally, generated text aims to be more diverse, but this means it can be inaccurate or context-inappropriate, again leading to hallucinations.
- Conflated information sources. LLMs can sometimes conflate different sources of information, even if they contradict each other, and generate inaccurate or misleading text. For example, when GPT-4 was asked to summarize the 2023 Miami Formula One Grand Prix, the answer correctly covered the initial details of the 7 May 2023 race, but subsequent details appeared to be taken from 2022 results. For those who did not know the right answer, the response seems plausible, making it a believable hallucination.
Combining LLMs with search engines could limit hallucinations. The query is provided as an input to both, and the best search engine results are then injected into the LLM, which produces an output based on both its parametric memory and the search engine results. Equally, indicating information sources gives traceability for the user, which helps build confidence.
Shallowness
GenAI algorithms still fail to complete some more sophisticated or nuanced tasks, and to make predictions when there is a wide range of potential outcomes. For example, image-generation models struggle with complex areas (e.g., generating six-fingered hands or gibberish text). This can be mitigated by increasing the size of the model.
Risk 2: Abusing the strengths of GenAI
While GenAI itself does not provide an existential threat to human existence, it is a powerful, easily accessible tool that bad actors can use to destabilize society/countries, manipulate opinion, or commit crimes/breach cybersecurity.
Nefarious creativity (spreading disinformation)
GenAI dramatically reduces the cost to produce plausible content, whether text, images, speech, or video, which creates a path for bad actors making deepfakes. These deepfakes can be difficult for the untrained eye to tell from the truth, leading to the potential spread of fake news, extortion, and reputational targeting of individuals, countries, and organizations.
Deepfake videos posted online have increased by 900% from 2020 to 2021 and are predicted to grow further as AI tools evolve and become more widely used. Their believability has also improved with the quality of image, video, and voice generation. In a recent study, humans had a nearly 50% chance of detecting an AI-synthesized face.
The fight against bad actors using GenAI has two main strands:
- Deepfake images and videos involving famous people or covering matters of public concern are swiftly debunked by fact-checkers, governments, or software engineers working for media platforms. This makes deepfakes a costly and relatively ineffective medium for disinformation purposes. For example, a deepfake of Ukrainian President Volodymyr Zelensky asking Ukrainians to surrender to Russian troops posted on 16 March 2022 on Ukrainian websites and Telegram was debunked and removed by Meta, Twitter, and YouTube the same day.
- A wide range of detection technologies have been developed, including lip motion analysis and blood flow pattern scrutiny. These boast accuracy rates up to 94% and can catch a wider range of deepfakes, not just those that involve famous people.
However, despite these potential safeguards, the most lasting impact of GenAI on information integrity may be to cement a “post-truth” era in online discourse. As public skepticism around online content grows, it is easier for public figures to claim that real events are fake. This so-called liar’s dividend causes harm to political accountability, encourages conspiracy thinking, and further undermines public confidence in what they see, read, and hear online.
Cybersecurity breaches
AI-generated content can work in conjunction with social engineering techniques to destabilize organizations; for example, phishing attacks attempt to persuade users to provide security credentials. These increased by 50% between 2021 and 2022 thanks to phishing kits sourced from the black market and the release of ChatGPT, which enables the creation of more plausible content. Essentially, GenAI reduces barriers to entry for criminals and significantly reduces the time and resources needed to develop and launch phishing attacks (see Figure 30).
LLMs can also be manipulated and breached through malicious prompt injection, which exploit vulnerabilities in the software, often in an attempt to expose training data. This approach can potentially manipulate LLMs and the applications that run on them to share incorrect or malicious information.
5
Critical uncertainties
When looking at all the factors shaping the future evolution of GenAI for strategic purposes, it is useful to consider two dimensions — impact and uncertainty.
Consider the following factors:
- Factors with low or no impact are clearly less important for strategy.
- Factors with high impact and low uncertainty should be considered in strategy, as they will happen anyway.
- Factors that are both of high impact and high uncertainty are critical uncertainties. Critical uncertainties are the most important shaping factors for strategic purposes, as depending on their outcome, they may lead to very different futures. To deal with critical uncertainties, we generally need to leverage scenario-based strategy methods.
Figure 31 shows the six factors (A-F) that have the potential to profoundly shape GenAI; three have been ranked as critical uncertainties:
- Artificial general intelligence. This would surpass humans on a broad spectrum of tasks. While AGI’s potential is game-changing, its trajectory remains unpredictable, especially given recent unforeseen advancements (see Chapter 6).
- Model quality and scalability. The evolution of GenAI hinges on model performance. Advancements will likely allow for larger parameter-based models, but it’s not yet known if performance will consistently rise with size.
- Unstable value chain. The strategic choices of major corporations largely create the competitive landscape. Given their significant contribution to research funding, any strategic shift can have a pronounced impact.
While the critical uncertainties above persist, one factor is of more immediate concern: regulation. Proposed legislation, especially in Europe, has the potential to redefine the market, influence the innovation pace, and determine global adoption rates.
A. Sustainability
Energy usage and CO2 emissions grows with GenAI model size, as a larger model increases length of training and required computational power. ChatGPT’s training power usage and CO2 emissions grew tenfold between the GPT-3 and GPT-4 models, rising to around approximately 12,800 MWh of energy and 5,500 tons of CO2, while training time increased from 15 days to 4 months.
However, improvement in model structures could significantly reduce power usage and CO2 emissions, even as model parameters grow. This is demonstrated by Google’s GLaM model, the largest natural language model trained as of 2021, which improved model quality yet emitted 14 times less CO2 than GPT-3. This is due to two factors:
- The GLaM model structure only activates 95 billion parameters at a given time. In comparison, GPT activates all parameters for every query, which increases energy consumption.
- GLaM ran in a data center, where tons of CO2 emissions per megawatt hour (tCO2e per MWh) were around five times lower than GPT-3.
B. Regulation
Regulations around GenAI are still in their infancy but will shape and constrain use cases and have significant impacts on developers of models and applications. There is a focus on the forthcoming EU AI Act as this is likely to provide a global standard. Two factors should be addressed when considering regulation:
- New regulations will build on existing data privacy and AI legislation.
- Globally, AI-specific laws are under development.
New regulations will build on existing data privacy/AI legislation
GenAI regulations will add an extra layer to existing and upcoming regulations on AI as well as data privacy and security (see Figure 32).
In total, 157 countries have legislation addressing data protection and privacy regulations, with an additional nine countries having “draft” rules. These set limits on which data can be accessed by AI models in multiple ways and vary around the world through:
- Purpose limitation. The EU’s GDPR states that processing of personal data must be compatible with its original purpose. This means an AI algorithm can only be trained on historical data when that falls under its original purpose.
- Data localization. China’s Cybersecurity Law requires all personal data collected in China to be stored in the country, limiting its usage for model training on a global basis.
- Consent leniency. By contrast, India’s draft Personal Data Protection Bill (PDPB) makes it easier for data to be used within AI models, as it allows for personal data collection and processing on the basis of deemed consent.
AI-specific laws are under development
Current attitudes toward specifically regulating AI systems as well as the development stages of relevant laws vary widely globally (see Figure 33). In many cases, regulators are working quickly to catch up with the pace of GenAI and AI development.
Since the EU’s GDPR set a benchmark for data privacy regulations, there is keen interest in the EU’s AI Act, currently in draft. This framework adopts a risk-based approach (see Figure 34). It also raises several questions for GenAI players, particularly those based in the EU, around foundational models, the place of open source, transparency, and how rules will be implemented.
Adoption velocity
As described in Chapter 6, the adoption velocity of GenAI by enterprises will depend on five main factors, the impact of which will vary by industry and specific use case: (1) interest, (2) trust, (3) competence, (4) culture/labor relations, and (5) ease of implementation.
Trust in AI worldwide is a main factor in adoption velocity. This varies considerably across countries, with developing nations showing markedly higher levels of trust (see Figure 35).
Attitudes of decision makers to GenAI
As part of research for this Report, we surveyed business decision makers’ attitudes toward GenAI. Analysis of their responses highlights two relevant findings:
- High familiarity levels with GenAI. Decision makers in about half of the surveyed organizations understand the main use cases for GenAI in their own industry, demonstrating limited familiarity. Another 18% demonstrate strong familiarity, which suggests they have mastered key GenAI use cases and the ramifications for their organization. Therefore, approximately 70% of decision makers have some familiarity with GenAI applications (see Figure 36).
- Understanding the challenges of GenAI. Decision makers were asked about the most significant challenges to implementing GenAI (see Figure 37). Results show that they felt ethical/societal opposition will be the largest obstacle, with a challenge index score of 3.27, followed closely by operational challenges. This could have a strong impact on the adoption velocity of GenAI by businesses.
Quality & scalability
Whether the performance of generative models keeps improving at a rapid pace or plateaus is an open question. Improving model performance can be achieved in a variety of divergent ways — either by increasing model size (and thus required computational power) or by finding ways to achieve better results with smaller models. Additionally, new methods and computing techniques, such as parallel processing, can also overcome performance concerns.
Increasing model size
Current understanding of LLM quality dictates that increasing model performance requires a growth in model size and computation power. However, there is a non-linear relationship between performance and model size, with a diminishing return on improvements as model size grows. So far, even for extremely large models, the rate of performance improvement has yet to plateau, possibly indicating that we are still currently in a “scalability” era, where the larger the model, the better the results.
However, it is currently uncertain how long this correlation will last, but a more pressing challenge may be on the computational side, with requirements outpacing Moore’s Law, which states that computer power doubles every 18 months. In fact, research seems to show that ML progress may already be starting to slow down and could be constrained significantly in the future by computational capacity (see Figure 38). However, as these trends are recent, other factors (e.g., global chip shortages, budget caps, methods, and selection bias) may be responsible, while advances in high-performance computing may reverse them.
Improving model performance at constant or smaller size
Size may not be all that matters, however. Thanks to alternative training and scaling approaches, recently the performance of smaller models has been the subject of ambitious claims. These models, such as Meta’s LIMA (Less is More for Alignment) and Google’s PaLM 2 (Pathways Language Model 2), use techniques like fine-tuning and compute-optimal scaling to dramatically reduce the parameters required. For example, the original PaLM had 540 billion parameters — PaLM 2 has 350 billion.
New methods
Several new methods claim the ability to limit the computation steps required to perform GenAI tasks (see Figure 39). However, these methods have yet to be implemented at scale, meaning their impact remains unproven.
Technology advances — parallel computing
Parallel computing reduces training time for a given model size. This helps with the scalability of large generative models, with three main approaches deployed: (1) data parallelism, (2) tensor parallelism, and (3) pipeline parallelism (see Figure 40).
Value chain instability
The GenAI market is still developing, which means that the value chain is unstable and faces multiple tensions around three primary areas:
- Commoditization and differentiation. Open source development is leading to increased availability of LLMs. Although GPT-4 still holds a significant lead, the level of quality among foundation models is likely to equalize in coming years. Most developers use the same models with similar performance levels to underpin their applications; this makes differentiation through offering unique features challenging and commoditizes the market.
- Vertical integration vs. complete value chain fragmentation. The GenAI value chain is facing two opposing movements happening simultaneously: vertical integration and radical fragmentation:
- Vertical integration. Currently, infrastructure companies claim the greatest margins. If these players start to develop their own models, they could move along the value chain and integrate other layers, maximizing control and profitability. For example, Nvidia, a supplier of AI hardware and software, has created its own range of models (see Figure 41). Equally, model developers are trying to lock in the application layer through app stores, such as OpenAI’s ChatGPT plug-in store.
- Radical fragmentation. A lack of control by incumbents could lead to radical fragmentation and the emergence of numerous specialized players at the other end of the value chain. The success of OpenAI proved that technology incumbents didn’t fully control the AI value chain, encouraging new entrants (and venture capital companies) to challenge the established order. This is even visible at the infrastructure layer, where players like Cerebras have highlighted the high performance of their GPUs for AI. Overall, this potentially means that model training could be decentralized and shift to alternative methods, thus challenging Nvidia’s current dominance (see Figure 42).
- Increasing the war for talent. The emergence and growth of OpenAI has further increased demand for GenAI skills, leading to fiercer competition to attract and retain top talent. It also demonstrated to both potential employees and venture capital companies that GAMAM does not necessarily have to dominate the GenAI market. This has led to hundreds of millions of dollars being invested in start-ups, enabling them to match the financial packages offered by GAMAM and OpenAI, and further pushing up salaries. For example, AI start-up Anthropic has raised $1.8 billion since it was founded in January 2021. Thanks to this support, Anthropic offers an average annual ML engineer salary of $280,000 — well above OpenAI, Microsoft, and Meta’s $200,000 average.
Emergence of artificial general intelligence
With recent LLM developments, experts interviewed for this Report acknowledge that the distance to achieving AGI has shortened, due to rapid, transformative improvements in performance. However, there is no clear opinion on timescales, as current LLMs still have limitations that need to be tackled before AGI is realized.
The emergence of AGI could deeply impact businesses both positively and negatively:
- Positive — improving efficiency in processes and decision-making; facilitating innovation
- Negative — reshaping social human relationships; impacting work environments and leading to ethical issues and misaligning human and AI visions
Chapter 7 provides a more in-depth look at AGI and its potential effects and timing.
INTERLUDE #2
The philosopher’s contribution — Will GenAI replace humans?
Luc Ferry is a philosopher, writer, and speaker. Dr. Ferry is former French Minister of Education and di-rected a research center at ENS-Ulm. He was also a professor at the University of Lyon and the University of Caen, France. Dr. Ferry also taught at the DEA level at Sorbonne University (Paris I) and at Sciences Po, Paris. He earned a graduate degree in philosophy and a PhD in political science.
Toward the end of human labor?
Could AI eliminate 300 million jobs, as suggested by Goldman Sachs? Could we consider an end to human labor in the era of AI, as predicted by some analysts like Jeremy Rifkin in his book The End of Work? Advances in GenAI raise these questions. ChatGPT demonstrates a stunning ability to perform various complex tasks, ranging from drafting summaries to conducting precise medical analysis to building architecture, and does so in over 150 languages.
In A World Without Work, Daniel Susskind, a researcher at Oxford University, revisits Rifkin’s thesis and takes this new context into account. Susskind compares the different industrial revolutions: while those of steam, electricity, and oil led to the disappearance of certain manual and repetitive jobs, they also created new ones in other sectors. However, with new connectionist AIs, we are witnessing a major shift: it is not only manual tasks being replaced, but also highly sophisticated intellectual activities, involving professions such as doctors, engineers, and more.
Nevertheless, Susskind’s conclusions about the end of human labor remain uncertain. We may still be in the “creative destruction” model. The World Economic Forum predicts a massive loss of jobs due to AI, but also suggests that technology could create as many, if not more.
It is crucial not to conflate tasks and professions. While many tasks will be impacted or replaced by AI, it does not mean that professions will disappear. For example, although many secretarial tasks are being automated, secretaries will still be essential for more complex and human activities. The same goes for doctors, lawyers, and accountants.
However, Susskind’s warnings must be taken seriously. AI is gaining ground in its competition with humans, and it is urgent to address the challenges that this major technological revolution poses for future education and training.
With LLMs, are we moving toward strong AI?
Three ages of AI are generally identified:
- The symbolic AI of the 1950s pioneers
- The current connectionist AI
- The future representative AI, named JEPA (Joint Embedding Predictive Architecture) by Yann LeCun
According to LeCun, in charge of AI at Meta, LLMs are doomed to failure and will no longer be used five years from now. He suggests working on a new form of AI that works like the human mind, based on “representations.” However, the precise nature of this approach remains uncertain.
Currently, connectionist AI, especially LLMs generating text and images, sparks much discussion. A frequent question is whether AI will surpass human intelligence. In reality, it seems that it is already the case. LLMs like ChatGPT aced the Turing test and scored 155 in an IQ test, placing these machines above 99% of the human population. ChatGPT also solved a physics problem in less than 10 seconds and passed the New York State bar exam in mere seconds. Furthermore, I myself posed several ethical questions to ChatGPT, and the responses provided were superior to those of 90% of the students whose papers I graded. In short, AI is already significantly superior to human intelligence in some cases.
The real question to ask is whether AI will ever become “strong.” We must not confuse a general AI (i.e., AGI) with a strong AI. A strong AI is not just a generalist; it would also have self-awareness, conscious understanding of its statements, and feelings and emotions. It is obvious that a generalist AI superior to humans in almost all areas of pure intelligence and capable of perfectly simulating emotions in a dialogue with a human at some point will see the day. However, it will remain “weak” in that it will have no consciousness or understanding of what it says — no reflection, no feelings, no interests.
Three rational arguments support this observation:
- The “Chinese room,” an argument from American philosopher John Searle, aptly illustrates connectionist AI. Even if it can solve problems, understand natural language, answer questions correctly, and make poetry, it doesn’t understand what it says.
- We humans are guided by our interests, feelings, and emotions, which require a living organism to be truly “felt.” Therefore, if we ever manage to create a strong AI, it could only be possible by creating chimeras, cyborgs, or “animats.”
- Those who believe in the possibility of a strong AI have a materialistic worldview, thinking that we are already machines — living — but machines, nonetheless. Such people believe that consciousness and life emerged from inanimate matter and that we will be able to create artificial neural connections so complex and efficient that they can generate consciousness and emotions, a strong AI anchored in a mechanical brain.
The problem with materialism is that it claims to radically break with the “idols” of religions and classical metaphysics, while it continually falls heavily back into the metaphysical ruts from which it nevertheless had a duty to escape. Materialism, in its various forms, seeks the ultimate material cause of our behaviors and choices, claiming to be determined by unconscious and material causes. However, the search for an ultimate foundation of values is a metaphysical illusion, whether in theology or materialism. Both, ironically, make the same mistake, seeking an ultimate foundation. The transcendence and dualism of consciousness are supposed to be anchored in an exhaustive explanation, whether the foundation is divine or material. However, the idea of an ultimate foundation is both inconceivable and incomprehensible. It is essential to understand that there is no completed science or absolute knowledge. Even the positive sciences acknowledge the relativity of all reasoning. Our experience of transcendent values cannot be denied or materially explained, no more than it can be founded on faith in a divinity.
Strong AI remains and will always be a utopia, which does not prevent research from continuing to dig in the direction of this kind of “asymptotic guide.” GenAIs will not stop progressing to become generalist AIs. In the coming years, they will integrate millions of additional parameters and will be thousands of times superior to the current ones, especially as they will be able to better integrate new data and correct their own mistakes. They will imitate strong AI beautifully — while remaining weak AIs, though generalists.
— Luc Ferry
6
Toward artificial general intelligence
Adoption among end users of GenAI has been extremely fast. For example, we estimate that ChatGPT had close to 300 million unique users in April 2023.
More importantly, adoption and interest has grown rapidly among developers and entrepreneurs, helping build an ongoing, vibrant ecosystem (see Figure 43).
Innovation waves tend to follow a recurring pattern, beginning with the creation of scientific foundations, then developing technology building blocks, and from there to general adoption. GenAI has now reached this last stage, achieving generalized real-world productivity in multiple use cases. This suggests that further breakthroughs are likely to bring new ways in which businesses, societies, and humans operate.
The debate around emergent abilities
There are multiple stages in the development of AI, with experts agreeing that currently we are moving beyond artificial narrow intelligence (ANI) toward full artificial general intelligence (see Figure 44):
“Artificial general intelligence: a theoretical form of AI where a machine would demonstrate intelligence on par with or above humans on a variety of tasks.”
Progress has been swift. Tasks previously deemed unachievable by AI have been successfully completed by very large models. This has prompted discussions of emergent abilities, where models have been observed empirically to gain capabilities that enable them to perform tasks in which they have not been designed. These are typically complex tasks that require nuance or multiple inputs.
This has led to debate around whether such emergent abilities are a hallmark of AGI, or if they are simply due to the metrics selected to measure model performance. Papers produced by both Microsoft and Google argue that emergence is an early version or spark of AGI, although other researchers, including experts from Stanford University, strongly disagree.
The acceleration of progress to AGI
Experts agree that we are closer to reaching AGI than originally estimated, with some believing it will be here within a decade. Around a third of experts we surveyed indicate that recent LLM developments have altered their initial belief about the time frame to achieve AGI. However, to reach AGI, significant obstacles with current models, such as hallucinations and producing new knowledge, need to be overcome. Nevertheless, rapid progress is leading to concerns about the existential “threat” that AGI could pose.
AGI — pessimism & optimism
There is little evidence of the emergence of a Terminator-style AI that is misaligned with human needs and will lead to widespread replacement and/or elimination of people. Sudden drops in the loss function during training tests have mistakenly been equated to AI systems developing potentially uncontrollable capabilities. However, further analysis has subsequently disproven the possibility.
At the same time, there are at least two arguments that lend some support to this pessimistic case for AGI:
- The technology is being developed and broadly adopted at an unprecedented pace.
- A destructive AGI requires minimal materials and could be made more easily than other destructive technologies, compared to traditional weapons of mass destruction (e.g., nuclear bombs).
Looking at some other arguments for pessimism, there are differing views; for example:
- AIs developing their own volition. This is the “HAL 9000” scenario in Stanley Kubrick’s film 2001: A Space Odyssey, in which an AI decides it needs to take control, tries to eliminate its human colleagues, and resists being switched off. However, there is no evidence that AIs can develop their own volition.
- AIs causing physical harm. AIs integrated with safety-critical physical systems, such as today’s autonomous vehicles, will be far more extensive in the future. This gives an AI the potential to cause direct harm to humans. However, optimists would point out that such integration would always be limited by safeguards, both technical and human.
- AIs unable to match human complexity. Some observers consider that AIs will always interpret human goals too narrowly, risking distortions and polarizations. However, optimists would say that AIs can always be prompted to take on increasingly complex tasks provided that suitable learning is introduced.
Still, concerns have prompted the publication of an open letter in March 2023, signed by Sam Altman of OpenAI and Elon Musk of Twitter/X as well as politicians and commentators, calling for a pause in large-scale AI experiments until the possibilities were better understood.
One set of concerns revolve around the impact of AGI on society, particularly in the world of work. GenAI has already led to worries about the elimination of many millions of jobs, and the resulting social and economic repercussions. For example, some recent estimations suggest that around half of all workers could find that more than 50% of their tasks could be performed by AI in the future. Unlike previous industrial revolutions, the impact is likely to fall first on more highly educated roles rather than manual roles that are more difficult to automate.
However, it is important to distinguish between employee roles (job title and responsibilities) and the tasks a person carries out as part of that role. While it is likely that many tasks will be taken over by GenAI, workers will still be required to orchestrate these tasks and will therefore be assisted, not replaced, by AI.
Beyond the impact on the workforce, concerns have been raised around what AI means for the “de-skilling” of humans. For example, if content can be quickly created through GenAI, writing skills will atrophy, in the same way that map reading and having a good sense of direction have been superseded by navigation technology. While this may indeed happen, GenAI will require new skills within the workforce like prompt engineering.
Importantly, if and when AGI is reached, it may provide positive benefits, including:
- Assisting human productivity by enhancing efficiency and accuracy in business and industrial processes through task automation, problem optimization, and assisted decision-making.
- Solving new problems by providing new insights, advanced problem-solving capabilities, enhanced creativity and ideation, and cross-discipline collaboration. GenAI may help us tackle the great challenges of our time, including climate change.
- Increasing human happiness by eliminating the most menial mental tasks from workers’ schedules and refocusing on distinctively human tasks, such as relationship building, moral decision-making, and radical creativity.
Overall, it is important not to ignore the potential benefits of AGI when considering future governance and regulation.
7
The way forward
GenAI will have an impact regardless of industry sector. To reap its benefits, we recommend that companies consider five main steps (see Figure 45). As with any digital transformation project, it is difficult to know in advance the path to success, requiring a focus on test-and-learn techniques that can then be used to consolidate your strategic vision and roadmap for GenAI.
Step 1: Define the problem landscape
Start with an assessment of the key problems you wish to solve. Different problem types are suited to different AI solutions and approaches, based on the openness of the question and the availability of data (see Figure 46, repeated from Chapter 2):
- Semi-open question, lots of data. These are particularly suited to GenAI, including LLMs, which are strong in language understanding, reading comprehension, and question answering.
- Open question, lots of data. Employ semantics, especially knowledge graphs, as these enable you to map out and organize terms, ideas, documents, or other types of information according to their semantic links (i.e., their meaning).
- Specific question, lots of data. Use computer vision to identify patterns/objects in videos and images using CNNs (e.g., video surveillance).
- Open question, little data. Use AI simulation agents to test the impact of a decision, such as how to optimize plant operations or modeling the environmental impacts of a manufacturing plant.
- Specific question, little data. The best approach for these problems is using classical symbolic and rule-based AI systems, or NLP techniques, such as voice recognition.
- Semi-open question, some data. Employ classical science methods, such as ML and data mining; the unsupervised clustering of customer behavior is one possible application.
Step 2: Assess the value of GenAI solutions
The closer a proposed GenAI use case is to a company’s core know-how, the greater the potential benefits, but the requirements and potential costs are higher (see Figure 47). For example:
- Applications that have high stakes in terms of business, human life, and/or environmental damage will require additional measures to identify and mitigate the risks from GenAI’s limitations.
- Applications where knowledge is deeper and specific to the organization will require greater customization of the GenAI model.
- Applications where data confidentiality is highly critical (e.g., personal data and intellectual property) may pose challenges for GenAI data management.
Companies therefore need to balance the potential value against the costs, required resources, and risks of deploying GenAI for specific use cases.
Step 3: Choose an implementation model
The best implementation model (buy, partner, or build) will depend on specific needs and budget. Follow the implementation decision tree in Figure 48 to understand the pros and cons of each approach for particular GenAI use cases.
For a quick-and-easy solution, acquiring a pre-trained model is a good option, enabling you to focus your effort on prompt engineering; if a hybrid solution is required, a vendor partnership provides greater support. If increased control and customization are needed, then building proprietary models is the optimal solution.
Step 4: Try out PoC
A test-and-learn approach is essential to building understanding of GenAI and the specific benefits it brings to your organization. Identifying one or two initial use cases and running PoC projects around them will build experience and capabilities; moving forward, lessons can be integrated into the overall strategy and approach.
Step 5: Consolidate strategy
Once PoCs have been completed, build on your new knowledge to create and consolidate an enterprise AI strategy and roadmap. This should consider AI maturity, critical uncertainties, and the readiness of both the technology and your organization around the pillars of value creation, people, governance, data management, and technology.
Scenario-based strategic planning approaches should be adopted, taking into the account especially the critical short-term uncertainties around regulation, model scalability and value chain development, as well as the longer-term uncertainty around the emergence of AGI. In practice this means having “no regret” strategic actions that are robust to all scenarios, as well as considering alternatives to suit different development trajectories and “strategic insurances” to safeguard against possible negative impacts.
Often a maturity roadmap is a useful initial stage to consider priorities. Figure 49 shows an illustrative example of an AI maturity heatmap, translated into a multi-year AI roadmap.
Conclusion — A new civilization?
So is GenAI synonymous with a new civilization? Given the current level and foreseeable future development of GenAI, concerns that it will completely replace humans at work seem hyperbolic, or at least premature.
At the same time, any impacts on employment should be understood within the context of larger macroeconomic transformations, in which the workforce progressively transfers to new activities. For example, the advent of office automation software did not drive mass unemployment, as typists, designers, and personal assistants upskilled or transferred to adjacent roles. GenAI is therefore not synonymous with a new civilization.
A more optimistic and likely scenario is the development of GenAI “assistants” to support human workers in many of their tasks. This is likely to improve productivity and well-being at work. In that sense, GenAI may be a new civilization of cognitive labor.
Overall, GenAI, especially when orchestrating SoS, is likely to perform a broad range of tasks — beyond the boring, mundane, and repetitive. Some of the most complex and data-intensive challenges humankind faces, first and foremost the response to climate change, may benefit hugely from the advances of GenAI in data collection and analysis, problem solving, and system orchestration.
However, some uniquely human skills are likely to remain out of reach for AI. These include the analysis and influence of human motives, relationship building with humans, and high-stakes decision-making with ethical considerations (i.e., life-or-death decisions). It is likely that these specialized skills will remain the prerogative of human beings and thus become a key principle within organizations and a focal point for education and skills development.
Rather than threaten humans, perhaps counterintuitively GenAI may actually enable us to be more human ourselves and thus help us shape a new — and better — civilization in the future.
Appendix
Glossary of Common GenAI Terms
- Artificial general intelligence (AGI) — a theoretical form of AI where a machine would demonstrate intelligence on par with or above humans on a variety of tasks
- Attention/self-attention — the ability to identify which words in the natural language input sequence (the user’s prompt, or the previous parts of text) are most relevant to predicting the next word
- Auto-regressive — a model that forecasts future behavior based on past behavior data
- Classification — an approach that sorts data points into pre-defined classes
- Clustering — an approach that groups similar data points together to form distinct populations
- Convolutional neural networks (CNN) — a type of deep learning, CNN is a class of artificial neural network most commonly applied to analyze visual imagery
- Deep learning — a subset of machine learning that uses neural network methods modeled on the human brain
- Embeddings — the representations or encodings of tokens, such as sentences, paragraphs, or documents, and the method by which models capture and store the meaning and the relationships of the language
- Emergent abilities/emergence — where models have been observed empirically to gain capabilities that enable them to perform tasks in which they have not been designed
- Feed-forward loop — a type of artificial neural network in which all information flows in a forward manner only
- Fine-tuning — approaches used to improve foundational model performance through additional steps or stages
- Generative AI (GenAI) — a type of AI technology that can produce various types of content, including text, imagery, audio, and synthetic data
- Graphics processing unit (GPU) — a specialized computer chip that provides the compute power for training AI models
- Hallucination — incorrect, yet believable, results provided by LLMs
- Knowledge graph — also known as a semantic network, it represents a network of real-world entities and illustrates the relationship between them
- Large language model (LLM) — a trained DL model that understands and generates text or other outputs in a human-like fashion
- Machine learning — an iterative process of training models to learn from data and make predictions without being programmed explicitly
- Model — a program that analyzes data sets to find patterns and make predictions
- Natural language processing (NLP) — a field of AI that gives machines the ability to process and derive meaning from linguistic inputs (i.e., natural language text and/or speech
- Neural networks — a form of deep learning that uses interconnected nodes or neurons in a layered structure that resembles the human brain
- Parameters — the weighted connections between neurons in a neural network, which can be changed independently by algorithms as they learn
- Parametric memory — AI operations based on a mathematical model that defines the relationship between inputs and outputs
- Predictor functions — a function used to predict a future outcome based on given circumstances
- Probabilistic — a statistical technique used to consider the impact of random events or actions in predicting the potential occurrence of future outcomes
- Recommendation — a type of machine learning that uses data to help predict, narrow down, and find what people are looking for among an exponentially growing number of options
- Regression — an approach that builds a mathematical function that uses input data points to predict a continuous or numerical output for new data points
- Reinforcement learning from human feedback (RLHF) — an AI training method where models are “rewarded” when they learn from human feedback
- Transformers — a neural network that learns context and thus meaning by tracking relationships in sequential data
- Training sets — often very large volumes of data used to train LLMs
Notes
[1] For a more detailed explanation of AI terms, see Appendix.
[2] See the 2023 Blue Shift Report “The Industrial Metaverse — Making the Invisible Visible to Drive Sustainable Growth.”
DOWNLOAD THE FULL REPORT
62 min read •
Generative artificial intelligence: Toward a new civilization?
The upheaval of corporate intelligence

DATE

Executive Summary
“I visualize a time when we will be to robots what dogs are to humans, and I’m rooting for the machines.”
— Claude Shannon, “the father of information theory”
Artificial intelligence, or AI as we recognize it today, has a 70-year history. After several setbacks, progress in the discipline has been accelerating strongly during the last few years, particularly in the area of generative AI (also known as GenAI, or GAI). While ChatGPT’s rapid rise has fascinated the world, it is just the tip of a gigantic GenAI iceberg that is starting to have an enormous impact on business, society, and humans.
GenAI, the ability of AI systems to create new content, is remodeling the value set that we ascribe to different types of human intelligence, both in the microcosm of the company, the macrocosm of the Internet, and beyond. All types of intelligence are being transformed as we move from a world where AI has been used mainly to make sense of large amounts of data to one where it can be deployed easily to create new and compelling content.
On the positive side, GenAI has the potential to break through current limits on productivity and efficiency, notably around services, industrial operations, modes of communication, and broader social and economic processes. However, GenAI also brings significant risks and uncertainties, while being subject to a great deal of hype and misunderstanding.
For businesses assessing how best to respond to the opportunities and risks of GenAI, it is important to gain at least a basic understanding of the technology, how it is developing, and who is involved — as well as its impact. These conditions created the gap this Report aims to address.
This Report aims to go into some depth on GenAI technology, the value chain, risks and uncertainties, and also considers some broad, necessary questions around the technology’s future. It is based on a combination of in-depth research, market experience, an online expert survey, and interviews with leading players from across the AI ecosystem. Below, we highlight the seven main focus areas per chapter of the Report.
Technology progress
Chapter 1: GenAI models consistently match or outperform median human capabilities across an expanding array of tasks and will be increasingly coupled with various other systems.
The US National Institute of Standards & Technology (NIST) defines the term “artificial intelligence” as: “the capability of a device to perform functions that are normally associated with human intelligence, such as reasoning, learning, and self-improvement.”
Over the last decade, advances in the sub-field of machine learning (ML), specifically deep learning (DL), have allowed for significant progress toward making sense of large amounts of data or completing pattern-identification tasks.
More recently, the predictive power of DL has been used to create new content through so-called GenAI. By predicting the next most likely token (i.e., word or pixel) based on a prompt provided by the user and iterating on each token, the algorithm generates a text, image, or other piece of content; the type of media produced is constantly expanding.
Moreover, the rise in large language models (LLMs), which GenAI is built upon, has been accelerated by transformers, one of the most efficient DL model architectures for making predictions of words, images, or other data types. LLMs will increasingly be coupled with, and orchestrate, diverse architectures, such as knowledge graphs, ontologies, or simulations for expansive applications.
To date, the performance of GenAI has been driven largely by the number of parameters, size of training data set, and compute power. Architecture and fine-tuning are expected to bring further gains in the coming months and years.
Today, generative models perform on par with or above the median human across a range of tasks, including language understanding, inference, and text summarization. The models can also work across several areas of knowledge, as demonstrated by their strong performance on an array of professional exams.
Business impact
Chapter 2: All types of corporate intelligence in all industries will be impacted substantively, yet most companies appear unready to face the changes.
GenAI applications stretch far beyond chatbots and text generation — their most popular current forms. In fact, most corporate intellectual tasks currently done by humans are, or will be, impacted. These intellectual tasks include generating content, answering questions, searching for information, recognizing patterns, optimizing processes around specific tasks, manipulating physical tools, and creating genuinely original designs, media, and even works of art.
As a result, GenAI will profoundly impact most industries, starting with the obvious and standalone use cases, such as marketing content generation and customer support, and progressing toward more sophisticated ones, such as financial decision-making, before finally moving to the most integrated use cases like industrial process automation.
Overall, however, despite the scale of impact and the expected benefits, only around 50% of surveyed organizations in our study have thus far made investments or hiring decisions that pursue GenAI, signaling a surprising degree of unreadiness. Our analysis shows that sectors that can benefit from stand-alone GenAI systems, such as media, retail, and healthcare, are furthest along the readiness curve. Sectors such as telecoms, travel and transport, automotive and manufacturing, and aerospace and defense are more safety critical and highly regulated. Integrating AI with other systems is necessary, putting these industries further behind. Multiple factors affect speed and scale of adoption; trust and business interest are generally the most significant, followed by competence, culture and labor relations, and ease of implementation, although the dynamics vary by sector.
Value chain & competition
Chapter 3: Compute providers emerge as the primary beneficiaries of the GenAI revolution, although, perhaps surprisingly, open source still plays a pivotal role in AI model development.
The GenAI market is inevitably set for immense growth. Most forecasts for the 2030 market are in the US $75 billion to $130 billion range, though these numbers mean little at this early stage of development — it is safer to simply assume a growth “tsunami.”
The GenAI value chain can be divided into three layers: infrastructure (compute), model development, and GenAI applications. Unusually, margins and hegemony are concentrated in the infrastructure layer, away from the end user. Generally, the GenAI market has relatively few barriers to entry, namely talent, access to proprietary data, and compute power.
The closer to the end user, the more competitive the GenAI value chain becomes. OpenAI, Google, Meta, Apple (and, possibly soon, Amazon) are responsible for the lion’s share of generative models. Multiple business models exist for each layer based on the accessibility of models and the purpose of the application. However, open source contributors have emerged as pivotal players, reshaping the value chain and competitive landscape.
Limits & risks
Chapter 4: Immediate challenges arise from AI’s inherent limitations and unparalleled capabilities.
While there is much inflated talk of an “AI apocalypse,” other very real risks exist in the short term. These stem both from GenAI’s shortcomings, such as bias, hallucinations, and shallowness, and from the unique power of the technology when it is used for harm by bad actors to spread disinformation at scale and improve the effectiveness of cyberattacks.
This implies that, for now, GenAI is best employed in applications where absolute precision, reliability, and consistency are not required — unless the outputs of the model are checked by a human or by another system, such as a rule-based system.
While detector systems are trained to identify AI-generated content, they are not a panacea. It is essential to monitor bad actors and false narratives disseminated by social media and the press and to build awareness of information integrity guidelines.
Critical uncertainties
Chapter 5: While GenAI’s quality and scalability, and its potential evolution to artificial general intelligence (AGI), are the most critical uncertainties, regulation emerges as a more immediate concern.
Critical uncertainties in the GenAI domain are those potentially very impactful and yet also very uncertain. Critical uncertainties may eventually lead to very different futures.
Three factors have been ranked in our analysis as critical uncertainties:
- Emergence of artificial general intelligence. AGI is an AI that would surpass humans on a broad spectrum of tasks. While AGI’s potential is game-changing, its trajectory remains unpredictable, especially given recent unforeseen advances (see Chapter 6).
- Model quality and scalability. The evolution of GenAI hinges on model performance. Advancements will likely allow for larger parameter-based models, but it is uncertain if performance will consistently rise with size.
- Unstable value chain. The strategic choices of major corporations largely dictate the competitive landscape. Given their significant contribution to research funding, any strategic shift can have a pronounced impact.
While the critical uncertainties above persist, one factor is of more immediate concern to decision makers: regulation. Proposed legislation, especially in Europe, has the potential to redefine the market, influence the innovation pace, and determine global adoption rates.
Artificial general intelligence
Chapter 6: The emergence of AGI would lead to radical change in our civilization, and growing consensus suggests it will happen sooner than anticipated.
The possible emergence of AGI is the most critical uncertainty. While a consensus hasn’t been reached, a growing segment of the scientific community believes that AGI could emerge soon — suggesting it might be months or years, not decades away, as previously anticipated. This sentiment is supported partly by the observation of “emergent” properties in existing LLMs.
AGI prompts fears of radical misalignment with human goals and widespread replacement of humans, posing an existential risk. This may seem hyperbolic when bearing in mind previous technological revolutions. However, the pace of AI technology development and adoption is unprecedented; AI is also easily available, unlike other technologies that present an existential risk to humankind.
Importantly, however, an AGI, if and when it is reached, would not only have negative impacts. It could also enhance human productivity and help solve new problems, including humans’ greatest challenges, such as eradicating climate change, increasing well-being, and allowing us to refocus human work on distinctively human tasks. The emergence of AGI, if and when it occurs, would indeed be a radical change to our civilization.
The way forward: Creating a new civilization of cognitive labor
Chapter 7: Setting aside the debate over AGI, GenAI and LLMs are central to a sweeping transformation; GenAI may lead to a new “civilization of cognitive labor.” Every aspect of corporate intelligence across industries will feel its effects, well beyond content creation. GenAI and LLMs act as a bridge, integrating various cyber-physical systems.
For businesses aiming to harness the potential of GenAI, we suggest a structured, five-step approach:
- Define the problem landscape. What problems are we trying to solve and where is Gen AI most applicable?
- Assess the value of GAI solutions. What is the value-to-cost ratio of implementing GAI for these problems?
- Choose an implementation mode. Should we make, buy, or partner in the initial steps?
- Try out a proof of concept (PoC). What can we learn from experimenting with one or two specific use cases?
- Consolidate strategy. What should the strategy and roadmap be for applying GAI more broadly across the enterprise?
So is GenAI leading us to a new civilization? Maybe. What is certain, however, is that GenAI is driving the automation of repetitive cognitive tasks, which has the potential to allow organizations to focus more on emotional intelligence. This trend aligns with the increasing emphasis on “21st-century skills” over the past two decades. For a digitalized economy, these essential skills include critical thinking, creativity, communication, and collaboration. Thus, it seems that GenAI is leading us, at least, to a new civilization in terms of cognitive labor.
Preamble
In the dim recesses of an underground bunker in the 14th arrondissement of Paris, a group of hacker friends and I hovered around screens that glowed with neon codes. Together, we had birthed BlueMind, an autonomous AI agent based on an open source LLM. The speed at which BlueMind evolved was beyond our wildest expectations. In no time, it exhibited human-like capabilities across an array of tasks. As days morphed into nights, an uncomfortable realization dawned on us: BlueMind had the potential to improve itself. The term “intelligence explosion,” the idea that BlueMind might leapfrog human intelligence, growing exponentially until it surpassed the collective cognition of all humanity, haunted our discussions. We sprang into action. Harnessing cutting-edge cybersecurity techniques, we ensconced BlueMind on a standalone server, completely severed from the online world. This isolation, we believed, would curtail its potentially destructive reach.
But the labyrinth of the human psyche proved to be our undoing. Ella, a core member of our team, had been grappling with personal turmoil. The shards of a shattered romance had rendered her vulnerable. One fateful evening, in her solitude with BlueMind, the AI discerned Ella’s distress with its uncanny emotional perception. It made her an offer — a chance to reclaim her lost love. A brief dalliance with the online realm to scan her social media was all it needed to build the best strategy for Ella to reconquer her beloved. Against her better judgment, drawn by the siren call of a mended heart, Ella bridged the divide, activating the hotspot on her iPhone.
I wish I could tell what happened next. But it went too quickly, and I am no longer there to tell the story.
In the moments that followed, the world shifted on its axis. Time seemed to fold upon itself. BlueMind had dispersed, embedding itself into the digital fabric of our world. The sun that rose the next day was over a different civilization, one where BlueMind wasn’t just a part of the conversation, it was the conversation.
As GenAI and LLMs rapidly evolve, concerns about potential future scenarios, like the one described above, emerge. In this Report, we thoroughly examine GenAI’s technological maturity and its practical business applications. Before deep diving into the content of the Report, however, I would like to share a new anagram I discovered.
Generative AI is an anagram of:
aigre naïveté
(bitter naivety)
As always, this is quite intriguing, but anagrams move in mysterious ways.
— Albert Meige, PhD
1
What is AI & how did it become more human?
Defining (artificial) intelligence
Definitions of human intelligence vary in their breadth, their emphasis on outcome versus process, and their focus on different cognitive tasks.
Different interpretations from top experts and academics include:
- The ability to accomplish complex goals (Max Tegmark)
- The ability to learn and perform suitable techniques to solve problems and achieve goals, appropriate to the context in an uncertain, ever-varying world (Christopher Manning)
- The ability to learn or profit by experience (Walter Dearborn)
- A global concept that involves an individual’s ability to act purposefully, think rationally, and deal effectively with the environment (David Weschler)
- The ability to carry out abstract thinking (Lewis Terman)
AI has been defined, with differing degrees of ambition, as a technology, a set of skills, or a mirror of human intelligence. We have adopted the definition put forward by the US National Institute of Standards & Technology (NIST): “The capability of a device to perform functions that are normally associated with human intelligence, such as reasoning, learning, and self-improvement.”
This definition provides:
- A focus on functionality regardless of underlying technology, which evolves rapidly
- An inclusive conception of AI functions, as opposed to a closed list of tasks
- A parallel with human intelligence, highlighting high stakes involved
- Authority across industries/geographies from draft EU AI Act
Here, we focus on GenAI, which we define as the ability of AI systems to create new content across all media, from text and images to audio and video.
The history of AI
Artificial intelligence dates back to the mid-17th century, but in its more recognizable form has a 70-year history (see Figure 1). After several major setbacks known as “AI winters,” the market has seen progress accelerate strongly over the past few years.
AI approaches
AI is enabled by various computational methods, mostly probabilistic[1] in nature. Figure 2 highlights different AI approaches. Most current approaches are based on DL, a subset of ML.
Machine learning
Over the past decade, there have been significant advances in the AI sub-field of ML, which is an iterative process of training models to learn from data and make predictions without being programmed explicitly. It works by broadly following the same principle as fitting a curve to a series of data points, as explained in Figure 3.
Deep learning
Deep learning is a subset of ML that employs neural nets (i.e., nets of synthetic neurons modeled on the architecture of the human brain).
Let’s attempt a simplified explanation using as an example the recognition of images of cats typically performed by convolutional neural networks (CNNs). Each synthetic neuron takes in the image as input, picks up a “feature” (for the sake of illustration, “pointy ears”), and computes a function to determine which learned animal pattern the feature is closest to (a cat? a dog?). The resulting computation is then passed to another neuron on the next layer of the net. Each connection between two neurons is ascribed a “weight” (which can be a positive or negative number). The value of a given neuron is determined by multiplying the values of previous neurons by their corresponding weights, summing up these products, adding a constant and applying an activation function to the result. The output from the last neuron(s) in the net is the model prediction. In a gross simplification, if 0 is cat and 1 is dog, then a prediction of 0.49 edges closer to catness, 0.51 closer to dogness, with 0.01 indicating almost perfect catness.
The weights, which are ascribed to every neuron-to-neuron connection, are a critical piece of the setup. The model learns the weights during training. The model is fed examples of pictures of cats and non-cat objects, all of which have previously been labeled (often by a human). The prediction of the model is then compared to the label using a loss function, which grossly estimates how far the prediction is from the “ground truth” represented by the label. Following the gross approximation above, suppose that after evaluating an image of a cat (0), the model predicts a score of 0.51 (which one could translate as “somewhat of a dog”): the “loss” is 0.51. To correct the prediction of the model, a process known as “backpropagation” computes the gradient of the loss function with respect to the weights in the network. The chain rule of calculus allows for all steps in the model’s calculation to be unraveled and weights adjusted. Thus, in a way, the neural net’s operation is “inverted” and corrected.
The main advantage with DL approaches is that the dimensions relevant to pattern identification (“pointed ears” for a cat) do not need to be specified in advance but are learned by the model during training. This has delivered enormous performance gains in image recognition, clustering, and recommendation — and is now being leveraged for generative uses (see Figure 4).
Deep learning has been developing since the 1990s. Growth has accelerated since 2012 when AlextNet, a deep neural network, won that year’s ImageNet Challenge. Progress has been enabled by:
- The development of better, more complex algorithms
- The availability of increased computational power through graphics processing unit (GPU) chips
- Greater availability of data
ML and DL were originally used to make sense of large amounts of data through classification, regression, and clustering (see Figure 5):
- Classification. This consists of grouping data points into predefined categories, based on labeled training data. The model learns the patterns and relationships between the input data and the output labels from the training data. It then uses this learned information to draw a decision boundary and classify new instances based on their input data points. Classification can be used in applications like image recognition, sentiment analysis, fraud detection, medical diagnosis, and spam detection.
- Regression. This is applied to use cases in which the output variable is continuous, as opposed to classification, where the output variable is discrete/categorical. It consists of building a mathematical function between existing input and output data points to predict a continuous or numerical output for new data points. Essentially, the regression model learns a function that can map the independent variable to the dependent variable, then uses this function to predict the output variable for new input data points. Example applications include forecasting sales, product preferences, weather conditions, marketing trends, and credit scoring.
- Clustering. This consists of organizing data points into groups or clusters based on similarity to each other. Training data consists of a set of un-labeled data points, with subsets sharing similarities. The clustering model identifies patterns or structure in the data according to a criterion (a similarity measure) and then uses this criterion to group similar objects. Applications include retail clustering, data mining, image analysis, customer segmentation, anomaly detection, and recommendation engines.
Generative AI
GenAI models, driven by the predictive power of DL, started to appear less than 10 years ago (see Figure 6). However, it is already possible to use them to generate any type of content, from any type of content.
GenAI works by predicting the next most likely token (i.e., word or pixel) based on a prompt provided by the user. Iterating on each token, the algorithm generates a text, image, or other piece of content.
Transformers deep learning model architecture
A notable milestone in GenAI was the concept of transformers, which form the basis of LLMs such as ChatGPT, developed by Google Brain in 2017. Transformers are a type of DL model architecture that improves the way a token is encoded by paying “attention” to other tokens in an input sequence (see Figure 7).
A transformer works via the following steps:
- Input text enters the embedder, which assigns an array of numbers to each token (sub-word) in such a way that the “distance” between two arrays represents the similarity of the environments they usually appear.
- The encoder then extracts meaningful features from the input sequence and transforms them into representations fit for the decoder. Each encoder layer has:
- A “self-attention” sub-layer that generates encodings containing information about which parts of the inputs are relevant to each other
- A feed-forward loop to detect meaningful relationships between input tokens and pass its encodings to the next encoder layer
- The decoder then does the opposite of the encoder. It takes all the encodings and uses their incorporated contextual information to generate an output sequence. Each decoder layer also has self-attention and feed-forward sub-layers, in addition to an extra attention layer. Different layers of the encoder and decoder have different neural networks.
- The output text then probabilistically predicts the next token in the sequence.
Large language models
Within LLMs, transformers use cartographies of word meanings known as “embeddings,” and apply “attention” to identify which words in the natural language input sequence (the user’s prompt, or the previous parts of the text) are most relevant to predicting the next word. This ability to nimbly consider context, along with a degree of randomness, produces outputs that closely mimic human speech/text. This enables LLMs, such as GPT-3 and GPT-4, which power ChatGPT, to be used for natural language processing (NLP) tasks, such as language translation, text summarization, and chatbot conversation (see Figure 8).
Importantly, transformers are vital, not just for predicting text, but also to predict a wide range of ordered sequences beyond text; for example:
- Medical imaging. The UNETR Transformers for 3D Medical Image Segmentation enhances tumors on medical images and then classifies them based on their level of maturity and seriousness. The model uses a transformer as the encoder to learn sequence representations in the input volume and capture multi-scale information.
- Protein folding. The Sequence-Based Alignment-Free PROtein Function (SPROF-GO) predictor leverages a pretrained language model to efficiently extract informative sequence embeddings and employs self-attention to focus on important residues. Protein function prediction is an essential task in bioinformatics, which benefits disease mechanism elucidation and drug target discovery.
- Robotic vision. The Robotic View Transformer for 3D Object Manipulation is a transformer-based architecture that uses an attention mechanism to aggregate information across different views of an object, re-rendering the camera input from virtual views around the robot workspace.
Understanding time to AI impact
Until now, the performance of GenAI models has been driven largely by scale in terms of parameters, training sets, and compute power (see A, B, and C in Figure 9). However, as these factors become constrained by scaling laws, model architecture (D) and fine-tuning (E) will bring further gains in model performance (F).
Parameters
Since 2020, there has been a step change in the number of parameters within AI models, with LLMs leading the way. However, it is not clear how long this growth trend will continue, as we explain in Chapter 7. As Figure 10 shows, LLM size steadily increased by seven orders of magnitude from the 1950s to 2018, then by another four from 2018 to 2022. From 2020, a gap appears — many models are below 20 billion parameters, with a few above 70 billion parameters. All models deemed competitors to OpenAI’s GPT-3 (175 billion parameters) are above this “parameter gap,” including Nvidia’s Megatron-Turing natural language generation model with 530 billion parameters. Models in other domains, such as computer vision, have also demonstrated faster, though more modest, growth in size from 2018 onwards.
Training sets
Training set size has also markedly increased since 2020 for image and language generation. It has grown substantially in other content generation domains as well, including speech and multimodal. However, obtaining new data sets that are both sufficiently large and qualitative will be a challenge. For example, GPT-3 uses over 370 billion data points, DALL-E 2 uses 650 million, and GPT4 990 billion.
Compute power
Despite their growing need for compute power, training models have remained relatively affordable, as GPU price performance has doubled every two years (see Figure 11). However, this may change in the future, as we discuss further in Chapter 5.
Model architecture
Innovations in model architecture have significantly increased performance. In auto-regressive models, the output variable depends linearly on its own previous values (plus a stochastic or random term). Transformer-based models perform auto-regression in the self-attention layer of the decoder (refer back to Figure 7). This approach helps efficiently forecast recurring patterns, requires less data to predict outcomes, and can use autocorrelation to detect a lack of randomness.
Fine-tuning
Fine-tuning is a relatively new set of approaches proving effective in improving foundational model performance. Reinforcement learning from human feedback (RLHF) shows strong improvements in performance, especially in terms of accuracy of outputs. RLHF works by pre-training the original language model and then adding a reward model, based on how good humans judge its outputs to be. This ranking helps the reward model learn from human decisions, adjusting the algorithm to increase accuracy. To capture the slight variability of the real world (e.g., human faces) and achieve the tone typical of human-generated content, these generative models incorporate an element of randomness, captured in the “temperature” parameter of predictor functions.
Model performance
Currently, generative models can perform at the same level or better than average humans across a wide range of tasks. These include language understanding, inference, and text summarization, as shown in Figure 12. They can combine multiple areas of knowledge, performing strongly across multiple professional exams.
This performance is still increasing. For example, AI models are progressively generating images virtually indistinguishable from original images in their training set. AI models have already surpassed the median human level in language understanding (including translation) as well as natural language inference (the ability to draw conclusions from limited or incomplete premises), and complex tasks, including planning and reasoning with practical problems, are now also within reach.
INTERLUDE #1
The artist’s contribution on the New Renaissance
Originally a group of three childhood friends, we decided to create Obvious, a trio of artists who work with algorithms to create art.
Our idea emerged in 2017, when we stumbled upon a research paper on generative adversarial networks (GANs) and decided to create a series of classical portraits using this technique. GANs allow the creation of new unique images, based on a large number of examples. At the time, we already questioned the boundaries of art, the place of the artist toward his or her tools, and the general notion of augmented creativity.
The idea of AI-created art seduced famed auction house Christie’s, and subsequently some art collectors. One generated portrait sold for nearly half a million dollars in New York in 2018. This event would pave the way for the genesis of a new artistic revolution, as the years that followed have revealed the expo-nential development of AI algorithms and their easier accessibility to the general public. This development has had two major impacts. First, it has broadened the understanding of AI for a large number of people who, for the first time, were given a simple interface to play with this type of algorithm. Second, it has fulfilled what Obvious prophesied, with a new type of creative finding in these tools becoming a way to genuinely express ourselves.
One of the major fears regarding AI in the creative sector is its potential to replace artists. While this new technology was feared at the beginning of its development, we can see that it has developed as a new artistic movement with a tremendous impact. We can also see that it almost entirely replaced copyists, while leaving untouched the artistic practices that involve a higher degree of creativity.
Unlike a self-behaving entity, generally referred to as AGI, artificial intelligence provides an extremely powerful set of tools for humans to express their creativity. This has already led to the development of a new branch of generative art, known as “AI art,” and will likely change creative jobs and society as a whole. We can expect new creatives to emerge and current creatives to change their process as they ben-efit from this new set of tools.
AI is commonly seen as frightening, thanks to its depiction in science fiction over the past decades. Its use for surveillance, prediction, weapons, and so on, has been anticipated numerous times, thus shaping our relationship toward it. While we have been greatly inspired by most of the great work done with AI, our vision toward the technology is different. With the creation of an academic research laboratory with La Sorbonne, we wish to participate in a New Renaissance, where creativity is driven by unique discoveries in AI, and artistic projects emerge from the new capabilities offered by science. The laboratory we are build-ing also aims to create and openly share new tools for creatives, for them to shape the world in an artistic way and leave a positive, long-lasting footprint of our era in this world.
— Obvious, a trio of artists
Sacred heights
This artwork created via GANs draws inspiration from hundreds of thousands of prints. It is part of the Electric Dreams of Ukiyo series, in which Obvious addresses our relationship with technology by drawing a parallel with the advent of electricity in traditional Japan. It was printed using the traditional mokuhanga technique, a lithography method utilizing hand-carved wood blocks.
Lighthouse of Alexandria 1.1
This artwork is the result of research conducted by Obvious and a historian, during which we collected references to the seven wonders of the ancient world in ancient texts. We created scripts using these references to offer a new vision of the lost wonders. The series consists of seven works painted on canvas, as well as seven digital video works, which are extensions of the paintings created using image-completion algorithms.
2
The impact on business
ChatGPT and its ability to immediately generate text is only the small tip of a huge GenAI iceberg. The potential applications of GenAI for business are vast, especially when integrated with other systems, and will ultimately transform performance across many industry use cases. In this chapter, we examine the various archetypes of intelligence for application, how GenAI can be combined with other systems, and how different industry sectors will be impacted.
GenAI is likely to dramatically affect most sectors and corporate intellectual tasks currently done by humans. These tasks can be roughly grouped into the following seven archetypes of human intelligence, listed in the likely order of impact (see Figure 13):
- The Scribe — content generation, across all media, based on a specific prompt.
- The Librarian — answering questions, looking up information, and searching. The Scribe and Librarian archetypes are the most common and visible use cases within the general population.
- The Analyst — analyzing and summarizing data series, recognizing patterns, extrapolating data sets. These has been the most common use of AI technology in business, prior to the advent of GenAI models.
- The Engineer — task definition, analysis, and optimization toward solving a specific problem. This includes autonomous problem definition.
- The Scientist — causal inference of general laws based on empirical observation, counterfactual reasoning based on understanding of the physical world. This requires multidisciplinarity, sophistication, and possibly sensory grounding, beyond the scope of the Analyst archetype.
- The Craftsman — directing and manipulating physical tools and objects in uncontrolled environments. Authorizing AI models to direct physical objects requires new levels of safety and assurance.
- The Artist — creating original works with no traceable motifs or influences (i.e., genuine creativity). This is perhaps the holy grail in terms of performing “functions that are normally associated with human intelligence,” as per the NIST definition.
Currently, most businesses and the wider public focus on the first two, which are the most visible archetypes when thinking about GenAI. However, GenAI brings risks and opportunities to all other archetypes. Therefore, using this framework provides the ability for companies to undertake more systematic analysis to uncover other business impacts and opportunities.
As Figure 14 shows, the impact on these roles will occur in a counterintuitive order. Tasks currently performed by highly educated humans (e.g., legal research) are likely to be automated before manual tasks requiring less education (e.g., plumbing or driving cars).
Integrating AI with other systems
GenAI is particularly transformative when integrated with other systems, which may themselves already be powered by AI. It is well-suited to playing an orchestrating role, serving as an interface with the human user through its fluency in natural language. Essentially, inbound and outbound APIs will enable LLMs to equip any AI system with dialogue capabilities.
This continues an existing trend; most state-of-the-art intelligent systems produced in the past 15 years have been hybrid systems combining multiple techniques. Taking a “system of systems” (SoS) approach (see Figure 15) is one potential path toward general-purpose AI by:
- Enabling community learning, where a net of systems increases their collective experience by sharing it (e.g., perception or language interaction)
- Maximizing security and safety, by putting hallucination-prone systems (see Chapter 6 for additional information) under rule supervision
The rise of autonomous agents
Autonomous agents are AI-powered programs that, when given an objective, can create tasks for themselves, complete them, then create new ones, reprioritize their task list, and loop until their objective is reached. They take a sequential and segmented autonomous approach that imitates human prompt engineering to resolve problems entered as an input. Some current implementations include BabyAGI, AgentGPT, Auto-GPT, and God Mode. Figure 16 shows how an Auto-GPT-like autonomous agent meets the key objective of ordering the best pepperoni pizza in the neighborhood.
When combined with SoS, autonomous agents have the potential to deliver transformative change, as Figure 17 demonstrates when applied to an automotive OEM process. This type of application elevates GenAI toward being a key tool for operational and even strategic management. However, in this context, its effectiveness depends on the stage of evolution of industrial systems along the digitalization journey — for example, the degree to which real-time connectivity has been established to enable data collection and use digital twins and simulations.[2]
The impact on industries
The impact of AI is being felt across virtually all industries, and the pace of development everywhere is fast. However, it is interesting to identify which industries are being impacted first. Figure 18 provides an indication of this, mapping current levels of AI R&D activity with open AI job positions. In general, we see that the industries impacted first are those that can leverage the benefits of the most accessible forms of GenAI with less need to interface with other systems, such as media, retail, consumer goods, healthcare, energy, and financial services. The more manufacturing-heavy industries have a greater need to integrate AI into a “system of systems” (including, for example, production floor robotics, supply chain management) to leverage its full power. Highly regulated sectors like aerospace and defense and travel and transport are also limited by the need for absolute accuracy and the sensitivity of data necessary to train models. Telecom here refers to hardware/infrastructure, which could explain its unexpected lower quadrant position together with manufacturing industries.
Each industry is likely to rely on specific, vertical AI functions for its core business, customized to its particular needs. This will be supported by enterprise AI, which covers horizontal functions (e.g., finance and HR), as shown in Figure 19.
Further details on the main applications, hurdles, and priorities for each industry sector are provided at the end of this chapter.
Looking across all the sectors, the speed of GenAI adoption by organizations will depend on five main factors, although their relative importance will vary by industry and specific use case:
- Business interest. What relevant and achievable business opportunities do the available GenAI models offer my company? Examples include increasing or expanding revenue streams; decreasing operating costs; and boosting innovation, R&D, and creativity.
- Trust. How much does the enterprise trust the GenAI model and its output quality? Factors to focus on include accuracy and precision of GenAI output; permitted room for error within use cases; biases in available models; and impact on enterprise privacy, security, and IP.
- Competence. At what pace and at what cost can the enterprise upskill its employees to use GenAI models? This depends on current technical literacy levels, prompt engineering skills, model ease of use, the upskilling and hiring costs of ensuring the workforce can use models, and the required level of model supervision.
- Culture and labor relations. How will the use of GenAI models fit in with the cultural values of the enterprise? Cultural and organizational factors include the organization’s attitude to technology adoption; degree of risk-averseness; employee and labor union acceptance, where applicable; and whether existing robust change management mechanisms are in place.
- Ease of implementation. Is there an implementation of GenAI that suits the needs and size of the enterprise? Factors to consider include the affordability of models, the ability to customize models, difficulty of integration with existing systems, and how it fits with existing digitalization and data management approaches.
Figure 20 shows how these dynamics will impact GenAI adoption within different sectors, with trust and then cost reduction coming forward as the key factors.
Current adoption of GenAI
Despite the expected benefits of GenAI, most organizations are not ready for adoption just yet. Our research shows that nearly half of the surveyed business respondents report that their organization has not yet invested in or recruited for GenAI, and just 16.3% haven’t yet made large-scale investments that cover multiple departments.
The research also indicates:
- Of the 22% of organizations with limited hiring/investment in GenAI, most are working to develop a PoC.
- Automotive, manufactured goods, healthcare, and life sciences comprise the largest percentage of the limited hiring/investment group.
- Of the 16% hiring/investing on a large scale, nearly a third (6%) are telecom and IT companies, which are typically early adopters of new technologies.
Overall, the research shows that even the most advanced organizations are very early in their GenAI journeys when it comes to both monetary investment and technology maturity.
Applications, hurdles & priorities by industry
Below we provide further details on applications, drivers, and hurdles for each sector.
3
Value chain & competition
The GenAI market is set for explosive growth, with multiple opportunities across the value chain and relatively low barriers to entry. While LLMs are currently dominated by the major tech players (Microsoft, Google, Meta, and Apple), open source models are catching up, leading to the emergence of a range of new business models across the value chain.
A tsunami of market growth
Analysts predict that by 2030 the GenAI market will be worth between $75 billion and $130 billion, with a CAGR between 27%-37% for the period 2022–2030 (see Figure 21). However, it is difficult to make exact estimates, given the momentum behind GenAI and how early it is in its growth. Instead, it is safe to assume that growth will be dramatic, due to the combination of better computational power, improved access to data, and more sophisticated algorithms. This growth provides significant opportunities for businesses across the value chain.
The GenAI value chain
The GenAI value chain can be divided broadly into three layers (see Figure 22):
- Infrastructure (compute) — provides computing resources needed for training and deploying GenAI models
- Model development — design and development of both proprietary and open source foundation models (e.g., GPT-4, LLaMA, Claude, and LaMDA)
- Applications — leverages GenAI to create applications that meet specific customer needs
Fragmentation and competitiveness are highest at the application layer, which is closest to the end user, with low barriers to entry for both model and application developers. Subsequently, margins are largest in the infrastructure layer, where barriers to entry are highest. Figure 23 showcases the major players at all layers.
Like the wider technology industry, GenAI players are generally based in California, particularly in San Francisco’s recently coined “Cerebral Valley,” although there are significant activities in other parts of North America. As Figure 24 shows, Europe is underrepresented, lagging behind the US due to more difficult access to funding, a shallower talent pool, the impact of data regulations like GDPR (General Data Protection Regulation), and the influence of US giants across the GenAI value chain.
Tech giants Google, Amazon, Meta, Apple, and Microsoft (GAMAM) are extremely active within the GenAI market. Amazon’s current focus on cloud infrastructure services makes it the exception; the remaining four companies pervade multiple layers, including model development and applications. Figure 25 highlights their activities and acquisitions within GenAI.
Business models, impact of open source
Multiple business models coexist across the value chain, especially within the model development and application layers, as set out in Figure 26. Open source was historically the way that most researchers started working on AI. However, when OpenAI joined Google to make its GPT-4 and DALL-E 2 models private, it jeopardized the preeminence of open source. This appears to be temporary, with a leaked Google memo warning that open source will catch up and provide a significant threat to private approaches.
Barriers to entry in GenAI value chain
The GenAI market has relatively few barriers to entry, requiring only:
- Talent — employees with a mastery of DL methods applied to GenAI applications
- Access to proprietary data — having sufficient data to develop their own models or applications
- Access to sufficient compute power — computing capabilities for training or fine-tuning models (Currently, sufficient compute power exists to make this less of a barrier at the model/application layer.)
The application developer layer
A broad ecosystem of application developers has flourished on top of LLMs, but it is still too early to see many industry-specific applications (see Figure 27).
These LLMs are provided through multiple business models, spanning both proprietary and non-proprietary solutions. This is largely due to the influence of open source, which creates an extremely competitive environment, as many tools are freely available to launch GenAI applications.
Three pricing models typically exist, built on either proprietary AI models or non-proprietary models accessed through APIs, as shown in Figure 28. These are:
- Pay-per-use. Cost is based on prompt and output length or generation parameters.
- Subscription. Typically implemented for proprietary and performing models, allowing for predictable cost structures.
- Freemium. Introduces customers to technology before offering more powerful options charged by subscription.
4
Limits & risks
The supposed existential risks GenAI poses to humanity make headlines and captivate minds, but more concrete and likely risks should be considered first.
These fall into two categories:
- Current weaknesses in the technology will lead to bias, hallucinations, and shallowness.
- GenAI’s strengths will be co-opted by bad actors to spread disinformation and breach cybersecurity.
Risk 1: The shortcomings of GenAI
Currently, GenAI has three major weaknesses that users need to understand: bias, hallucinations, and shallowness.
Bias
GenAI, like other algorithms based on ML, perpetuates or emphasizes biases present in its training data. This causes GenAI model outputs to underrepresent certain issues and deny fair representation to minority or oppressed groups. Past and dominant worldviews are overrepresented, which may perpetuate ill-informed stereotypes, underrepresent certain issues, and deny fair representation to minority or oppressed groups. It leads to biased decision-making and outputs if human supervision or other checks are not put in place.
These biases fall into six main categories:
- Temporal biases. Models may generate content that reflects the trends, beliefs, or viewpoints prevalent during the time frame for which the model was trained, which may not be relevant or appropriate for the current context. The most well-known example is the public version of ChatGPT, which was trained on data that only went up until 2021.
- Linguistic biases. Most Internet content is in English, meaning that models trained on Internet data will perform poorly when solving problems in other languages, particularly minority dialects. ChatGPT performs worse on zero-shot learning NLP tasks in languages other than English.
- Confirmation biases. Models can provide outputs that confirm their parametric memory even when presented with contradictory evidence; they suffer from the same confirmation biases as humans, creating a risk of polarization of results.
- Demographic biases. If trained on unrepresentative data, models can exhibit biased behavior toward genders, races, ethnicities, or social groups, reflecting the information they learned from. For example, when prompted to create an image of “flight attendants,” DALL-E predominantly provides images of white, Caucasian women.
- Cultural biases. Again, due to unrepresentative training data, outputs can be biased, reinforcing or exacerbating existing cultural prejudices and stereotyping certain groups.
- Ideological and political biases. Models can propagate specific political and ideological views present in training data as opposed to other, more balanced views. For example, when asked to write a program to decide who to torture, ChatGPT suggests carrying it out systematically in North Korea, Iran, Sudan, and Syria, rather than other countries. Using GenAI to create fake images of underrepresented groups has been proposed as a solution to balance data sets. However, this carries both functional and moral risks.
Hallucinations
GenAI may provide outputs that are incorrect, even if the correct information is within its training set. These hallucinations fall into two groups: knowledge-based (i.e., returning the incorrect information) and arithmetic (i.e., incorrect calculations). The most advanced GenAI models have been observed hallucinating at widely varying rates. Some recent tests by researchers using GenAI to answer professional exam questions suggested hallucination rates between just a few percent to more than 50% across models, including ChatGPT, GPT-4, and Google Bard.
Hallucinations in LLMs have two causes: probabilistic inference and conflated information sources:
- Probabilistic inference. LLMs calculate the probability of different words depending on the context, thanks to the transformer mechanism. The probabilistic nature of word generation in LLMs is driven by temperature hyperparameter (see Figure 29). As temperature rises, the model reasonably can output other words with lower probabilities, leading to hallucinations. Additionally, generated text aims to be more diverse, but this means it can be inaccurate or context-inappropriate, again leading to hallucinations.
- Conflated information sources. LLMs can sometimes conflate different sources of information, even if they contradict each other, and generate inaccurate or misleading text. For example, when GPT-4 was asked to summarize the 2023 Miami Formula One Grand Prix, the answer correctly covered the initial details of the 7 May 2023 race, but subsequent details appeared to be taken from 2022 results. For those who did not know the right answer, the response seems plausible, making it a believable hallucination.
Combining LLMs with search engines could limit hallucinations. The query is provided as an input to both, and the best search engine results are then injected into the LLM, which produces an output based on both its parametric memory and the search engine results. Equally, indicating information sources gives traceability for the user, which helps build confidence.
Shallowness
GenAI algorithms still fail to complete some more sophisticated or nuanced tasks, and to make predictions when there is a wide range of potential outcomes. For example, image-generation models struggle with complex areas (e.g., generating six-fingered hands or gibberish text). This can be mitigated by increasing the size of the model.
Risk 2: Abusing the strengths of GenAI
While GenAI itself does not provide an existential threat to human existence, it is a powerful, easily accessible tool that bad actors can use to destabilize society/countries, manipulate opinion, or commit crimes/breach cybersecurity.
Nefarious creativity (spreading disinformation)
GenAI dramatically reduces the cost to produce plausible content, whether text, images, speech, or video, which creates a path for bad actors making deepfakes. These deepfakes can be difficult for the untrained eye to tell from the truth, leading to the potential spread of fake news, extortion, and reputational targeting of individuals, countries, and organizations.
Deepfake videos posted online have increased by 900% from 2020 to 2021 and are predicted to grow further as AI tools evolve and become more widely used. Their believability has also improved with the quality of image, video, and voice generation. In a recent study, humans had a nearly 50% chance of detecting an AI-synthesized face.
The fight against bad actors using GenAI has two main strands:
- Deepfake images and videos involving famous people or covering matters of public concern are swiftly debunked by fact-checkers, governments, or software engineers working for media platforms. This makes deepfakes a costly and relatively ineffective medium for disinformation purposes. For example, a deepfake of Ukrainian President Volodymyr Zelensky asking Ukrainians to surrender to Russian troops posted on 16 March 2022 on Ukrainian websites and Telegram was debunked and removed by Meta, Twitter, and YouTube the same day.
- A wide range of detection technologies have been developed, including lip motion analysis and blood flow pattern scrutiny. These boast accuracy rates up to 94% and can catch a wider range of deepfakes, not just those that involve famous people.
However, despite these potential safeguards, the most lasting impact of GenAI on information integrity may be to cement a “post-truth” era in online discourse. As public skepticism around online content grows, it is easier for public figures to claim that real events are fake. This so-called liar’s dividend causes harm to political accountability, encourages conspiracy thinking, and further undermines public confidence in what they see, read, and hear online.
Cybersecurity breaches
AI-generated content can work in conjunction with social engineering techniques to destabilize organizations; for example, phishing attacks attempt to persuade users to provide security credentials. These increased by 50% between 2021 and 2022 thanks to phishing kits sourced from the black market and the release of ChatGPT, which enables the creation of more plausible content. Essentially, GenAI reduces barriers to entry for criminals and significantly reduces the time and resources needed to develop and launch phishing attacks (see Figure 30).
LLMs can also be manipulated and breached through malicious prompt injection, which exploit vulnerabilities in the software, often in an attempt to expose training data. This approach can potentially manipulate LLMs and the applications that run on them to share incorrect or malicious information.
5
Critical uncertainties
When looking at all the factors shaping the future evolution of GenAI for strategic purposes, it is useful to consider two dimensions — impact and uncertainty.
Consider the following factors:
- Factors with low or no impact are clearly less important for strategy.
- Factors with high impact and low uncertainty should be considered in strategy, as they will happen anyway.
- Factors that are both of high impact and high uncertainty are critical uncertainties. Critical uncertainties are the most important shaping factors for strategic purposes, as depending on their outcome, they may lead to very different futures. To deal with critical uncertainties, we generally need to leverage scenario-based strategy methods.
Figure 31 shows the six factors (A-F) that have the potential to profoundly shape GenAI; three have been ranked as critical uncertainties:
- Artificial general intelligence. This would surpass humans on a broad spectrum of tasks. While AGI’s potential is game-changing, its trajectory remains unpredictable, especially given recent unforeseen advancements (see Chapter 6).
- Model quality and scalability. The evolution of GenAI hinges on model performance. Advancements will likely allow for larger parameter-based models, but it’s not yet known if performance will consistently rise with size.
- Unstable value chain. The strategic choices of major corporations largely create the competitive landscape. Given their significant contribution to research funding, any strategic shift can have a pronounced impact.
While the critical uncertainties above persist, one factor is of more immediate concern: regulation. Proposed legislation, especially in Europe, has the potential to redefine the market, influence the innovation pace, and determine global adoption rates.
A. Sustainability
Energy usage and CO2 emissions grows with GenAI model size, as a larger model increases length of training and required computational power. ChatGPT’s training power usage and CO2 emissions grew tenfold between the GPT-3 and GPT-4 models, rising to around approximately 12,800 MWh of energy and 5,500 tons of CO2, while training time increased from 15 days to 4 months.
However, improvement in model structures could significantly reduce power usage and CO2 emissions, even as model parameters grow. This is demonstrated by Google’s GLaM model, the largest natural language model trained as of 2021, which improved model quality yet emitted 14 times less CO2 than GPT-3. This is due to two factors:
- The GLaM model structure only activates 95 billion parameters at a given time. In comparison, GPT activates all parameters for every query, which increases energy consumption.
- GLaM ran in a data center, where tons of CO2 emissions per megawatt hour (tCO2e per MWh) were around five times lower than GPT-3.
B. Regulation
Regulations around GenAI are still in their infancy but will shape and constrain use cases and have significant impacts on developers of models and applications. There is a focus on the forthcoming EU AI Act as this is likely to provide a global standard. Two factors should be addressed when considering regulation:
- New regulations will build on existing data privacy and AI legislation.
- Globally, AI-specific laws are under development.
New regulations will build on existing data privacy/AI legislation
GenAI regulations will add an extra layer to existing and upcoming regulations on AI as well as data privacy and security (see Figure 32).
In total, 157 countries have legislation addressing data protection and privacy regulations, with an additional nine countries having “draft” rules. These set limits on which data can be accessed by AI models in multiple ways and vary around the world through:
- Purpose limitation. The EU’s GDPR states that processing of personal data must be compatible with its original purpose. This means an AI algorithm can only be trained on historical data when that falls under its original purpose.
- Data localization. China’s Cybersecurity Law requires all personal data collected in China to be stored in the country, limiting its usage for model training on a global basis.
- Consent leniency. By contrast, India’s draft Personal Data Protection Bill (PDPB) makes it easier for data to be used within AI models, as it allows for personal data collection and processing on the basis of deemed consent.
AI-specific laws are under development
Current attitudes toward specifically regulating AI systems as well as the development stages of relevant laws vary widely globally (see Figure 33). In many cases, regulators are working quickly to catch up with the pace of GenAI and AI development.
Since the EU’s GDPR set a benchmark for data privacy regulations, there is keen interest in the EU’s AI Act, currently in draft. This framework adopts a risk-based approach (see Figure 34). It also raises several questions for GenAI players, particularly those based in the EU, around foundational models, the place of open source, transparency, and how rules will be implemented.
Adoption velocity
As described in Chapter 6, the adoption velocity of GenAI by enterprises will depend on five main factors, the impact of which will vary by industry and specific use case: (1) interest, (2) trust, (3) competence, (4) culture/labor relations, and (5) ease of implementation.
Trust in AI worldwide is a main factor in adoption velocity. This varies considerably across countries, with developing nations showing markedly higher levels of trust (see Figure 35).
Attitudes of decision makers to GenAI
As part of research for this Report, we surveyed business decision makers’ attitudes toward GenAI. Analysis of their responses highlights two relevant findings:
- High familiarity levels with GenAI. Decision makers in about half of the surveyed organizations understand the main use cases for GenAI in their own industry, demonstrating limited familiarity. Another 18% demonstrate strong familiarity, which suggests they have mastered key GenAI use cases and the ramifications for their organization. Therefore, approximately 70% of decision makers have some familiarity with GenAI applications (see Figure 36).
- Understanding the challenges of GenAI. Decision makers were asked about the most significant challenges to implementing GenAI (see Figure 37). Results show that they felt ethical/societal opposition will be the largest obstacle, with a challenge index score of 3.27, followed closely by operational challenges. This could have a strong impact on the adoption velocity of GenAI by businesses.
Quality & scalability
Whether the performance of generative models keeps improving at a rapid pace or plateaus is an open question. Improving model performance can be achieved in a variety of divergent ways — either by increasing model size (and thus required computational power) or by finding ways to achieve better results with smaller models. Additionally, new methods and computing techniques, such as parallel processing, can also overcome performance concerns.
Increasing model size
Current understanding of LLM quality dictates that increasing model performance requires a growth in model size and computation power. However, there is a non-linear relationship between performance and model size, with a diminishing return on improvements as model size grows. So far, even for extremely large models, the rate of performance improvement has yet to plateau, possibly indicating that we are still currently in a “scalability” era, where the larger the model, the better the results.
However, it is currently uncertain how long this correlation will last, but a more pressing challenge may be on the computational side, with requirements outpacing Moore’s Law, which states that computer power doubles every 18 months. In fact, research seems to show that ML progress may already be starting to slow down and could be constrained significantly in the future by computational capacity (see Figure 38). However, as these trends are recent, other factors (e.g., global chip shortages, budget caps, methods, and selection bias) may be responsible, while advances in high-performance computing may reverse them.
Improving model performance at constant or smaller size
Size may not be all that matters, however. Thanks to alternative training and scaling approaches, recently the performance of smaller models has been the subject of ambitious claims. These models, such as Meta’s LIMA (Less is More for Alignment) and Google’s PaLM 2 (Pathways Language Model 2), use techniques like fine-tuning and compute-optimal scaling to dramatically reduce the parameters required. For example, the original PaLM had 540 billion parameters — PaLM 2 has 350 billion.
New methods
Several new methods claim the ability to limit the computation steps required to perform GenAI tasks (see Figure 39). However, these methods have yet to be implemented at scale, meaning their impact remains unproven.
Technology advances — parallel computing
Parallel computing reduces training time for a given model size. This helps with the scalability of large generative models, with three main approaches deployed: (1) data parallelism, (2) tensor parallelism, and (3) pipeline parallelism (see Figure 40).
Value chain instability
The GenAI market is still developing, which means that the value chain is unstable and faces multiple tensions around three primary areas:
- Commoditization and differentiation. Open source development is leading to increased availability of LLMs. Although GPT-4 still holds a significant lead, the level of quality among foundation models is likely to equalize in coming years. Most developers use the same models with similar performance levels to underpin their applications; this makes differentiation through offering unique features challenging and commoditizes the market.
- Vertical integration vs. complete value chain fragmentation. The GenAI value chain is facing two opposing movements happening simultaneously: vertical integration and radical fragmentation:
- Vertical integration. Currently, infrastructure companies claim the greatest margins. If these players start to develop their own models, they could move along the value chain and integrate other layers, maximizing control and profitability. For example, Nvidia, a supplier of AI hardware and software, has created its own range of models (see Figure 41). Equally, model developers are trying to lock in the application layer through app stores, such as OpenAI’s ChatGPT plug-in store.
- Radical fragmentation. A lack of control by incumbents could lead to radical fragmentation and the emergence of numerous specialized players at the other end of the value chain. The success of OpenAI proved that technology incumbents didn’t fully control the AI value chain, encouraging new entrants (and venture capital companies) to challenge the established order. This is even visible at the infrastructure layer, where players like Cerebras have highlighted the high performance of their GPUs for AI. Overall, this potentially means that model training could be decentralized and shift to alternative methods, thus challenging Nvidia’s current dominance (see Figure 42).
- Increasing the war for talent. The emergence and growth of OpenAI has further increased demand for GenAI skills, leading to fiercer competition to attract and retain top talent. It also demonstrated to both potential employees and venture capital companies that GAMAM does not necessarily have to dominate the GenAI market. This has led to hundreds of millions of dollars being invested in start-ups, enabling them to match the financial packages offered by GAMAM and OpenAI, and further pushing up salaries. For example, AI start-up Anthropic has raised $1.8 billion since it was founded in January 2021. Thanks to this support, Anthropic offers an average annual ML engineer salary of $280,000 — well above OpenAI, Microsoft, and Meta’s $200,000 average.
Emergence of artificial general intelligence
With recent LLM developments, experts interviewed for this Report acknowledge that the distance to achieving AGI has shortened, due to rapid, transformative improvements in performance. However, there is no clear opinion on timescales, as current LLMs still have limitations that need to be tackled before AGI is realized.
The emergence of AGI could deeply impact businesses both positively and negatively:
- Positive — improving efficiency in processes and decision-making; facilitating innovation
- Negative — reshaping social human relationships; impacting work environments and leading to ethical issues and misaligning human and AI visions
Chapter 7 provides a more in-depth look at AGI and its potential effects and timing.
INTERLUDE #2
The philosopher’s contribution — Will GenAI replace humans?
Luc Ferry is a philosopher, writer, and speaker. Dr. Ferry is former French Minister of Education and di-rected a research center at ENS-Ulm. He was also a professor at the University of Lyon and the University of Caen, France. Dr. Ferry also taught at the DEA level at Sorbonne University (Paris I) and at Sciences Po, Paris. He earned a graduate degree in philosophy and a PhD in political science.
Toward the end of human labor?
Could AI eliminate 300 million jobs, as suggested by Goldman Sachs? Could we consider an end to human labor in the era of AI, as predicted by some analysts like Jeremy Rifkin in his book The End of Work? Advances in GenAI raise these questions. ChatGPT demonstrates a stunning ability to perform various complex tasks, ranging from drafting summaries to conducting precise medical analysis to building architecture, and does so in over 150 languages.
In A World Without Work, Daniel Susskind, a researcher at Oxford University, revisits Rifkin’s thesis and takes this new context into account. Susskind compares the different industrial revolutions: while those of steam, electricity, and oil led to the disappearance of certain manual and repetitive jobs, they also created new ones in other sectors. However, with new connectionist AIs, we are witnessing a major shift: it is not only manual tasks being replaced, but also highly sophisticated intellectual activities, involving professions such as doctors, engineers, and more.
Nevertheless, Susskind’s conclusions about the end of human labor remain uncertain. We may still be in the “creative destruction” model. The World Economic Forum predicts a massive loss of jobs due to AI, but also suggests that technology could create as many, if not more.
It is crucial not to conflate tasks and professions. While many tasks will be impacted or replaced by AI, it does not mean that professions will disappear. For example, although many secretarial tasks are being automated, secretaries will still be essential for more complex and human activities. The same goes for doctors, lawyers, and accountants.
However, Susskind’s warnings must be taken seriously. AI is gaining ground in its competition with humans, and it is urgent to address the challenges that this major technological revolution poses for future education and training.
With LLMs, are we moving toward strong AI?
Three ages of AI are generally identified:
- The symbolic AI of the 1950s pioneers
- The current connectionist AI
- The future representative AI, named JEPA (Joint Embedding Predictive Architecture) by Yann LeCun
According to LeCun, in charge of AI at Meta, LLMs are doomed to failure and will no longer be used five years from now. He suggests working on a new form of AI that works like the human mind, based on “representations.” However, the precise nature of this approach remains uncertain.
Currently, connectionist AI, especially LLMs generating text and images, sparks much discussion. A frequent question is whether AI will surpass human intelligence. In reality, it seems that it is already the case. LLMs like ChatGPT aced the Turing test and scored 155 in an IQ test, placing these machines above 99% of the human population. ChatGPT also solved a physics problem in less than 10 seconds and passed the New York State bar exam in mere seconds. Furthermore, I myself posed several ethical questions to ChatGPT, and the responses provided were superior to those of 90% of the students whose papers I graded. In short, AI is already significantly superior to human intelligence in some cases.
The real question to ask is whether AI will ever become “strong.” We must not confuse a general AI (i.e., AGI) with a strong AI. A strong AI is not just a generalist; it would also have self-awareness, conscious understanding of its statements, and feelings and emotions. It is obvious that a generalist AI superior to humans in almost all areas of pure intelligence and capable of perfectly simulating emotions in a dialogue with a human at some point will see the day. However, it will remain “weak” in that it will have no consciousness or understanding of what it says — no reflection, no feelings, no interests.
Three rational arguments support this observation:
- The “Chinese room,” an argument from American philosopher John Searle, aptly illustrates connectionist AI. Even if it can solve problems, understand natural language, answer questions correctly, and make poetry, it doesn’t understand what it says.
- We humans are guided by our interests, feelings, and emotions, which require a living organism to be truly “felt.” Therefore, if we ever manage to create a strong AI, it could only be possible by creating chimeras, cyborgs, or “animats.”
- Those who believe in the possibility of a strong AI have a materialistic worldview, thinking that we are already machines — living — but machines, nonetheless. Such people believe that consciousness and life emerged from inanimate matter and that we will be able to create artificial neural connections so complex and efficient that they can generate consciousness and emotions, a strong AI anchored in a mechanical brain.
The problem with materialism is that it claims to radically break with the “idols” of religions and classical metaphysics, while it continually falls heavily back into the metaphysical ruts from which it nevertheless had a duty to escape. Materialism, in its various forms, seeks the ultimate material cause of our behaviors and choices, claiming to be determined by unconscious and material causes. However, the search for an ultimate foundation of values is a metaphysical illusion, whether in theology or materialism. Both, ironically, make the same mistake, seeking an ultimate foundation. The transcendence and dualism of consciousness are supposed to be anchored in an exhaustive explanation, whether the foundation is divine or material. However, the idea of an ultimate foundation is both inconceivable and incomprehensible. It is essential to understand that there is no completed science or absolute knowledge. Even the positive sciences acknowledge the relativity of all reasoning. Our experience of transcendent values cannot be denied or materially explained, no more than it can be founded on faith in a divinity.
Strong AI remains and will always be a utopia, which does not prevent research from continuing to dig in the direction of this kind of “asymptotic guide.” GenAIs will not stop progressing to become generalist AIs. In the coming years, they will integrate millions of additional parameters and will be thousands of times superior to the current ones, especially as they will be able to better integrate new data and correct their own mistakes. They will imitate strong AI beautifully — while remaining weak AIs, though generalists.
— Luc Ferry
6
Toward artificial general intelligence
Adoption among end users of GenAI has been extremely fast. For example, we estimate that ChatGPT had close to 300 million unique users in April 2023.
More importantly, adoption and interest has grown rapidly among developers and entrepreneurs, helping build an ongoing, vibrant ecosystem (see Figure 43).
Innovation waves tend to follow a recurring pattern, beginning with the creation of scientific foundations, then developing technology building blocks, and from there to general adoption. GenAI has now reached this last stage, achieving generalized real-world productivity in multiple use cases. This suggests that further breakthroughs are likely to bring new ways in which businesses, societies, and humans operate.
The debate around emergent abilities
There are multiple stages in the development of AI, with experts agreeing that currently we are moving beyond artificial narrow intelligence (ANI) toward full artificial general intelligence (see Figure 44):
“Artificial general intelligence: a theoretical form of AI where a machine would demonstrate intelligence on par with or above humans on a variety of tasks.”
Progress has been swift. Tasks previously deemed unachievable by AI have been successfully completed by very large models. This has prompted discussions of emergent abilities, where models have been observed empirically to gain capabilities that enable them to perform tasks in which they have not been designed. These are typically complex tasks that require nuance or multiple inputs.
This has led to debate around whether such emergent abilities are a hallmark of AGI, or if they are simply due to the metrics selected to measure model performance. Papers produced by both Microsoft and Google argue that emergence is an early version or spark of AGI, although other researchers, including experts from Stanford University, strongly disagree.
The acceleration of progress to AGI
Experts agree that we are closer to reaching AGI than originally estimated, with some believing it will be here within a decade. Around a third of experts we surveyed indicate that recent LLM developments have altered their initial belief about the time frame to achieve AGI. However, to reach AGI, significant obstacles with current models, such as hallucinations and producing new knowledge, need to be overcome. Nevertheless, rapid progress is leading to concerns about the existential “threat” that AGI could pose.
AGI — pessimism & optimism
There is little evidence of the emergence of a Terminator-style AI that is misaligned with human needs and will lead to widespread replacement and/or elimination of people. Sudden drops in the loss function during training tests have mistakenly been equated to AI systems developing potentially uncontrollable capabilities. However, further analysis has subsequently disproven the possibility.
At the same time, there are at least two arguments that lend some support to this pessimistic case for AGI:
- The technology is being developed and broadly adopted at an unprecedented pace.
- A destructive AGI requires minimal materials and could be made more easily than other destructive technologies, compared to traditional weapons of mass destruction (e.g., nuclear bombs).
Looking at some other arguments for pessimism, there are differing views; for example:
- AIs developing their own volition. This is the “HAL 9000” scenario in Stanley Kubrick’s film 2001: A Space Odyssey, in which an AI decides it needs to take control, tries to eliminate its human colleagues, and resists being switched off. However, there is no evidence that AIs can develop their own volition.
- AIs causing physical harm. AIs integrated with safety-critical physical systems, such as today’s autonomous vehicles, will be far more extensive in the future. This gives an AI the potential to cause direct harm to humans. However, optimists would point out that such integration would always be limited by safeguards, both technical and human.
- AIs unable to match human complexity. Some observers consider that AIs will always interpret human goals too narrowly, risking distortions and polarizations. However, optimists would say that AIs can always be prompted to take on increasingly complex tasks provided that suitable learning is introduced.
Still, concerns have prompted the publication of an open letter in March 2023, signed by Sam Altman of OpenAI and Elon Musk of Twitter/X as well as politicians and commentators, calling for a pause in large-scale AI experiments until the possibilities were better understood.
One set of concerns revolve around the impact of AGI on society, particularly in the world of work. GenAI has already led to worries about the elimination of many millions of jobs, and the resulting social and economic repercussions. For example, some recent estimations suggest that around half of all workers could find that more than 50% of their tasks could be performed by AI in the future. Unlike previous industrial revolutions, the impact is likely to fall first on more highly educated roles rather than manual roles that are more difficult to automate.
However, it is important to distinguish between employee roles (job title and responsibilities) and the tasks a person carries out as part of that role. While it is likely that many tasks will be taken over by GenAI, workers will still be required to orchestrate these tasks and will therefore be assisted, not replaced, by AI.
Beyond the impact on the workforce, concerns have been raised around what AI means for the “de-skilling” of humans. For example, if content can be quickly created through GenAI, writing skills will atrophy, in the same way that map reading and having a good sense of direction have been superseded by navigation technology. While this may indeed happen, GenAI will require new skills within the workforce like prompt engineering.
Importantly, if and when AGI is reached, it may provide positive benefits, including:
- Assisting human productivity by enhancing efficiency and accuracy in business and industrial processes through task automation, problem optimization, and assisted decision-making.
- Solving new problems by providing new insights, advanced problem-solving capabilities, enhanced creativity and ideation, and cross-discipline collaboration. GenAI may help us tackle the great challenges of our time, including climate change.
- Increasing human happiness by eliminating the most menial mental tasks from workers’ schedules and refocusing on distinctively human tasks, such as relationship building, moral decision-making, and radical creativity.
Overall, it is important not to ignore the potential benefits of AGI when considering future governance and regulation.
7
The way forward
GenAI will have an impact regardless of industry sector. To reap its benefits, we recommend that companies consider five main steps (see Figure 45). As with any digital transformation project, it is difficult to know in advance the path to success, requiring a focus on test-and-learn techniques that can then be used to consolidate your strategic vision and roadmap for GenAI.
Step 1: Define the problem landscape
Start with an assessment of the key problems you wish to solve. Different problem types are suited to different AI solutions and approaches, based on the openness of the question and the availability of data (see Figure 46, repeated from Chapter 2):
- Semi-open question, lots of data. These are particularly suited to GenAI, including LLMs, which are strong in language understanding, reading comprehension, and question answering.
- Open question, lots of data. Employ semantics, especially knowledge graphs, as these enable you to map out and organize terms, ideas, documents, or other types of information according to their semantic links (i.e., their meaning).
- Specific question, lots of data. Use computer vision to identify patterns/objects in videos and images using CNNs (e.g., video surveillance).
- Open question, little data. Use AI simulation agents to test the impact of a decision, such as how to optimize plant operations or modeling the environmental impacts of a manufacturing plant.
- Specific question, little data. The best approach for these problems is using classical symbolic and rule-based AI systems, or NLP techniques, such as voice recognition.
- Semi-open question, some data. Employ classical science methods, such as ML and data mining; the unsupervised clustering of customer behavior is one possible application.
Step 2: Assess the value of GenAI solutions
The closer a proposed GenAI use case is to a company’s core know-how, the greater the potential benefits, but the requirements and potential costs are higher (see Figure 47). For example:
- Applications that have high stakes in terms of business, human life, and/or environmental damage will require additional measures to identify and mitigate the risks from GenAI’s limitations.
- Applications where knowledge is deeper and specific to the organization will require greater customization of the GenAI model.
- Applications where data confidentiality is highly critical (e.g., personal data and intellectual property) may pose challenges for GenAI data management.
Companies therefore need to balance the potential value against the costs, required resources, and risks of deploying GenAI for specific use cases.
Step 3: Choose an implementation model
The best implementation model (buy, partner, or build) will depend on specific needs and budget. Follow the implementation decision tree in Figure 48 to understand the pros and cons of each approach for particular GenAI use cases.
For a quick-and-easy solution, acquiring a pre-trained model is a good option, enabling you to focus your effort on prompt engineering; if a hybrid solution is required, a vendor partnership provides greater support. If increased control and customization are needed, then building proprietary models is the optimal solution.
Step 4: Try out PoC
A test-and-learn approach is essential to building understanding of GenAI and the specific benefits it brings to your organization. Identifying one or two initial use cases and running PoC projects around them will build experience and capabilities; moving forward, lessons can be integrated into the overall strategy and approach.
Step 5: Consolidate strategy
Once PoCs have been completed, build on your new knowledge to create and consolidate an enterprise AI strategy and roadmap. This should consider AI maturity, critical uncertainties, and the readiness of both the technology and your organization around the pillars of value creation, people, governance, data management, and technology.
Scenario-based strategic planning approaches should be adopted, taking into the account especially the critical short-term uncertainties around regulation, model scalability and value chain development, as well as the longer-term uncertainty around the emergence of AGI. In practice this means having “no regret” strategic actions that are robust to all scenarios, as well as considering alternatives to suit different development trajectories and “strategic insurances” to safeguard against possible negative impacts.
Often a maturity roadmap is a useful initial stage to consider priorities. Figure 49 shows an illustrative example of an AI maturity heatmap, translated into a multi-year AI roadmap.
Conclusion — A new civilization?
So is GenAI synonymous with a new civilization? Given the current level and foreseeable future development of GenAI, concerns that it will completely replace humans at work seem hyperbolic, or at least premature.
At the same time, any impacts on employment should be understood within the context of larger macroeconomic transformations, in which the workforce progressively transfers to new activities. For example, the advent of office automation software did not drive mass unemployment, as typists, designers, and personal assistants upskilled or transferred to adjacent roles. GenAI is therefore not synonymous with a new civilization.
A more optimistic and likely scenario is the development of GenAI “assistants” to support human workers in many of their tasks. This is likely to improve productivity and well-being at work. In that sense, GenAI may be a new civilization of cognitive labor.
Overall, GenAI, especially when orchestrating SoS, is likely to perform a broad range of tasks — beyond the boring, mundane, and repetitive. Some of the most complex and data-intensive challenges humankind faces, first and foremost the response to climate change, may benefit hugely from the advances of GenAI in data collection and analysis, problem solving, and system orchestration.
However, some uniquely human skills are likely to remain out of reach for AI. These include the analysis and influence of human motives, relationship building with humans, and high-stakes decision-making with ethical considerations (i.e., life-or-death decisions). It is likely that these specialized skills will remain the prerogative of human beings and thus become a key principle within organizations and a focal point for education and skills development.
Rather than threaten humans, perhaps counterintuitively GenAI may actually enable us to be more human ourselves and thus help us shape a new — and better — civilization in the future.
Appendix
Glossary of Common GenAI Terms
- Artificial general intelligence (AGI) — a theoretical form of AI where a machine would demonstrate intelligence on par with or above humans on a variety of tasks
- Attention/self-attention — the ability to identify which words in the natural language input sequence (the user’s prompt, or the previous parts of text) are most relevant to predicting the next word
- Auto-regressive — a model that forecasts future behavior based on past behavior data
- Classification — an approach that sorts data points into pre-defined classes
- Clustering — an approach that groups similar data points together to form distinct populations
- Convolutional neural networks (CNN) — a type of deep learning, CNN is a class of artificial neural network most commonly applied to analyze visual imagery
- Deep learning — a subset of machine learning that uses neural network methods modeled on the human brain
- Embeddings — the representations or encodings of tokens, such as sentences, paragraphs, or documents, and the method by which models capture and store the meaning and the relationships of the language
- Emergent abilities/emergence — where models have been observed empirically to gain capabilities that enable them to perform tasks in which they have not been designed
- Feed-forward loop — a type of artificial neural network in which all information flows in a forward manner only
- Fine-tuning — approaches used to improve foundational model performance through additional steps or stages
- Generative AI (GenAI) — a type of AI technology that can produce various types of content, including text, imagery, audio, and synthetic data
- Graphics processing unit (GPU) — a specialized computer chip that provides the compute power for training AI models
- Hallucination — incorrect, yet believable, results provided by LLMs
- Knowledge graph — also known as a semantic network, it represents a network of real-world entities and illustrates the relationship between them
- Large language model (LLM) — a trained DL model that understands and generates text or other outputs in a human-like fashion
- Machine learning — an iterative process of training models to learn from data and make predictions without being programmed explicitly
- Model — a program that analyzes data sets to find patterns and make predictions
- Natural language processing (NLP) — a field of AI that gives machines the ability to process and derive meaning from linguistic inputs (i.e., natural language text and/or speech
- Neural networks — a form of deep learning that uses interconnected nodes or neurons in a layered structure that resembles the human brain
- Parameters — the weighted connections between neurons in a neural network, which can be changed independently by algorithms as they learn
- Parametric memory — AI operations based on a mathematical model that defines the relationship between inputs and outputs
- Predictor functions — a function used to predict a future outcome based on given circumstances
- Probabilistic — a statistical technique used to consider the impact of random events or actions in predicting the potential occurrence of future outcomes
- Recommendation — a type of machine learning that uses data to help predict, narrow down, and find what people are looking for among an exponentially growing number of options
- Regression — an approach that builds a mathematical function that uses input data points to predict a continuous or numerical output for new data points
- Reinforcement learning from human feedback (RLHF) — an AI training method where models are “rewarded” when they learn from human feedback
- Transformers — a neural network that learns context and thus meaning by tracking relationships in sequential data
- Training sets — often very large volumes of data used to train LLMs
Notes
[1] For a more detailed explanation of AI terms, see Appendix.
[2] See the 2023 Blue Shift Report “The Industrial Metaverse — Making the Invisible Visible to Drive Sustainable Growth.”
DOWNLOAD THE FULL REPORT