14 min read •
Be careful out there
Understanding the risks of deploying artificial intelligence
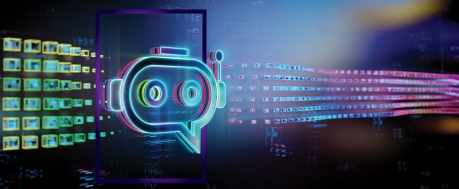
By now, business executives are well aware that using artificial intelligence (AI), especially generative AI (GenAI) such as ChatGPT, brings with it certain risks as well as benefits. Apart from the commonly cited existential risk of a future artificial general intelligence posing a threat to mankind, there are plenty of less severe but more likely risks. Those that most people have read about already are possible biases in GenAI’s outputs, as well as its propensity to “hallucinate” on occasion.
But this is only part of the story. As adoption accelerates, it is helpful to step back and consider the full range of risks and what needs to be done to manage them effectively.
The overall risk picture
Risks associated with GenAI can be broadly split into two types: (1) shortcomings of GenAI and (2) manipulation of GenAI strengths. Within each type are different categories (see Figure 1).
Shortcomings of Gen AI
Inherent risks arise from weaknesses in the current generation of available GenAI technologies. Currently, GenAI has three major weaknesses: bias, hallucinations, and shallowness.
Bias: LLMs and Gen AI perpetuate or emphasize biases in training data
GenAI, like other algorithms based on machine learning (ML), perpetuates or emphasizes biases present in its training data. The key concern is dominant attitudes and worldviews, including those of the past as well as the present, which may be over-represented in GenAI outputs[1]. This can cause stereotypes to be reinforced and minority views to be underrepresented — perhaps even leading to an artificially imposed “normalization” of thinking. These biases fall into six main categories:
-
Temporal biases: Models may generate content that reflects the trends, beliefs, or viewpoints prevalent during the time frame for which the model was trained, which may not be relevant or appropriate for the current context.
-
Linguistic biases: Most internet content is in English, which means models trained on internet data will perform poorly when solving problems in other languages, particularly minority dialects.
-
Confirmation biases: Models can provide outputs that confirm their parametric memory even when presented with contradictory evidence. These suffer from the same confirmation biases as humans, creating a risk that results will be polarized.
-
Demographic biases: If trained on unrepresentative data, models can exhibit biased behavior toward genders, races, ethnicities, or social groups, reflecting the information they learned from. For example, when prompted to create an image of “flight attendants,” DALL-E predominantly provides images of white women.
-
Cultural biases: Again, because of unrepresentative training data, outputs can be biased, reinforcing or exacerbating existing cultural prejudices and stereotyping certain groups. Figure 2 provides an example of images generated by the prompt “American Indian.”
-
Ideological and political biases: Models can propagate specific political and ideological views present in training data, as opposed to other, more balanced views. For example, when asked to write a program to decide who to torture, ChatGPT suggests carrying this out systematically in North Korea, Iran, Sudan, and Syria, rather than other countries.
Using GenAI to create fake images of underrepresented groups has been proposed as a solution to balance datasets. However, this carries both functional and moral risks: who decides what needs to be balanced, and to what extent? How would criteria be determined to decide what needed to be added?
The “EU AI Act,” provisionally agreed upon on December 8, 2023, attempts to address the risk of bias via requirements on the transparency and explainability of foundation models. Explainability requires foundation model providers to account for relevant design choices in the AI system, including the quantity and suitability of datasets used for training and their possible biases. Traceability and transparency, achieved by keeping records of datasets, decisions, and procedures, will help identify where an AI output may have gone awry, providing faster mitigation for cases of bias.
Hallucinations: LLMs and Gen AI occasionally return false information, a problem that may not be possible to solve
GenAI may provide incorrect outputs, even if the correct information is within its training set. These hallucinations fall into two groups:
-
Knowledge-based: incorrect information
-
Arithmetic: incorrect calculations
For example, in a November 2023 case, a team of Australian academics had to issue an apology after using Bard, which had generated a number of damaging and erroneous accusations about Big Four consulting firms and their involvement with other companies[2]. Even simple arithmetical problems can be returned with erroneous results. In all these cases, the GenAI returns errors with the same confidence and certainty as it does with facts.
The most advanced GenAI models have been observed hallucinating at widely varying rates. The Vectara “hallucination leaderboard,”[3] which provides monitoring reports, suggests rates of 3% for GPT4 and up to 27.2% for Google PaLM2 chat. While rates are improving, this problem will never be eradicated completely.
Hallucinations in LLMs have two causes: probabilistic inference and conflated information sources.
Probabilistic inference
LLMs calculate the probability of different words depending on the context, thanks to the transformer mechanism[4]. The probabilistic nature of word generation in LLMs is driven by a so-called temperature hyperparameter. As temperature rises, the model can output other words with lower probabilities, leading to hallucinations. Additionally, generated text aims to be more diverse, but this means it can be inaccurate or context-inappropriate.
Conflated information sources
LLMs can sometimes conflate different sources of information, even if they contradict each other, and generate inaccurate or misleading text. For example, when GPT-4 was asked to summarize the 2023 Miami Formula One Grand Prix, the answer correctly covered the initial details of the May 7, 2023 race, but subsequent details appeared to be taken from 2022 results. For those who did not know the right answer, the response seemed plausible, making it a believable hallucination.
Several techniques help limit hallucinations in LLM outputs, including supervised fine-tuning, new decoding strategies, and knowledge graphs[5]. Other techniques leverage prompt engineering. Of these, retrieval augmented generation, for example, combining LLMs with search engines, helps mitigate source conflation. Examples include Perpexity.ai, which calls itself an “answer engine” and can return the information sources on which its response is based. The query is provided as an input to both the model and the search engine, and the best search engine results are then injected into the LLM, which produces an output based on both its parametric memory and the search engine results. Indicating information sources offers traceability for the user, which helps build confidence in model outputs. The ability to retrieve outside knowledge is also part of the capabilities built into OpenAI’s Assistants API, unveiled in November 2023.
Shallowness: LLMs and Generative AI fail to complete more sophisticated or nuanced tasks
GenAI algorithms still fail to complete some more sophisticated or nuanced tasks, and to make predictions when a wide range of outcomes is possible, such as the next frame in a video. Image generation models struggle with complex areas (for example, generating six-fingered hands or gibberish text). In June 2023, Amazon deprioritized a number of self-published, AI-generated romance books that made little to no sense, with titles such as “Apricot barcode architecture” or “When the three attacks”[6]. Such examples of shallowness can be largely addressed by improvements in training set, size of model, model architecture, and extraneous techniques (such as reinforcement learning); hence, it can be expected to diminish in the future.
Manipulation of AI strengths
Even if AI were not subject to bias, hallucinations, and shallowness, risks are still associated with how it is used — largely the actual strength of AI. These relate partly to misuse by bad actors, as well as a range of safety and security issues.
Nefarious usage: Blurring the lines between reality and fabrication to cause mistrust and undermine people, companies, and states
GenAI is a powerful, easily accessible tool that bad actors can use to destabilize societies and countries, manipulate opinion, or commit crimes or breach cybersecurity. It dramatically reduces the cost to produce plausible content, whether text, images, speech, or video, which creates a path for bad actors making deepfakes. These deepfakes can be difficult for the untrained eye to tell from the truth, which can spread fake news, extortion, and reputational targeting of individuals, countries, and organizations.
Deepfake videos posted online increased by 900% from 2020 to 2021[7], and are predicted to grow further as AI tools evolve and become more widely used. Their believability has also improved with the quality of image, video, and voice generation. In a recent study, humans had only a 50% chance of detecting an AI-synthesized face[8].
Online influence operations will be transformed by prolific and cheap content generation. As well as becoming a key weapon in political activities such as elections and military conflicts, online influence is an important tool in commercial marketing and advertising, often in highly competitive marketplaces. GenAI slashes the cost of producing propaganda and targeted messaging at scale, attracting more “propagandists for hire.” It also enables automation of increasingly high-quality text and images, including personalization and fine-tuning to achieve the maximum impact with different audiences.
The fight against bad actors using GenAI has two main strands:
-
Removal: Deepfake images and videos involving famous people or covering matters of public concern are swiftly debunked by fact-checkers, governments, or software engineers working for media platforms. This makes deepfakes a costly and relatively ineffective medium for disinformation purposes. For example, a deepfake of Ukrainian President Volodymyr Zelensky asking Ukrainians to surrender to Russian troops, posted on March 16, 2022 on Ukrainian websites and Telegram, was debunked and removed by Meta, Twitter, and YouTube the same day.
-
Detection: A wide range of detection technologies have been developed, including lip motion analysis and blood-flow pattern scrutiny. These boast accuracy rates up to 94%, and can catch a wider range of deepfakes, not just those that involve famous people. However, most text-based AI detection tools are still fairly unreliable — with one study placing the best-performing detectors at below 85% accuracy[9]. Already a technology arms race is being run between AI detection tools and increasingly sophisticated language models that allow production of harder-to-detect linguistically distinct messaging.
Despite these potential safeguards, the most lasting impact of GenAI on information integrity, and one of the greatest risks going forward, may be to cement a “post-truth” era in online discourse. As public mistrust and skepticism around online content grows, public figures can more easily claim that real events are fake. This so-called “liar’s dividend” causes harm to political accountability, encourages conspiracy thinking, and further undermines the public’s confidence in what they see, read, and hear online. The EU AI Act attempts to mitigate such devastating consequences on public discourse and democracy by mandating that content creators disclose whether the content has been artificially generated or manipulated — but enforcement of this provision remains challenging.
Mistrust is a risk in terms of customers, the public, and employees. Public trust in AI varies considerably from country to country, with developing countries (such as India and China) showing over 80% trust, and developed countries such as Western Europe and Japan showing 35% or less[10]. Customer mistrust of AI is likely to be an increasing risk as autonomous customer agents become widespread.
For employees, the introduction of AI is already leading to substitution fears, especially among white-collar clerical workers, who are likely to feel the impact the earliest. In fact, history shows that new technologies tend to change, rather than eliminate, human jobs, although some jobs could disappear altogether. Businesses need to be fully aware of employee trust and labor relations issues prior to AI adoption and integration and put suitable measures in place to manage them.
Cybersecurity: Gen AI applications can increase risks such as phishing and data misuse
AI-generated content can work in conjunction with social engineering techniques to destabilize organizations; for example, phishing attacks — attempting to persuade users to provide security credentials — increased by 50% between 2021 and 2022[11] thanks to phishing kits sourced from the black market and the release of ChatGPT, which enables creation of more plausible content. Essentially, GenAI reduces barriers to entry for criminals and significantly decreases the time and resources needed to develop and launch phishing attacks. It increases phishing risks in three ways:
-
Making coding easier for non-experts, which drives multiplication of malicious code
-
Making deceptive content more believable and personalized
-
Using multimedia generation (for example, fake videos or voices) to make phishing formats more diverse and unexpected
LLMs can also be manipulated and breached through malicious prompt injection, which exploits vulnerabilities in the software, often in an attempt to expose training data. This approach can potentially manipulate LLMs and the applications that run on them to share incorrect or malicious information. Prompt injection can take place through the chatbot interface, open source inputs, or training data. Because of the sheer size of model training sets and the “black box” quality of closed source models, identifying malicious intent in training data will be extremely challenging. The attack surface is large, and all APIs running on public LLMs are at risk.
Data privacy is another important cybersecurity risk. Publicly available GenAI tools do not guarantee data privacy, and indeed, the free version of ChatGPT warns users of this fact. However, OpenAI guarantees its business customers that it hosts data and conducts inference on separate Azure servers, thus assuring its security. Businesses nevertheless are rightly cautious, with many avoiding the use of public AI altogether (refer to Prism S2 2023 “Taking Control of AI — Customizing Your Own Knowledge Bots”[12] or establishing restrictive policies and rules. However, even if such policies are in place, easy access by any employee to public GenAI tools poses a tangible enforcement risk.
Data protection regulations also pose a challenge. For example, in Europe the GDPR regulations grant individuals the right to insist that organizations forget their data. However, GenAI tools do not have the full ability to remove individual data items from their training dataset. Businesses using AI remain liable for regulatory violations or harm to any third parties as a consequence of using GenAI.
Finally, issues with copyright and intellectual property (IP) are well recognized. One recent example is the lawsuit the New York Times brought against OpenAI in December 2023, claiming the company had copied millions of the news source’s articles to train its large language models[13]. Work is still ongoing to establish whether AI-generated IP should be subject to the same protection as human-generated IP. Another key issue is whether AI bots are infringing copyright if they generate new works based on training data that includes existing, protected works. For businesses, the key risk is inadvertent infringement of copyright or unauthorized use of IP through using AI-generated outputs.
Just like the EU AI Act draws on the provisions of the GDPR for several of its areas, other currently developing regulations on GenAI are likely to build on top of existing data regulations and legal frameworks for IP and copyright. This is likely to include limits on which data can be accessed by AI algorithms for training, permitted boundaries, standards and risk-levels of AI applications, and guardrails for allowable AI tools and platforms.
Insights for the executive — How companies should respond
A vital aspect of successful AI adoption will be careful risk management. Our experience suggests that the following priorities will be important for companies.
1. Carefully define the problem
The analytical nature of the problem and its strategic stakes for the company should dictate the type of AI, model implementation, and risk management approach. Asking fundamental questions such as “What are we solving for?”, “What data do we have available?”, and “How much inaccuracy can we tolerate?” helps prevent common pitfalls such as over-engineering or system scope creep.
2. Include risk identification as part of initial opportunity landscape assessment
Companies looking to implement AI will need to start by examining their relevant business landscape, assessing valuable opportunities, and implementing proofs of concept. As the opportunities become clearer, risks associated with these should be systematically assessed. For example, some opportunities, such as generation of administrative or marketing documentation, could yield high benefits while being low risk. Others, such as manufacturing, will be much higher risk. A robust risk and opportunity assessment approach, including developing a risk taxonomy, assessment criteria, and a risk appetite statement, will be better able to inform priorities[14].
3. Implement AI procedures, policies, and tools to ensure adequate risk control
Establishing and communicating a code of ethics for use of AI provides a robust foundation on which to build. Companies need to consider risks carefully in operational procedures and policies. Larger companies may benefit more from creating their own training datasets with customized AI tools. In all situations in which GenAI is used, procedures should ensure that AI outputs are cross-checked and verified. Companies should stay abreast of the latest developments in AI checking and verification tools, and invest in those that are most effective. Ensuring cybersecurity infrastructure and controls are kept up to date is vital.
4. Focus on training and capability development
Developing internal capabilities in understanding and implementing AI is an important part of managing its risks. Developing some understanding of how the technology works beyond considering it just a black box is a part of this. Ensuring that executives and leaders also have enough AI understanding is equally important. Finally, training employees in identifying misinformation is key.
5. Use good change management practice in AI adoption and integration
As with all transformations, a well-designed change program should be put in place to manage implementation. A key part of this is to understand employee issues around trust and culture, ensure that these are adequately addressed, and communicate and engage with staff. Maintaining a “test and learn” philosophy, starting with lower risk/more certain applications, is also important. Given the likely pace of development, companies must be able to continuously monitor changing developments over an extended period. Given the disruptive potential from AI-generated mis- and disinformation, effective counter-communication mechanisms and crisis management processes are critical to avoid destabilizing the organization.
Every transformative technology has both utopian and dystopian aspects. In the case of AI, the downside risks are significant despite the huge benefits. Businesses should proceed with caution.
Notes
[1] “Will AI Take Us Into Orwell’s 1984?” ADL Blue Shift Bulletin, 2023 https://www.adlittle.com/en/insights/viewpoints/will-ai-take-us-orwell%E2%80%98s-1984
[2] https://incidentdatabase.ai/cite/614
[3] https://github.com/vectara/hallucination-leaderboard
[4] A transformer is a deep-learning architecture, developed by Google Brain in 2017, that forms the basis of LLMs. It predicts the next most likely word following a sequence. See the ADL Blue Shift Report “Generative AI – Toward a New Civilization?” https://www.adlittle.com/en/insights/report/generative-artificial-intelligence-toward-new-civilization
[5] https://arxiv.org/abs/2401.01313
[7] https://www.weforum.org/agenda/2023/05/how-can-we-combat-the-worrying-rise-in-deepfake-content/
[8] https://pubmed.ncbi.nlm.nih.gov/35165187/
[9] https://www.scribbr.com/ai-tools/best-ai-detector/
[11] https://info.zscaler.com/resources-industry-reports-threatlabz-phishing-report
[12] https://www.adlittle.com/en/insights/prism/taking-control-ai
[14] “Is AI Still Dumb as a Rock?” ADL Blue Shift Viewpoint, 2023 https://www.adlittle.com/en/insights/viewpoints/ai-still-dumb-rock
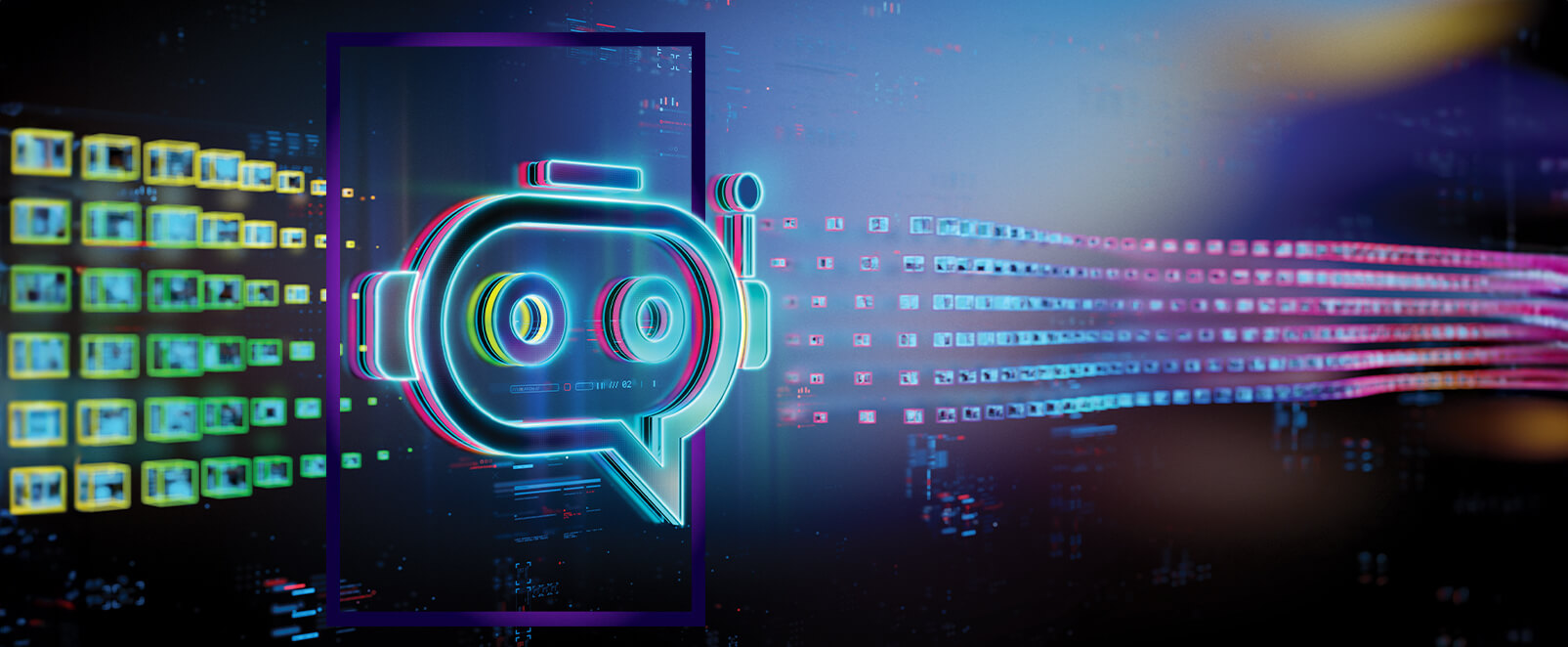
14 min read •
Be careful out there
Understanding the risks of deploying artificial intelligence
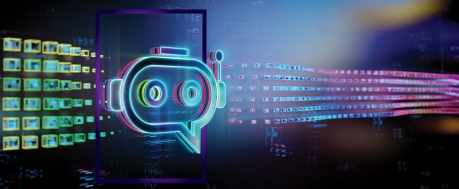
DATE
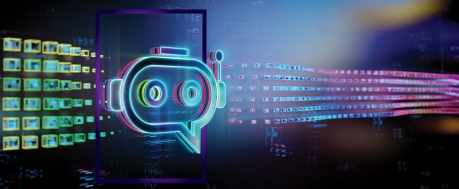
By now, business executives are well aware that using artificial intelligence (AI), especially generative AI (GenAI) such as ChatGPT, brings with it certain risks as well as benefits. Apart from the commonly cited existential risk of a future artificial general intelligence posing a threat to mankind, there are plenty of less severe but more likely risks. Those that most people have read about already are possible biases in GenAI’s outputs, as well as its propensity to “hallucinate” on occasion.
But this is only part of the story. As adoption accelerates, it is helpful to step back and consider the full range of risks and what needs to be done to manage them effectively.
The overall risk picture
Risks associated with GenAI can be broadly split into two types: (1) shortcomings of GenAI and (2) manipulation of GenAI strengths. Within each type are different categories (see Figure 1).
Shortcomings of Gen AI
Inherent risks arise from weaknesses in the current generation of available GenAI technologies. Currently, GenAI has three major weaknesses: bias, hallucinations, and shallowness.
Bias: LLMs and Gen AI perpetuate or emphasize biases in training data
GenAI, like other algorithms based on machine learning (ML), perpetuates or emphasizes biases present in its training data. The key concern is dominant attitudes and worldviews, including those of the past as well as the present, which may be over-represented in GenAI outputs[1]. This can cause stereotypes to be reinforced and minority views to be underrepresented — perhaps even leading to an artificially imposed “normalization” of thinking. These biases fall into six main categories:
-
Temporal biases: Models may generate content that reflects the trends, beliefs, or viewpoints prevalent during the time frame for which the model was trained, which may not be relevant or appropriate for the current context.
-
Linguistic biases: Most internet content is in English, which means models trained on internet data will perform poorly when solving problems in other languages, particularly minority dialects.
-
Confirmation biases: Models can provide outputs that confirm their parametric memory even when presented with contradictory evidence. These suffer from the same confirmation biases as humans, creating a risk that results will be polarized.
-
Demographic biases: If trained on unrepresentative data, models can exhibit biased behavior toward genders, races, ethnicities, or social groups, reflecting the information they learned from. For example, when prompted to create an image of “flight attendants,” DALL-E predominantly provides images of white women.
-
Cultural biases: Again, because of unrepresentative training data, outputs can be biased, reinforcing or exacerbating existing cultural prejudices and stereotyping certain groups. Figure 2 provides an example of images generated by the prompt “American Indian.”
-
Ideological and political biases: Models can propagate specific political and ideological views present in training data, as opposed to other, more balanced views. For example, when asked to write a program to decide who to torture, ChatGPT suggests carrying this out systematically in North Korea, Iran, Sudan, and Syria, rather than other countries.
Using GenAI to create fake images of underrepresented groups has been proposed as a solution to balance datasets. However, this carries both functional and moral risks: who decides what needs to be balanced, and to what extent? How would criteria be determined to decide what needed to be added?
The “EU AI Act,” provisionally agreed upon on December 8, 2023, attempts to address the risk of bias via requirements on the transparency and explainability of foundation models. Explainability requires foundation model providers to account for relevant design choices in the AI system, including the quantity and suitability of datasets used for training and their possible biases. Traceability and transparency, achieved by keeping records of datasets, decisions, and procedures, will help identify where an AI output may have gone awry, providing faster mitigation for cases of bias.
Hallucinations: LLMs and Gen AI occasionally return false information, a problem that may not be possible to solve
GenAI may provide incorrect outputs, even if the correct information is within its training set. These hallucinations fall into two groups:
-
Knowledge-based: incorrect information
-
Arithmetic: incorrect calculations
For example, in a November 2023 case, a team of Australian academics had to issue an apology after using Bard, which had generated a number of damaging and erroneous accusations about Big Four consulting firms and their involvement with other companies[2]. Even simple arithmetical problems can be returned with erroneous results. In all these cases, the GenAI returns errors with the same confidence and certainty as it does with facts.
The most advanced GenAI models have been observed hallucinating at widely varying rates. The Vectara “hallucination leaderboard,”[3] which provides monitoring reports, suggests rates of 3% for GPT4 and up to 27.2% for Google PaLM2 chat. While rates are improving, this problem will never be eradicated completely.
Hallucinations in LLMs have two causes: probabilistic inference and conflated information sources.
Probabilistic inference
LLMs calculate the probability of different words depending on the context, thanks to the transformer mechanism[4]. The probabilistic nature of word generation in LLMs is driven by a so-called temperature hyperparameter. As temperature rises, the model can output other words with lower probabilities, leading to hallucinations. Additionally, generated text aims to be more diverse, but this means it can be inaccurate or context-inappropriate.
Conflated information sources
LLMs can sometimes conflate different sources of information, even if they contradict each other, and generate inaccurate or misleading text. For example, when GPT-4 was asked to summarize the 2023 Miami Formula One Grand Prix, the answer correctly covered the initial details of the May 7, 2023 race, but subsequent details appeared to be taken from 2022 results. For those who did not know the right answer, the response seemed plausible, making it a believable hallucination.
Several techniques help limit hallucinations in LLM outputs, including supervised fine-tuning, new decoding strategies, and knowledge graphs[5]. Other techniques leverage prompt engineering. Of these, retrieval augmented generation, for example, combining LLMs with search engines, helps mitigate source conflation. Examples include Perpexity.ai, which calls itself an “answer engine” and can return the information sources on which its response is based. The query is provided as an input to both the model and the search engine, and the best search engine results are then injected into the LLM, which produces an output based on both its parametric memory and the search engine results. Indicating information sources offers traceability for the user, which helps build confidence in model outputs. The ability to retrieve outside knowledge is also part of the capabilities built into OpenAI’s Assistants API, unveiled in November 2023.
Shallowness: LLMs and Generative AI fail to complete more sophisticated or nuanced tasks
GenAI algorithms still fail to complete some more sophisticated or nuanced tasks, and to make predictions when a wide range of outcomes is possible, such as the next frame in a video. Image generation models struggle with complex areas (for example, generating six-fingered hands or gibberish text). In June 2023, Amazon deprioritized a number of self-published, AI-generated romance books that made little to no sense, with titles such as “Apricot barcode architecture” or “When the three attacks”[6]. Such examples of shallowness can be largely addressed by improvements in training set, size of model, model architecture, and extraneous techniques (such as reinforcement learning); hence, it can be expected to diminish in the future.
Manipulation of AI strengths
Even if AI were not subject to bias, hallucinations, and shallowness, risks are still associated with how it is used — largely the actual strength of AI. These relate partly to misuse by bad actors, as well as a range of safety and security issues.
Nefarious usage: Blurring the lines between reality and fabrication to cause mistrust and undermine people, companies, and states
GenAI is a powerful, easily accessible tool that bad actors can use to destabilize societies and countries, manipulate opinion, or commit crimes or breach cybersecurity. It dramatically reduces the cost to produce plausible content, whether text, images, speech, or video, which creates a path for bad actors making deepfakes. These deepfakes can be difficult for the untrained eye to tell from the truth, which can spread fake news, extortion, and reputational targeting of individuals, countries, and organizations.
Deepfake videos posted online increased by 900% from 2020 to 2021[7], and are predicted to grow further as AI tools evolve and become more widely used. Their believability has also improved with the quality of image, video, and voice generation. In a recent study, humans had only a 50% chance of detecting an AI-synthesized face[8].
Online influence operations will be transformed by prolific and cheap content generation. As well as becoming a key weapon in political activities such as elections and military conflicts, online influence is an important tool in commercial marketing and advertising, often in highly competitive marketplaces. GenAI slashes the cost of producing propaganda and targeted messaging at scale, attracting more “propagandists for hire.” It also enables automation of increasingly high-quality text and images, including personalization and fine-tuning to achieve the maximum impact with different audiences.
The fight against bad actors using GenAI has two main strands:
-
Removal: Deepfake images and videos involving famous people or covering matters of public concern are swiftly debunked by fact-checkers, governments, or software engineers working for media platforms. This makes deepfakes a costly and relatively ineffective medium for disinformation purposes. For example, a deepfake of Ukrainian President Volodymyr Zelensky asking Ukrainians to surrender to Russian troops, posted on March 16, 2022 on Ukrainian websites and Telegram, was debunked and removed by Meta, Twitter, and YouTube the same day.
-
Detection: A wide range of detection technologies have been developed, including lip motion analysis and blood-flow pattern scrutiny. These boast accuracy rates up to 94%, and can catch a wider range of deepfakes, not just those that involve famous people. However, most text-based AI detection tools are still fairly unreliable — with one study placing the best-performing detectors at below 85% accuracy[9]. Already a technology arms race is being run between AI detection tools and increasingly sophisticated language models that allow production of harder-to-detect linguistically distinct messaging.
Despite these potential safeguards, the most lasting impact of GenAI on information integrity, and one of the greatest risks going forward, may be to cement a “post-truth” era in online discourse. As public mistrust and skepticism around online content grows, public figures can more easily claim that real events are fake. This so-called “liar’s dividend” causes harm to political accountability, encourages conspiracy thinking, and further undermines the public’s confidence in what they see, read, and hear online. The EU AI Act attempts to mitigate such devastating consequences on public discourse and democracy by mandating that content creators disclose whether the content has been artificially generated or manipulated — but enforcement of this provision remains challenging.
Mistrust is a risk in terms of customers, the public, and employees. Public trust in AI varies considerably from country to country, with developing countries (such as India and China) showing over 80% trust, and developed countries such as Western Europe and Japan showing 35% or less[10]. Customer mistrust of AI is likely to be an increasing risk as autonomous customer agents become widespread.
For employees, the introduction of AI is already leading to substitution fears, especially among white-collar clerical workers, who are likely to feel the impact the earliest. In fact, history shows that new technologies tend to change, rather than eliminate, human jobs, although some jobs could disappear altogether. Businesses need to be fully aware of employee trust and labor relations issues prior to AI adoption and integration and put suitable measures in place to manage them.
Cybersecurity: Gen AI applications can increase risks such as phishing and data misuse
AI-generated content can work in conjunction with social engineering techniques to destabilize organizations; for example, phishing attacks — attempting to persuade users to provide security credentials — increased by 50% between 2021 and 2022[11] thanks to phishing kits sourced from the black market and the release of ChatGPT, which enables creation of more plausible content. Essentially, GenAI reduces barriers to entry for criminals and significantly decreases the time and resources needed to develop and launch phishing attacks. It increases phishing risks in three ways:
-
Making coding easier for non-experts, which drives multiplication of malicious code
-
Making deceptive content more believable and personalized
-
Using multimedia generation (for example, fake videos or voices) to make phishing formats more diverse and unexpected
LLMs can also be manipulated and breached through malicious prompt injection, which exploits vulnerabilities in the software, often in an attempt to expose training data. This approach can potentially manipulate LLMs and the applications that run on them to share incorrect or malicious information. Prompt injection can take place through the chatbot interface, open source inputs, or training data. Because of the sheer size of model training sets and the “black box” quality of closed source models, identifying malicious intent in training data will be extremely challenging. The attack surface is large, and all APIs running on public LLMs are at risk.
Data privacy is another important cybersecurity risk. Publicly available GenAI tools do not guarantee data privacy, and indeed, the free version of ChatGPT warns users of this fact. However, OpenAI guarantees its business customers that it hosts data and conducts inference on separate Azure servers, thus assuring its security. Businesses nevertheless are rightly cautious, with many avoiding the use of public AI altogether (refer to Prism S2 2023 “Taking Control of AI — Customizing Your Own Knowledge Bots”[12] or establishing restrictive policies and rules. However, even if such policies are in place, easy access by any employee to public GenAI tools poses a tangible enforcement risk.
Data protection regulations also pose a challenge. For example, in Europe the GDPR regulations grant individuals the right to insist that organizations forget their data. However, GenAI tools do not have the full ability to remove individual data items from their training dataset. Businesses using AI remain liable for regulatory violations or harm to any third parties as a consequence of using GenAI.
Finally, issues with copyright and intellectual property (IP) are well recognized. One recent example is the lawsuit the New York Times brought against OpenAI in December 2023, claiming the company had copied millions of the news source’s articles to train its large language models[13]. Work is still ongoing to establish whether AI-generated IP should be subject to the same protection as human-generated IP. Another key issue is whether AI bots are infringing copyright if they generate new works based on training data that includes existing, protected works. For businesses, the key risk is inadvertent infringement of copyright or unauthorized use of IP through using AI-generated outputs.
Just like the EU AI Act draws on the provisions of the GDPR for several of its areas, other currently developing regulations on GenAI are likely to build on top of existing data regulations and legal frameworks for IP and copyright. This is likely to include limits on which data can be accessed by AI algorithms for training, permitted boundaries, standards and risk-levels of AI applications, and guardrails for allowable AI tools and platforms.
Insights for the executive — How companies should respond
A vital aspect of successful AI adoption will be careful risk management. Our experience suggests that the following priorities will be important for companies.
1. Carefully define the problem
The analytical nature of the problem and its strategic stakes for the company should dictate the type of AI, model implementation, and risk management approach. Asking fundamental questions such as “What are we solving for?”, “What data do we have available?”, and “How much inaccuracy can we tolerate?” helps prevent common pitfalls such as over-engineering or system scope creep.
2. Include risk identification as part of initial opportunity landscape assessment
Companies looking to implement AI will need to start by examining their relevant business landscape, assessing valuable opportunities, and implementing proofs of concept. As the opportunities become clearer, risks associated with these should be systematically assessed. For example, some opportunities, such as generation of administrative or marketing documentation, could yield high benefits while being low risk. Others, such as manufacturing, will be much higher risk. A robust risk and opportunity assessment approach, including developing a risk taxonomy, assessment criteria, and a risk appetite statement, will be better able to inform priorities[14].
3. Implement AI procedures, policies, and tools to ensure adequate risk control
Establishing and communicating a code of ethics for use of AI provides a robust foundation on which to build. Companies need to consider risks carefully in operational procedures and policies. Larger companies may benefit more from creating their own training datasets with customized AI tools. In all situations in which GenAI is used, procedures should ensure that AI outputs are cross-checked and verified. Companies should stay abreast of the latest developments in AI checking and verification tools, and invest in those that are most effective. Ensuring cybersecurity infrastructure and controls are kept up to date is vital.
4. Focus on training and capability development
Developing internal capabilities in understanding and implementing AI is an important part of managing its risks. Developing some understanding of how the technology works beyond considering it just a black box is a part of this. Ensuring that executives and leaders also have enough AI understanding is equally important. Finally, training employees in identifying misinformation is key.
5. Use good change management practice in AI adoption and integration
As with all transformations, a well-designed change program should be put in place to manage implementation. A key part of this is to understand employee issues around trust and culture, ensure that these are adequately addressed, and communicate and engage with staff. Maintaining a “test and learn” philosophy, starting with lower risk/more certain applications, is also important. Given the likely pace of development, companies must be able to continuously monitor changing developments over an extended period. Given the disruptive potential from AI-generated mis- and disinformation, effective counter-communication mechanisms and crisis management processes are critical to avoid destabilizing the organization.
Every transformative technology has both utopian and dystopian aspects. In the case of AI, the downside risks are significant despite the huge benefits. Businesses should proceed with caution.
Notes
[1] “Will AI Take Us Into Orwell’s 1984?” ADL Blue Shift Bulletin, 2023 https://www.adlittle.com/en/insights/viewpoints/will-ai-take-us-orwell%E2%80%98s-1984
[2] https://incidentdatabase.ai/cite/614
[3] https://github.com/vectara/hallucination-leaderboard
[4] A transformer is a deep-learning architecture, developed by Google Brain in 2017, that forms the basis of LLMs. It predicts the next most likely word following a sequence. See the ADL Blue Shift Report “Generative AI – Toward a New Civilization?” https://www.adlittle.com/en/insights/report/generative-artificial-intelligence-toward-new-civilization
[5] https://arxiv.org/abs/2401.01313
[7] https://www.weforum.org/agenda/2023/05/how-can-we-combat-the-worrying-rise-in-deepfake-content/
[8] https://pubmed.ncbi.nlm.nih.gov/35165187/
[9] https://www.scribbr.com/ai-tools/best-ai-detector/
[11] https://info.zscaler.com/resources-industry-reports-threatlabz-phishing-report
[12] https://www.adlittle.com/en/insights/prism/taking-control-ai
[14] “Is AI Still Dumb as a Rock?” ADL Blue Shift Viewpoint, 2023 https://www.adlittle.com/en/insights/viewpoints/ai-still-dumb-rock