69 min read
Unleashing the business potential of quantum computing
"If quantum mechanics hasn’t profoundly shocked you — you haven’t understood it yet." — Niels Bohr
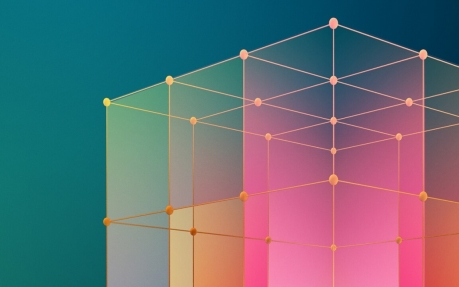
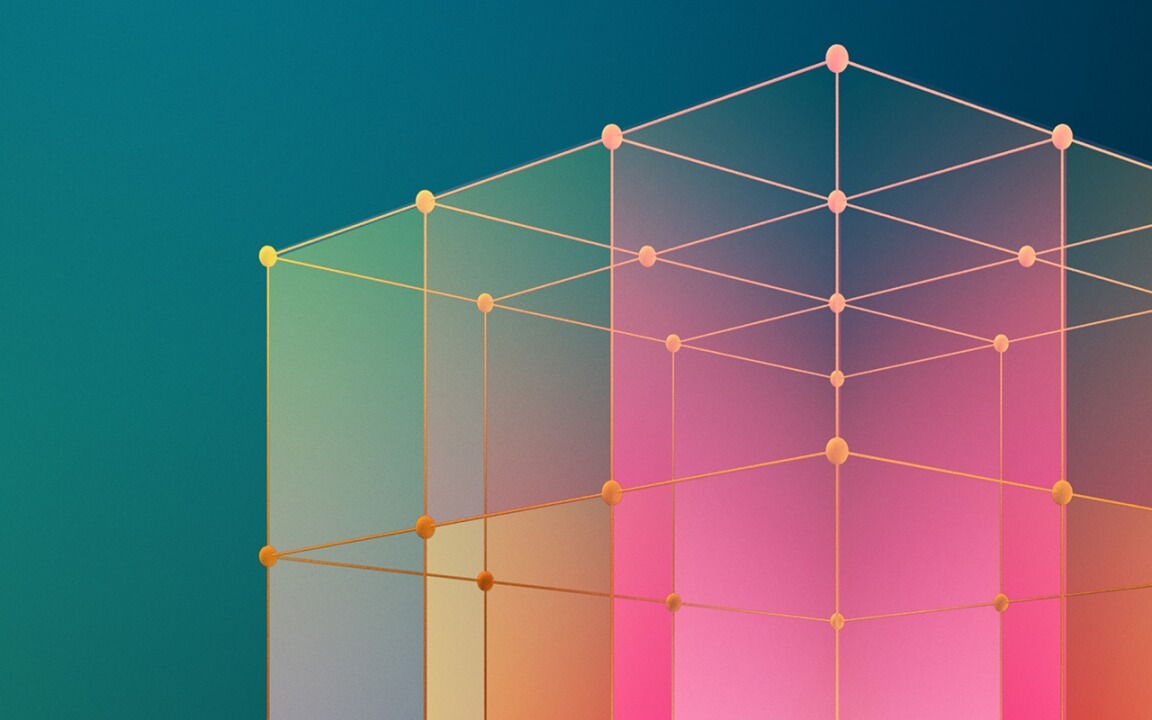
Executive Summary
Most businesses are already aware of the potentially disruptive impact that quantum computing (QC) will have if it develops successfully and becomes commercially available. It promises to transform business through its ability to tackle so-called intractable problems with exponentially increasing complexity that currently are beyond the reach of conventional high-performance computers. These types of problems can be grouped broadly into four categories: simulation, optimization, machine learning (ML), and cryptography. The use cases for business across the first three categories are almost endless, from fully digitalized drug discovery and new materials development through to logistics, supply chain management, and portfolio optimization.
Yet QC technology is extremely complex and hard for even the smartest generalist to properly understand. There is a constant stream of media stories and announcements about new developments and claimed breakthroughs. Seeing clearly through the “fog” is difficult.
In this Report from Blue Shift by Arthur D. Little (ADL), we have aimed to provide a clear overview of the state of play for business readers not familiar with the domain, sharing not just potential use cases and applications, but also technology development, including hardware and software, and highlighting key players active in the QC ecosystem. We also give our perspective on the all-important question of the likelihood and possible timescales for commercialization of QC.
Today, QC has not yet progressed beyond lab scale, even though it is integrated in some commercial offerings. There are still huge technical and engineering challenges to overcome. A fault-tolerant, large-scale, general-purpose device able to provide both technical and commercial advantage over conventional high-performance computers is still many years away, and there is still no certainty that it will be achieved. If and when quantum processing power becomes available, it will be a complement to conventional computing; it will not replace it.
However, recently we have seen a rapid acceleration of investment and activity in QC development, which will certainly continue over the next few years. Declared public investment is in excess of US $25 billion, with over 50 venture capital deals in 2021. There are more than 20 new prototype devices planned in published vendor roadmaps between now and 2030. We expect that there will be some progress in achieving so-called quantum advantage in specific limited applications during the coming years, possibly involving quantum simulators and/or hybrid solutions involving a combination of conventional and quantum processing. There could be an early breakthrough, but of course this is impossible to forecast with any certainty.
Given the current state of play, we recommend that companies that have not already done so take steps now to ensure they are not left behind. The QC learning slope is steep. It will not be possible, especially in the earlier stages of commercialization, for inexperienced users simply to do nothing until the technology matures, buy access to quantum processing power, and immediately expect to make transformational changes in their business operations. Furthermore, as the technology starts to mature, the current open exchange of intelligence and data across the global QC ecosystem may stop, driven by both commercial and geopolitical pressures. Companies that have no internal capability could be especially disadvantaged.
In this Report, we set out four steps companies should take to prepare for the future, including exploring applicability, monitoring technical developments, engaging with the ecosystem, and building knowledge and capability. It is only through taking such steps that companies will be able to see clearly through the fog and avoid being left behind when QC properly takes off.
Preface — The second quantum revolution, from concepts to technologies
There are different kinds of prefaces. Mine is from someone who is not an expert on the major aim of this Report: describing the business perspectives in quantum computing. I am an academic who has all his life worked on basic research, and my expertise in business perspective is negligible. But I am thrilled by the perspective that the purely fundamental research I did long ago on entanglement is now at the core of the second quantum revolution, including its technological sector. Therefore, I will not comment here about statements you will find within the Report. Rather, I would like to share some of my thoughts about the second quantum revolution and a few of my expectations, which stem more from intuition than cold business analysis. For those who already have some understanding of quantum computing technologies, I have provided more background and detail on my observations in the Appendix of this Report.
The first quantum revolution, whose formalism was developed in the first decades of the 20th century, was based on the concept of wave-particle duality. While the concept was expressed in relation to individual quantum particles, it was in fact applied to large ensembles of particles, such as photons in a light beam or electrons in an electric current. The measurements then bear on macroscopic quantities, such as light flux or electric current, which are classical in character but result from averaging the results of quantum calculations. Quantum physics was the key to understanding how electric current behaves in different materials, such as semiconductors, or how light is emitted by matter. Fantastic applications resulted from that understanding, including transistors and integrated circuits as well as lasers. These applications are at the root of the information and communication society. They have transformed our society as much as the heat engine did in the 19th century.
The second quantum revolution, which started in the 1980s, is based first on the understanding that entanglement, a quantum property of two or more particles, is yet more revolutionary than wave-particle duality for a single particle. To make a long story short, the trajectory of a particle, as well as the propagation of a wave, are notions that we describe without difficulty in our ordinary space time. The conceptual difficulty is to apply both notions to the same object. But two entangled objects can only be described consistently in an abstract mathematical space, which is the tensor product of the spaces of each particle. If one insists on describing the situation in our ordinary space time, then one must face surprising behaviors such as quantum non-locality; in other words, the fact that in our space time there seems to be an instantaneous interaction between spatially separated quantum objects.
The other ingredient of the second quantum revolution, crucial for the development of quantum technologies, is the experimental ability to isolate, observe, and manipulate individual quantum objects, such as electrons, ions, atoms, and photons. With such individual quantum objects, one can develop quantum bits — or qubits — which are basic elements of quantum information, much the same as ordinary bits are basic elements of classical information.
Managers who are not knowledgeable in quantum science often ask the question: “What is the best qubit to invest in massively?” I have been asked that question many times, including by European Union program deciders. The answer is: “We do not know, and it is likely that there will not be a single qubit better than the others for all the functions we want to realize.”
This Report scans the solutions explored in quantum computing and correctly distinguishes the various kinds of devices that are too often confused. Universal quantum computers may or may not exist in the future, but a minimum development time of one decade is rather on the optimistic side. So investors must be patient and open to high-risk, long-term investments. I am more optimistic regarding quantum simulators — an assembly of qubits with interactions designed to produce an entangled system whose fundamental state is hard to compute with classical high-performance computing.
Will the second quantum revolution, which is primarily a conceptual revolution, produce a technological revolution as disruptive as the first quantum revolution? I have no idea, and I doubt that anybody has a trustable answer to that question. But I have no doubt that some quantum technologies will be useful and provoke a revolution in some niches. For instance, I believe quantum random number generators based on a single photon falling on a beam splitter should overcome all other random number generators when security is at stake.
Quantum key distribution (QKD) based on entangled photons permits secure communication based on the fundamental laws of quantum physics rather than on relying on the hypothesis that an adversary has about the same level of technological or mathematical sophistication. QKD would be useful in diplomacy, where the disclosure of confidential information after a few years may cause serious problems. Quantum simulators also may be the solution to important problems such as the optimization of the electricity grid.
And it should be emphasized that quantum advantage might be something beyond making calculations faster. For example, what if a quantum simulator or a quantum computer was delivering the same result as a classical computer in a comparable time but with a much lower energy cost?
— Alain Aspect, Nobel Prize in Physics 2022
Preamble: Quantum computers are quantum
John Gribbin’s In Search of Schrödinger’s Cat is one of the first books I read on the history of physics. That was in high school, almost 30 years ago. It was also through Gribbin’s book that I first dipped my toe into quantum physics — notably with the famous analogy of Schrödinger’s cat, which is both dead and alive. The analogy is frequently used to explain certain disturbing behaviors of matter at atomic scales, such as the fact that an electron can simultaneously be in two places at the same time. Quantum physics is magical!
It wasn’t until years later, when I was doing my PhD in theoretical and numerical physics, that I heard about the quantum computer — almost 20 years ago. The quantum computer was also magical, and all the more so as popularized in the media, where QC is generally described in contrast to the classic computer, which uses bits (zeros and ones) that are combined using logic gates to perform operations. Instead, the quantum computer uses qubits. These qubits can have several values at the same time, allowing us to perform many operations at the same time. The complexity of the underlying physics and mathematics is usually obscured by the qubit’s exotic and magical properties.
In short, the subject has remained extremely mysterious to me, as is true for most people, I suppose.
The mission of Blue Shift by ADL — the forward-looking, techno-strategic think tank that I have the honor of leading — is to help executives from our industrial ecosystem to better see through the technological fog and thus better anticipate business and societal disruptions. One of the hallmarks of Blue Shift is drawing on the sometimes provocative perspectives of executives, experts, philosophers, and artists to create fresh thinking and illuminate some unexpected insights on technologies from within and beyond business. As an example, we share in this Report contributions from two artists that highlight some unexpected insights from outside the business realm.
In developing this Report, our team has been on a journey to the edge of the “Quantum Realm.” And if I had to summarize what we discovered, I would say:
The quantum computer is quantum: it does not exist, and yet it exists!
This summarizes well one of our key messages: even though full-scale quantum computers do not yet exist to solve real business problems, many prototypes do exist, and business disruptions are to be anticipated in the coming years. And because the topic is complex, the learning curve is very steep. Most companies should therefore start diving into it today!
But before diving in, I would like to share two anagrams I found for “ordinateur quantique” (quantum computer):
tout indique: “arnaquer” (everything indicates: “rip off”)
rare, quoiqu’ inatendu (rare, though unexpected)
They may sound a little pessimistic but, as always, anagrams move in mysterious ways. I wonder what noted French physicist and philosopher of science Etienne Klein would think of them. What do you think?
— Dr. Albert Meige
1
What is quantum computing?
QC is currently attracting billions of dollars in investment, both private and public, as well as extensive media attention. For those businesses not already engaged in the ecosystems that are researching and developing QC technology, navigating the future is challenging. The field is difficult to understand technically, even for smart generalists, and there are many announcements and claims of breakthroughs in the media that can be misleading.
The aim of this Report is to provide an overview of the state of play for business readers not familiar with the domain and to address the following questions:
- What is the current status of technology development in QC, including potential impacts and timescales?
- What are the potential use cases and applications for business so far?
- What should businesses be doing now to prepare for what is to come?
This Report focuses particularly on the state of play today. It covers QC specifically and makes only passing reference to other quantum technologies, such as quantum sensing and quantum communications.
Inevitably, the analysis and conclusions in this Report are at a high level given the huge scale and complexity of the QC domain.
Understanding the basics
QC is one of today’s most talked-about technologies. It is also one of the least understood, in part because of the complexity of quantum mechanics itself. A quantum computer operates in a completely different manner to a conventional computer, requiring a deep knowledge of physics to really understand how it works.
Rather than using bits, which can have a value of 0 or 1, quantum computers use qubits, which can be both 0 and 1 simultaneously. This phenomenon is known as superposition (see Appendix for a glossary of QC terms), and the value of its readout is probabilistic rather than deterministic. When linking multiple qubits through the process of quantum entanglement, every added qubit theoretically doubles the processing power. Placing a number of entangled qubits in an array and applying gate logic operations to them allows computing power to be harnessed. Figure 1 provides a very high-level schematic of the principles behind how a quantum computer operates.
This exponential growth in calculation capacity as qubits are added means that quantum computers can solve certain types of problems beyond the power of conventional computers. These types of problems are referred to as “intractable problems,” and they involve complex systems with multiple elements, variables, and interactions, where the number of potential outcomes scales up exponentially. There are many such intractable problems in the natural and human worlds, such as optimizing logistics operations between multiple nodes, or predicting weather patterns. In general, the applications can be split into four categories:
- Simulation. Complex physical and molecular simulations, such as so-called in-silico simulations, which could enable digital development of new materials, chemical compounds, and drugs without the need for lab experimentation.
- Optimization. Optimization of complex systems, such as logistics planning, distribution operations, energy grid systems, financial systems, telecoms networks, and many others.
- ML. Artificial intelligence (AI) and ML applications require very high processing power, stretching the limits of conventional computing. The applicability is large but the “quantum advantage” for these types of applications is much less obvious in this field, particularly since ML usually involves training with a lot of data.
- Cryptography. A quantum algorithm has already been developed to conduct rapid integer factoring, which poses a disruptive threat to conventional cryptographic protection approaches for IT, finance, and so on. Quantum technologies will be critically important for future cryptographic solutions. Quantum cryptography can be used to secure secret key distribution but does not rely on a quantum computer. Post-quantum cryptography is a new breed of classical key distribution that is resilient to quantum computer–based key breakings.
Quantum computers are unlikely to ever replace conventional computers for the majority of applications. However, assuming they reach maturity, they will still have a transformative effect on our ability to solve these types of intractable problems, either stand-alone or in combination with conventional computing systems. We will consider applications further in the chapter “Understanding QC applications across sectors.”
Navigating the QC hype
The huge potential of quantum computers, together with a recent acceleration of development activity and announcements of large-scale investments, has ensured a wave of interest, especially over the last few years. Countries, technology players, academia, and startups have all announced QC plans and strategies, with declared public investment alone in excess of $25 billion.[1]
All this noise may lead casual observers to believe that quantum computers will appear, fully working, in a short time frame and available for use across the board, which is certainly not the case. This misunderstanding is due to three issues:
- The difficulty of understanding QC concepts, which makes it hard for nonspecialists to properly analyze current development activity and announcements.
- The sheer volume of announcements of “breakthroughs” from players. Although the main players declare that they are anxious to avoid overhype, to avoid a “quantum winter” or “trough of disillusionment” if progress slows down, they nevertheless need to attract investment and position themselves favorably, and this inevitably leads to breakthrough claims being given a positive spin.
- Linked to the difficulty of understanding the complexity of QC, many media news stories are inaccurate or oversimplified, giving a false impression of current progress.
What is true is that the coming few years and beyond promise many new prototypes and developments that could provide a breakthrough (see also Chapter 4, “Understanding QC software algorithms”). That said, there are still some major challenges to be addressed.
The challenges to QC emergence
Unlocking the potential power of QC will require overcoming three large-scale challenges in the areas of physics, engineering, and algorithms. Solving these issues is not easy and in many cases will require significant work at a fundamental level.
Physics challenges
Several theoretical physics challenges remain. Currently, it is difficult to create scalable quantum computers with low enough error rates, due to the problem of noise affecting qubit stability, causing a phenomenon called “decoherence.” Errors in each gate operation multiply up, so a 0.005% error rate can quickly rise to more than 50% with multiple gate operations. This greatly limits the ability of quantum computers to solve complex algorithms. Physical qubits can be combined to provide error correction, although very large numbers of physical qubits are needed — currently at a ratio of up to 1,000 physical qubits for each logical qubit.[2] Finally, some required concepts, such as “quantum memory” (the equivalent of RAM on a conventional computer)[3] do not yet exist.
Engineering challenges
Building quantum computers is complicated and costly. To begin, to overcome decoherence qubits require isolation, meaning they need very low temperatures, delivered through expensive engineering solutions. These enabling technologies are currently complex and require significant investment (e.g., superconducting cabling, microwave generation, and readout systems).
Algorithm challenges
Ensuring that quantum computers actually deliver operationally is also a hurdle. Harnessing the power of quantum computes requires specific algorithms. These algorithms can be tested on quantum computing emulators running on conventional computers or on the limited quantum computers now available. However, this testing is restricted by the constraints of conventional computing power, making it difficult to show concrete, real-life applications that solve otherwise intractable problems.
Additionally, there are practical problems to overcome in achieving true exponential speedup versus conventional computing, such as the difficulty of data preparation and loading and the real costs of quantum error correction. Lack of available QC hardware of sufficient scale means that practical testing of algorithms is often impossible.
Nevertheless, despite these challenges and concerns over industry hype, businesses cannot afford to ignore QC technology. Otherwise, they risk being left behind if and when a breakthrough occurs, since the adoption rate post-breakthrough could be extremely rapid, and the learning curve is daunting for those with little or no knowledge and experience. QC has the potential to transform operations for specific businesses in specific areas, delivering enormous potential competitive advantage.
In the next two chapters, we will focus on the state of play of development on both the hardware and software sides, and how some of the major challenges are being addressed.
2
Understanding QC hardware
QC architecture requires extremely complex and expensive hardware, both for the processors themselves and for their enabling infrastructure. Many of these technologies are still immature, unproven, or even at a theoretical stage. However, given the current wave of investment and focus on QC, the likelihood of a commercial-scale machine entering the market in the next five to 15 years has significantly increased.
In this chapter, we explore the categories of quantum computer that exist today, the hardware side of a quantum computer, and the multiple approaches being pursued for generating qubits suitable for use in computer processing.
Various computing paradigms
Conventional computer architecture is based on a central processing unit with short-term memory for rapid data access. A quantum computer’s architecture is fundamentally different. There are several categories of quantum computer (or several computing paradigms), as illustrated in Figure 2.
Starting on the left-hand side of Figure 2, quantum emulators are conventional supercomputers that are used to test, debug, and verify quantum algorithms. They are not quantum computers themselves but are still an important tool in the development path.
Next are two categories of analog quantum computer: annealers and simulators. Annealers use low-quality qubits that are set to converge toward the solution, often the result of a search for minimal energy. The main applicability for annealers is for optimization and simulation problems, although there are major challenges with scalability to tackle complex algorithms. The vendor D-Wave leads work in this category, and quantum annealers are already accessible to users.
Quantum simulators refer to a broader category of analog device designed to model complex quantum mechanical systems. Up to now, they have been applied mainly to address physics challenges in the laboratory, as they have only been suitable for addressing certain types of problem. No major commercial vendors are involved in the development of quantum simulators. However, for certain specific optimization and simulation problems, they may actually achieve quantum advantage sooner than gate-based computers.
Universal gate–based quantum computers, which use logic gates like classical computers, have the broadest general-purpose application. They are therefore the primary focus for investment and development. For the remainder of this Report, we focus on this type of quantum computers.
The overall aim is to develop large-scale quantum computers (LSQC). These are fault-tolerant devices with high levels of error correction that can tackle much more complex algorithms at scale by having a high number of stable qubits. However, these are not expected to be available for at least a decade, meaning that current roadmaps focus on so-called noisy intermediate scale quantum (NISQ) devices. As these have a limited number of noisy (as opposed to stable) qubits and no error correction, they only outperform supercomputers for certain algorithms that do not require large numbers of instructions.
There are also hybrid QC concepts in which the quantum computer is used as an accelerator to a conventional supercomputer. These are described in more detail in the section “Taking a hybrid approach.”
Inside the quantum computer — how it operates
Gate-based quantum computers, sometimes referred to as universal quantum computers, use logic gates to perform calculations. This is analogous to the AND/OR/NOT gates in conventional computers, although quantum gates operate differently.
In a typical universal gate–based QC setup, a conventional computer acts as a host to control QC operations, providing its input and output interface, using control and readout electronics. The seven key elements of a basic gate-based quantum computer using superconducting qubits (shown in Figure 3) are as follows:
- Qubit register — collections of qubits that store the information manipulated in the computer. To make a parallel with classical computing, this could be considered as memory, but with the big difference that processing is also done directly in the quantum register.
- Quantum gate controller — physical devices that act on the quantum register qubits, both to initialize them and to perform quantum gates on them, according to the algorithms to be executed. They can also be used to manage error correction codes.
- Error correction — implemented with special code operating on a large number of logical qubits (see “Algorithm challenges,” above, for background on logical and physical qubits). As of 2021, no quantum computer is large enough to accommodate logical qubits, as the ratio of physical to logical qubits exceeds the maximum number of qubits currently available. This is a major challenge for scale-up.
- Qubit measurement — the process used to obtain the result at the end of the execution of an algorithm’s quantum gates and to evaluate error syndromes during quantum error correction. This cycle of initialization, calculation, and measurement is usually applied several times to evaluate an algorithm result, and the result is then averaged to a value between 0 and 1 for each qubit. The values read by the physical reading devices are then converted into digital values and transmitted to the conventional computer.
- The quantum chipset — normally includes registers, gate controls, and measuring devices. It is fed by microwaves, lasers, and the like to manipulate the qubits, depending on their type. The chipset for superconducting and electron spin qubits is small, measuring just a few square centimeters in size. It is usually integrated in a purified oxygen-free package.
- Qubit control electronics — drive the physical devices used to initialize, modify, and read the qubit status. In superconducting qubits, quantum gates are activated with microwave generators.
- Cryogeny — keeps the inside of the computer at a temperature close to absolute zero for most qubit types. Room temperature operation is a key development objective, but practical architectures for this are not yet operational.
A range of qubit approaches
Creating qubits and keeping them stable is an enormous technical challenge. They are inherently unstable and tend to “decohere,” which destroys the properties of superposition and entanglement, removing their ability to provide computing power. Error correction is possible, but this requires many additional physical qubits, as described earlier.
There are seven major approaches to creating qubits currently under research and development, with varying degrees of maturity. Each approach uses a different physical phenomenon to create a qubit, based on a variety of particles from superconducting electrons through to ions, photons, and atoms. These are outlined in Figure 4, with further details and explanation of technical maturity included in the Appendix:
- Superconducting qubits are based on electrons at superconducting temperatures (i.e., close to absolute zero). Under such conditions, electrons collectively behave like a single particle with an energy level that can be controlled accurately.
- Electron spin qubits are based on the spin orientation of an individual electron trapped in a semiconductor potential well.
- Photons can be used as qubits, exploiting their polarization orother physical characteristics.
- Trapped ion qubits are based on ionized atoms electromagnetically trapped in a limited space and controlled by lasers or microwaves.
- Cold atom qubits are based on cold atoms held in position of vacuum with lasers.
- Nitrogen vacancy (NV) centers are based on the control of electron spins trapped in artificial defects of crystalline carbon structures.
- Topological qubits are based on so-called anyon particles, which are “quasi-particles” (i.e., particle representation models that describe the state of electron clouds around atoms) in the superconducting regime. The concept involves engineering their topology to provide additional resistance to interference (there is no working prototype so far).
In addition, so-called Flying Electron qubits, based on electron transport circulating on wave guide nanostructures built on semi-conductor circuits, are being pursued at the basic research level only, with no prototypes or vendors involved at this stage.
Currently, superconducting technology is the most mature approach for creating qubits and consequently seems likely to become commercially available for practical use in the next decade. However, at this early stage in QC development and with the enormous complexity involved, it is too early to pick a winner. Other technologies could achieve breakthroughs and draw level with — or overtake — superconducting, meaning it is vital to keep a constant eye on R&D progress. Each technology has its own benefits and drawbacks. For example, superconducting technology has good scalability, but noise levels are high, while cold atom technology has longer qubit coherence times, but there are challenges in scalability of the required enabling technologies, and applicability has mainly been for simulators thus far. Photon technology has the advantage of room-temperature operation, but also has scalability challenges. Trapped-ion technology has achieved the best stability and error rates, but has not yet been scaled beyond 32 qubits. Electron spin has the potential for good scalability, leveraging existing silicon semiconductor manufacturing expertise, but has only been demonstrated at small scale and manufacturing costs could be high.
Complex enabling infrastructure
As explained above, ensuring that qubits remain stable is a major technical challenge. Most, but not all, approaches require very low operating temperatures (close to absolute zero). This means that supporting infrastructure is usually complex and costly, including equipment such as cryogenic refrigeration units, superconducting cables with complicated wiring, microwave generation and readout systems, low-temperature thermometers, and lasers, as described in Table 1.
The state of hardware research
Despite the excitement, none of the approaches explained above has matured beyond being demonstrated at a proof-of-concept stage at lab scale. However, companies have published extensive roadmaps for creating new prototype quantum computers, with more than 20 devices promised in the run-up to 2030 (see Figure 5). Many timescales seem overly optimistic given current technical challenges and uncertainties, although breakthrough innovations could change this. Instead, it is perhaps more realistic to assume that the milestone of creating a fault-tolerant LSQC will occur between 2030 and 2040.
Qubit numbers quoted in vendor roadmaps should be interpreted carefully. Generally, vendors quote numbers of physical qubits. However, the relationship between physical qubits and logical or “usable” qubits that take into account error correction varies considerably. For example, IonQ claims a much lower ratio of physical to logical qubits than other vendors. It is also important to be aware that development is not just about making devices with increasing numbers of qubits. It is also about addressing a range of technical and engineering challenges in both the core and enabling technologies.
The challenges of scaling up
As we mentioned, achieving the necessary scale-up for commercial operation is one of the greatest challenges developers face. As well as aiming to make devices with ever-increasing numbers of qubits as shown in the roadmap, it is also worth mentioning the potential of networked quantum computers, which is another area of intensive research. In theory, if we were able via the cloud to seamlessly access a network of quantum computers to perform a computing task, this could be another way to achieve scale (i.e., scale out rather than scale up). This could also, in theory, enable different types of quantum computer to perform different tasks for which they are best suited (e.g., combining superconducting with cold atom). This would naturally also include conventional high-performance computers (HPCs) working in combination in a hybrid mode. There are, however, still major technical challenges to achieving such a network in practice (e.g., in terms of interconnectors and repeaters). The existence of new standards and specifications for interoperability would also be a key prerequisite.
Interlude #1 — Quantum chandeliers
In the collective imagination, the quantum computer is a machine from science fiction, with mysterious ways of working and extraordinary powers.
The illustration is composed as a triptych. In the center are individuals dehumanized by quantum supremacy, which appears like a dream — or a nightmare. On either side, quantum chandeliers remind us that this is a machine. It is not a dream but an imminent technological reality. The quantum computer is no longer just a fad fed by fantasies from popular culture. It is a promising, heavily funded research project.
— Samuel Babinet, artist
3
Understanding QC software algorithms
Just as with conventional computers, quantum machines require software to run on top of their hardware to generate results. However, gate-based quantum computers require the use of quantum algorithms. These use very different concepts than traditional programming, making their creation a specialist field.
Research into quantum algorithms can, to some extent, be uncoupled from quantum hardware and run on conventional computers acting as emulators. This means that progress to date on algorithms has preceded hardware development. Mathematicians have been able to work since the mid- and late 1980s on creating algorithms for quantum computers and emulators. This led to the first quantum algorithms that were published in the early 1990s. Today there may be more than 450 known quantum algorithms in existence, and constant new activity adds to this total.
The categories and uses of quantum algorithms
Forming the basis of all applications currently being considered are six broad categories of quantum algorithms, based on their use case:
- Search algorithms that speed up the search for structured or unstructured elements in a database or big data implementations.
- Complex optimization algorithms find solutions to combinatorial optimization problems, such as route or supply chain optimization, financial portfolio optimization, and insurance risk assessment.
- Quantum ML aims to improve ML and deep learning models in areas such as autonomous driving, customer segmentation, and the automatic detection of diseases.
- Quantum physics simulations, covering the optimization of inorganic/organic chemical processes, biochemical process simulation, and the design of new materials.
- Biological molecule simulation, for drug development and atom/molecule simulation.
- Cryptography algorithms that can break current encryption standards but also provide the ability to create new, stronger encryption protection.
Limitations to current algorithms
Being able to speed up quantum algorithms is crucial to proving that they can solve intractable problems and outperform conventional computers. However, while quantum algorithms are available now, their application remains practically limited by hardware considerations. Factors such as gate dependency, the number of times the algorithm must be run (the shot count), the number of qubits available, data preparation time, and error correction all impact speed, and therefore results. Data preparation is a particular, often-overlooked issue as data loading can have a significant time cost, currently making QC inadequate when carrying out big data computations.
All of this means that demonstrating speedup compared to conventional algorithms is not straightforward. This was highlighted by Google’s 2019 announcement in which the company famously announced the achievement of “quantum supremacy” (i.e., solving a problem faster than a conventional computer could in any feasible amount of time). However, the chosen task was difficult to compare with any known conventional algorithm, and since then others have managed to solve the problem relatively fast (less than a day) and with fewer errors using conventional computing methods.
Current hardware constraints mean that in many cases relatively simple algorithms can be run, but true benefits will require fault-tolerant, large-scale quantum computers that are unlikely to be ready until the 2030s at the earliest. For example, emerging NISQ computers cannot run “deep” algorithms due to error rates, meaning they can only use algorithms that chain together a small number of quantum gates, limiting their application.
Taking a hybrid approach
In the short term, hybrid algorithms provide a promising, alternative approach to achieving quantum advantage. As the name suggests, these combine conventional and quantum approaches, with the computation broken down into a smaller quantum component and a larger conventional computation component. A typical hybrid setup involves loop iteration between the conventional and quantum processing units (see Figure 6).
Essentially, this hybrid setup means that the quantum computer is focused on the part of the computation that plays to the strengths of current quantum machines, while the conventional machine handles everything else. This approach accelerates performance and potentially overcomes the limitations of NISQs, whose error rates limit their practical processing capacity, enabling applications to scale. Hybrid algorithms are not restricted to certain qubit types and are claimed already to be feasible on machines of between 20 and 1,000 qubits processing power. However, tight design integration between the different parts of the hybrid quantum computer is required if each element is to perform effectively.
There are many current algorithm providers, for example:
- Avanetix — supply chain issues in automotive and logistics.
- Groovenauts — retail planning optimization and others.
- AI — combines ML with quantum algorithms to simulate organic chemistry and design enzymes, peptides, and proteins.
4
Key players and activities
The last few years have seen a major acceleration of investment in QC from players across the ecosystem, including countries, research institutions, major technology companies, and startups. Venture capital funding increased by 50% between 2020 and 2021, reaching over $1 billion.[4] The landscape is very extensive, as Figure 7 illustrates.
Each group of players has its own motivations for quantum research and is targeting distinct positions in the ecosystem. Many players are aligned with only one or two of the core qubit technologies.
Government-level research
Countries see building strengths in QC as delivering a strategic and technical advantage. Not only does it underpin specific, highly complex use cases, but QC also has significant defense and security implications. For example, current public cryptographic keys are highly vulnerable to be cracked through QC algorithms, meaning that creating new capabilities is vital for protecting national infrastructure (and potentially disrupting the infrastructure of rivals).
Consequently, more than 20 countries have significant QC R&D programs, ranging from New Zealand to Singapore (see Figure 8). R&D activity is distributed globally, with strengths across US, Europe, China, and the rest of Asia.
The US remains the leading country in quantum development, through its combination of high public investment in basic research, global tech giants, and a well-funded startup ecosystem. China is aiming to leapfrog America and has claimed it will invest $10 billion in the period up to 2030, although this total is open to question. China also suffers from a current lack of basic research strengths. Overall, it can be difficult to correctly measure exact government investment given differences in splitting QC from related technology spend, coupled with a lack of data on defense-related investment. The real level of funding for the US, for example, is likely to be higher than reported in Figure 8.
Examples of national/regional programs include:
- China — a major focus on quantum communication, with large investment in the complete range of quantum technologies.
- Germany — diverse areas of research and strong collaboration, with a focus on creating industrial applications in key sectors.
- France — building strong ecosystems between industry players, startups, and researchers with a focus on both quantum computers and enabling technologies.
- UK — targets computing, security, and sensing with a strong focus on medical imaging and has the third highest number of startups globally.
- US — focus on error reduction, techniques for interconnecting cubits, new quantum algorithms, and quantum networking/telecoms to counter Chinese investment in this area.
- Canada — aims to drive innovation through cooperation between universities and private companies, such as QC pioneer D-Wave Systems.
- EU — the European Quantum Flagship involves over 5,000 researchers from France, Germany, Italy, the Netherlands, Spain, and Switzerland. The long-term goal is to develop a “quantum web” of computers, simulators, and sensors.
- Japan — startups specialized in software, with major players focusing on quantum telecoms and cryptography.
Research institutes & universities
Given their relative size, most research institutes are focused on specific areas within QC, often working closely with national programs, industry players, and startups. A nonexhaustive list of examples includes:
- Massachusetts Institute of Technology (US) — research into quantum sensing with diamond, trapped-ion qubits, quantum photonics, and computational algorithms.
- University of Maryland (US) — focuses on QC architectures, algorithms, and complexity theories.
- Yale (US) — research includes quantum sensing and communications, superconducting, and photonic qubits.
- University of Science and Technology of China (USTC) National Laboratory for Quantum Information Sciences (China) — focuses on QC and metrology for military and civilian applications.
- NLQIS National Laboratory for Quantum Information Sciences (China) — recently launched laboratory focused on QC and metrology for military and civilian applications.
- Max Planck Institute (Germany) — research into quantum optics and fundamental research on quantum simulator and cold atoms in optical lattices.
- Fraunhofer Institute (Germany) — focuses on application-oriented research and operates the most powerful quantum computer in Europe with IBM.
- Commissariat à l’Energie Atomique et aux Energies Alternatives (CEA)-Leti (France) — leading laboratory for applied research on electron spin and photonic qubits and their fabrication.
- CEA-List (France) — research in software engineering and cryoelectronics.
- CEA-Direction de la Recherche Fondamentale (DRF) (France) — research in superconducting technologies.
- Centre national de la recherche scientifique (France) — fundamental research in physics, mathematics, and algorithms.
- National Institute for Research in Digital Science and Technology (Inria) (France) — research on quantum technologies, quantum error correction, cryptography, and quantum algorithms.
- University of Cambridge (UK) — research focused on quantum algorithms, simulations, metrology, and cryptography, alongside the foundations of quantum mechanics.
- University of Oxford (UK) — leads the UK’s QC & Simulation Hub, working on creating a network of trapped-ion quantum computers.
- University of Bristol (UK) — dedicated research group on photonic QC.
- University of Waterloo (Canada) — research focused on error correction and fault tolerance, quantum algorithms, ultracold atoms and trapped ions, spin-based quantum information processing, and cryptography.
- Institut Quantique (Canada) — focused on process/control of quantum information in low-temperature environments and theoretical research in design of qubit-based systems and algorithms.
Global technology companies
Most technology companies are focusing heavily on superconducting QC technologies, although some (e.g., Microsoft and Honeywell/Quantinuum) have also embraced other approaches:
- Amazon — entered the quantum cloud market at the end of 2019, and its cloud offering currently covers quantum computers from D-Wave, Rigetti, and IonQ. It is currently developing an in-house quantum computer using so-called cat-qubits (superpositions of two coherent sets of fluctuations with opposite phases in the same oscillator), which are said to be more resistant to some forms of error, such as environmental noise.
- Google — focusing on superconducting qubits, Google has the ambition to build an error-corrected quantum computer within the next decade and create a machine with 1 million qubits by 2029.
- Honeywell/Quantinuum — Honeywell created Quantinuum as a standalone business together with Cambridge Quantum in late 2021. It is building a trapped-ion quantum charge-coupled device (QCCD), using ytterbium-based ions coupled with barium ions to provide cooling.
- IBM — a main focus is on superconducting qubits, with additional fundamental-level research on electron spins and Majorana fermions qubits. It launched its 127-qubit Quantum Eagle processor in late 2021 and plans to release its Condor 1,121-qubit machine inside a large dilution “super fridge” in 2023.
- Intel — main focus is research and development on silicon qubits. The company produced a wafer with 26-qubit chipsets in 2017 and has made some progress in manufacturing quality and cryogenic control electronics since then.
- Microsoft — R&D focus is on the development of the topological qubit, which has not yet been demonstrated practically. On the cloud side it introduced Azure Quantum, a full-stack, open cloud ecosystem in 2019.
- Thales — working with academic partners in France and Singapore, Thales is focusing on quantum sensors, quantum communications, and post-quantum cryptography, using cold atom technology, NV centers, and superconducting devices.
- Alibaba — invested with USTC to start the Alibaba Quantum Computing Laboratory in Shanghai, focusing on quantum cryptography and QC. They develop superconducting fluxionium qubits.
- Tencent — launched a Quantum Lab in 2018 based in Shenzhen, offering QC resources in the cloud as well as work in quantum simulation and ML algorithms.
There are an estimated 450 startups and small businesses globally focused on quantum technologies, some of which are very well funded. They play key roles in prototype development and commercialization of specific QC technologies. Notable startups include D-Wave, creators of one of the first commercially available quantum simulators, as well as Rigetti, IonQ, and QCI. Some other significant startups have also been mentioned in the highly selective list below:
- Alice & Bob — focused on developing self-correcting qubits to develop fault-tolerant and universal gate–based QC. Alice & Bob has created a qubit immune to quantum state reversal, one of the two main types of errors quantum computers suffers, and has raised over €27 million.
- ColdQuanta — founded in 2007 in the US, ColdQuanta develops laser-based solutions for cooling cold atoms and is also designing a cold atom-based computer.
- D-Wave — the main commercial player in quantum annealing, it has developed an end-to-end quantum annealing computer solution. The company has installed more than a half-dozen quantum computers at customer sites and operates more than 30 in its own facilities, with more than half of them dedicated to cloud access. Over 250 early quantum applications have been developed through D-Wave, its customers, and partners, although only a few of them are production-grade.
- IonQ — offers full stack software adapted to its ytterbium (and now barium) trapped-ion quantum computer. It plans to make rack-mounted quantum computers small enough to connect in a data center by 2023.
- Quantum Computing Inc. (QCI) — created Qatalyst, a high-level software development and cloud provisioning tool. This enables developers to solve constrained optimization problems using gate-based accelerators, quantum annealers, or conventional computers.
- Quantum Motion — created in 2017 as an Oxford University spin-off, focusing on developing high-density silicon quantum computers.
- PASQAL— a spin-off from the French Institut d’Optique, PASQAL is working on quantum processing units made up of hundreds of atomic qubits in 2D and 3D arrays, combined with a programming environment to address customer needs in computing and simulation of quantum systems. It delivered its first 100-qubit system in 2022, running in the cloud through OVHcloud and soon, on Microsoft Quantum Azure cloud.
- PsiQuantum — founded in 2016 by Jeremy O’Brien, a former Stanford and Bristol University researcher, focusing on creating a photon-based quantum processor in complementary metal–oxide–semiconductor (CMOS) silicon technology.
- ORCA Computing — an Oxford University spinout aimed at developing scalable photon-based quantum computer systems based on the concept that “short-term” quantum memories could synchronize photonic operations.
- Rigetti — launched the world’s first multi-chip quantum processor, the 80-qubit Aspen-M system. It operates along the entire technology stack, designing and fabricating quantum chips and processors, managing the QC control system, and providing cloud-based services and software tools.
- QuantumCTek — a Chinese company focused on quantum-enabled ICT security products and services.
Remarks on the QC market
The QC market does exhibit some noteworthy characteristics:
- The scale of activity is already significant, yet we are still a long way from commercialization. QC devices are already available for use via the cloud (e.g., D-Wave’s quantum annealer and IBM’s 127-qubit machine), but customers are still almost entirely from the academic and corporate R&D communities.
- The QC market is somewhat unique in that it has been born essentially “cloud-native,” meaning that from its earliest stages it has been developed to be accessed via the cloud. It seems likely that this will continue to be overwhelmingly the case, although there may in future be some customer segments, e.g., governments and some industrials that would have different requirements.
- So far, there is a relatively high degree of openness from the key players in communicating their R&D results and roadmaps. At this stage of maturity, it is in everyone’s interest to be open, and extended partner ecosystems are essential to tackle the complex challenges involved. There may well be a stage further down the development and commercialization path where this changes, but the ecosystem currently is fairly open and transparent.
- The market can be expected to continue to expand if perceived progress is rapid enough to meet current expectations. What is needed for commercialization is a match of supply and demand: the availability of devices that can provide adequate performance and the availability of customers with requirements that match the performance on offer. This is another important reason for potential customers to become engaged in the market sooner rather than later.
5
Understanding QC applications across sectors
According to vendor roadmaps, the first commercial 1,000-qubit quantum computer could be available in 2023, with a 1 million-qubit machine launched by 2030. However, as discussed previously, these estimates should be treated with caution, and timescales of 2030-2040 are more realistic for the availability of large-scale quantum computers.
This does not mean that users need to wait until the next decade to start with QC. While it is a non-gate-based machine, the D-Wave analog quantum annealer is already available on the market, with customers either buying a machine for an estimated $15 million or accessing quantum capacity as a service from D-Wave or Amazon. Some customers are paying up to $200,000 per year for usage. IBM devices are also available via the cloud.
Given that the physical number of quantum computers is likely to remain small and purchase prices high, this cloud-based model of access probably will be the predominant way of accessing QC capacity, with the possible exception of, say, government or large corporations that might have the necessary resources to acquire and maintain their own devices. Typically, vendors will offer QC alongside existing supercomputer capacity to meet customer needs.
The quantum annealer and other forthcoming machines can handle simpler algorithms such as material design or small-scale protein folding and have already benefited some customers.
Ultimately, the goal of the cloud access providers is to offer a seamless service that could integrate networks of quantum and conventional devices to match the needs of the problem to be solved.
It is important to remember that simply demonstrating quantum advantage may not be enough to make a QC application attractive to an industrial customer. Customers will be concerned with the overall benefits, typically involving a trade-off between costs and performance. For example, in some applications, speed of processing may not be the most important criterion.
As QC technology advances, more complex algorithms and problems will be solvable via devices. Developers and end users are increasingly collaborating to create specific applications. We can reasonably expect some applications to be available sooner than others, given the differing complexities involved (see Figure 9).
For example, some successes have already been achieved in demonstrating the feasibility of modeling small-scale protein folding. In general, many observers expect that small-scale simulation applications will be among the earliest to become available.
Overall, there are many potential applications for QC across industry sectors, varying in terms of their relative complexity and timescales for becoming feasible. We have summarized these for selected key sectors below.
Healthcare
Given the high cost and complexity of creating new drugs, QC offers enormous potential rewards to healthcare and pharmaceutical companies, making it a particularly active market. QC can dramatically shorten drug discovery timescales by modeling/simulating drug-to-target interactions and molecular docking, providing better insight into a drug’s performance and viability before entering the lab and performing in vitro testing. This in-silico large-scale drug discovery is still some way (10+ years) off, given the complexity of the problem and currently available QC power.
Other application areas of interest include improving AI and ML for clinical trial planning, optimization of global supply routes, optimizing lab usage, assessing combinations of different treatment options, and supporting analytics for digital health systems.
However, some small-scale applications have already been developed, many using hybrid QC solutions:
- GlaxoSmithKline (GSK), using the D-Wave Advantage annealer and its Leap Hybrid Solver, solved a mRNA codon optimization problem, which worked for 30 amino acids but could be scaled to 1,000.
- Cambridge Quantum is applying EUMEN, a commercially available quantum chemistry application, to Alzheimer’s drug research.
- D-Wave has carried out experiments on minimizing radiotherapy patient exposures to X-rays by simulating the diffusion of electromagnetic waves in the human body.
Protein folding is another area of interest that is key to creating drugs and therapies for destructive diseases, as well as for the wider field of bioengineering. Conventional algorithms cannot handle the intrinsic problem of predicting the three-dimensional structure of proteins, but QC can. IBM has successfully folded a seven-amino acid neuropeptide using a nine-qubit machine and the 10-amino acid chain Angiotensin using a 22-qubit device. Biogen, 1QBit, and Accenture are aiming to retarget therapeutic molecules in existing treatments for neurodegenerative or inflammatory diseases.
Chemicals
Chemicals applications include in-silico molecular simulation, as well as catalyst design and production/supply chain optimization.
Molecular simulation may be used for chemical process design/improvement, new material development, and catalyst design. It gives insight into the physical and energetic behaviors of different processes and reactions and is difficult to model using conventional algorithms due to the requirement for exponential processing power growth.
Quantum simulations of molecules (in-silico) could reduce or even replace laboratory R&D and physical testing, reducing costs and improving process and material design effectiveness. As these simulations are generally less complex than in healthcare, they should be available to run on quantum hardware relatively quickly, although simulating larger chemical reactions will require a greater number of qubits to deliver results.
Production and supply chains involve multiple players and factors, which all interrelate. Unforeseen variables, such as geopolitical or supply chain disruptions, can all impact production. Use of QC in the chemicals industry should therefore help companies to better model and optimize these complex, fast-moving, and interrelated supply chains.
QC has already been applied at a proof-of-concept stage by multiple companies, including:
- 1QBit and Dow demonstrated a novel approach to achieving accurate and efficient simulation of electronic structures using an IonQ trapped-ion quantum computer.
- IBM, using a 16-qubit superconducting quantum computer, simulated a set of beryllium hydride molecules and their minimum energy balance.
- Microsoft and TotalEnergies simulated carbon capture at a molecular level to store in new complex materials.
- Airbus is using QC for the analysis of aerial photography and design of new materials.
- Utilizing an IBM quantum computer, Mitsubishi simulated the initial steps of the reaction mechanism between lithium and oxygen in lithium-air (Li-air) batteries.
Energy
The energy sector is transforming to achieve net-zero targets. The development of renewables (including batteries for electric vehicles and energy storage) and the deployment of smart grids will pose significant design and management challenges, which quantum computers could help address.
In the case of battery design, digital simulation and modeling of battery chemical and physical processes quickly exceeds the capacity of conventional computers. Quantum computers could provide a solution and help to enable a new era of highly efficient post-lithium batteries.
The increasing deployment of smart grids and distributed energy generation will greatly increase the complexity of power management. Quantum computers could be key to managing this complexity, successfully forecasting, planning, and optimizing power grids. Currently, quantum annealing algorithms for power grid optimization show promise on small problems but lack sufficient scalability to be applied to larger areas.
Examples of QC in the energy sector include the following:
- AMTE Power is using NV center-based magnetic quantum sensing to evaluate the battery aging process and develop lithium-based battery manufacturing solutions.
- BP is designing algorithms for optimizing oil exploration using data from various sensors, particularly seismic sensors, to consolidate models for simulating geological layers.
- The Dubai Electricity and Water Authority is collaborating with Microsoft to develop quantum solutions for energy optimization.
- Samsung is partnering with Quantinuum and Imperial College London to study battery development using QC.
Telecommunications
Telecom QC use cases revolve around cryptography and network optimization. Quantum computers will have the ability to break many of the currently used cryptography systems that protect the confidentiality and integrity of digital communication. To prepare for the almost-inevitable advance of QC power, the US National Institute of Standards and Technology is in the process of establishing standardized quantum-resistant cryptographic algorithms to be available in 2024. By developing and standardizing algorithms for post-quantum cryptography before quantum computers develop their cryptography-breaking capabilities, both governments and businesses will be better prepared to defend their digital services against quantum attacks.
Additionally, in terms of optimization, operators will be able to better plan and optimize their networks using QC. This includes understanding where best to deploy base stations and cells to maximize performance while ensuring financial efficiency.
Several use case implementations have already taken place among telecoms, with larger deployments possible in the future as quantum computer power increases and algorithms develop:
- BT and Toshiba have deployed a pilot 6-km quantum key distribution (QKD; a secure communication method for sending keys) to connect several industrial sites as part of the Agile Quantum Safe Communication program.
- Verizon has launched a three-site QKD trial in Washington, DC, and tested a virtual private network using a post-quantum cryptography solution to connect two sites in the US and the UK.
- TIM has optimized the setup of 4G/5G radio cell frequencies using a D-Wave QUBO-based algorithm.
- SK Telecom has secured its 5G network using quantum random number generator technology to the subscriber authentication center.
Transport & logistics
Transport and logistics quantum applications focus on fleet optimization, supply chain optimization, and traffic planning and management. This includes the routing of vehicles, such as the navigation of autonomous cars.
Supply chain optimization is a complex problem with multiple parties, processes, variables, and constraints involved. For rail, road, and air transportation networks, maximizing the efficiency of fleets, managing large-scale traffic systems, optimizing routes, and improving asset utilization are all key issues. For example, Deutsche Bahn has pointed out that even a simple 20-station railway route optimization problem would take a conventional computer two years to calculate. Yet the company operates a network of 5,500 stations in Germany. This exponentially growing complexity means that QC solutions could be transformative for the transport and logistics industry, and even more so when autonomous vehicles are deployed.
Already, some hybrid algorithms have been developed for flight optimization at a small scale. However, logistics route optimization at more granular levels will require more qubits and hardware stabilization against noise and errors if it is going to scale.
Use case examples in transport and logistics include:
- Sumitomo launched a pilot experiment for optimizing flight routes for urban air mobile vehicles in June 2021.
- Working with D-Wave, Ferrovie dello Stato Italia is working to optimize train arrivals in railway stations and minimize passengers’ connection times.
- Volkswagen has partnered with D-Wave to optimize the routes of a 10,000+ cab fleet in Beijing.
- Toyota will use Kawasaki Business Incubation Center’s IBM computer to analyze big data taken from previous car trips, which can be used to guide vehicles along specific routes to avoid traffic jams.
Finance & insurance
Finance and insurance applications center on improving on supercomputer performance in areas such as portfolio optimization, forecasting, risk modeling, and high-frequency trading, as well as increasing the accuracy of automated fraud detection.
In the financial sector, forecasting, modeling, and optimizing the risk associated with an investment portfolio is crucial. The most commonly used method of achieving this, Monte Carlo simulations, require a very long computational time, often running for days. This currently forces a tradeoff between ensuring problems are complex enough to capture the nuances of the real world without being so complex that they are too computationally demanding for current conventional supercomputers. In theory, the quadratic speedup QC provides can reduce calculations to make them almost real time, allowing enormous improvements in risk assessment. Similarly, rapid execution of complex quantitative buy-sell strategies (e.g., in the domain of high-frequency trading) will improve financial firms’ abilities to generate greater returns while controlling risk.
To spot potential fraud and determine the credit worthiness of applicants, financial services organizations need to analyze enormous data sets that can have millions of entries, each with thousands of different properties. Quantum computers can drastically reduce the cost and time of processing this data, ultimately enabling real-time analysis and response.
However, activities to date are mainly proofs of concept and trials. This is because the quality and fidelity of qubits need to be increased for certain financial algorithms to attain quantum supremacy. Current examples in the financial and insurance sectors include:
- NatWest has used quantum-inspired algorithms running on traditional computers to optimize its investment portfolios.
- Microsoft has devised a method to make stock value predictions using topological computing.
- IBM, JPMorgan Chase, and Barclays have partnered to study the uses of quantum in trade-strategy optimization, investment-portfolio optimization, pricing, and risk analysis.
- CaixaBank has used D-Wave’s quantum cloud and hybrid solver services for its portfolio hedging calculations. It reported a 90% decrease in time-to-solution, while optimizing bond portfolio internal rate of return by up to 10%.
- PayPal partnered with IBM in 2020 to apply QC to improve its fraud detection systems and overall digital security integrity.
Defense & aerospace
Defense and aerospace applications are huge in scale and diversity, ranging across sensing, cryptography, communications, optimization, and simulation. Many actual use cases remain secret, but others have been announced, such as around long-range weather forecasting and optimization of new materials. However, the complexity of many of these applications means that quantum technology will need to develop further before they can be successfully realized.
Current use case examples in the defense and aerospace industry include:
- Lockheed Martin partnered with Google and NASA to test D-Wave annealers in 2014 to develop a solution for formal proof-of-software operation.
- Airbus is using QC for the analysis of aerial photography and design of new materials.
- The US Air Force is aiming to utilize quantum timing, quantum sensing, quantum communications/networking, and QC in a variety of applications.
- The European Center for Medium-Range Weather Forecasts has partnered with Atos to develop a Center of Excellence, where they have access to Atos’ Quantum Learning Machine to research the potential of QC in weather and climate forecasting.
Interlude #2 — Quantum photography
When photography took off at the end of the 19th century, painters found themselves without a means of subsistence. Portraits, which used to be the privilege of rich families, became accessible to the bourgeoisie, and painters had to reinvent their art in order to survive. This turn of events gave birth to a radical evolution in painting with the advent of pictorial movements like Impressionism, Pointillism, or Fauvism. With Cubism, painters even drew their inspiration from cutting-edge science of their time to rethink the theories of representation and developed new ways to visualize three-dimensional objects on a two-dimensional canvas.
Today, photography is about to suffer a shake-up of similar magnitude. Smartphones are everywhere, and figurative photographs are quickly losing both their visual appeal and business value, forcing photographers to rethink their art entirely. While a significant part of the photographic community is returning to the roots with a renewed interest in the many techniques that were developed at the end of the 19th century to produce images from light, another part of the community is considering the digital aspect of modern photography and videography as a core element of their art, with no obligation whatsoever to emulate silver prints.
Quantum photography and quantum video find their roots in the second trend. Instead of considering the artifacts resulting from the pixelation of images as a necessary evil that must be hidden at all costs by a never-ending increase in the resolution of camera sensors, quantum photography proposes to take advantage of phenomena of quantum essence so that these “defects” serve as a basis for the development of an entirely new type of creative process. Quantum photography always starts with a digital photo or video. This approach differs from that of digital artists who synthesize images from scratch using AI. In a sense, quantum photography serves as a missing link between traditional photography and more abstract or conceptual visual arts.
Quantum photography produces a new breed of hybrid images, both figurative and abstract, evolving freely between photography and painting. This duality is reminiscent of the wave (smooth) and particle (granular) models of matter in quantum mechanics; from a visual standpoint, quantum photographs simultaneously show two complementary aspects of our world: the “granular texture” of the microscopic world at the atomic scale and the “smoothness” of the world at our own scale.
Beyond its marketing appeal, the name “Quantum Photography” itself thus refers to several key characteristics of quantum mechanics, such as:
- Particle-wave duality, as explained above (see Fig A)
- Quantification of both space (pixels) and time (video)
- Interférence phenomena (moiré effects for instance)
In addition to physics analogies proper, Quantum Photography also makes use of other global mathematical manipulations of images such as Mathematical Morphology, leading to images that have never been seen before (Fig B, C, and D above) or sometimes look like paintings (e.g., Fig E, which looks like a Cubist portrait). In that respect, the nascent field of Quantum Mathematical Morphology (quantum digital image-processing algorithms based on quantum measurement) may use quantum computers to speed up computations that quickly become prohibitive when processing quantum videos, as such processing is performed on an image-by-image basis, and computations for a single image, even using the best concurrent programming techniques, can sometimes requires tens of seconds of processing time on the most recent desktop computer.
— François Bourdoncle, artist
6
Conclusion: Readying your business for the quantum future
Going beyond the hype, QC clearly offers disruptive potential to solve specific, high-value problems that are beyond the effective capabilities of today’s supercomputers. When these machines will be available is an open question — there remain significant challenges at the physics, engineering, and algorithm levels that need to be overcome to enable success. At best, most current breakthroughs are at the lab scale proof-of-concept stage.
However, as with other emerging technologies, development is not linear. Breakthroughs may unlock and accelerate progress in key areas, with commercialization potentially following relatively swiftly. Consequently, while market growth predictions vary wildly (see Figure 10), they could reach $65 billion by 2030, although the consensus is closer to $20 billion. However, these are more assumptions than predictions given the uncertainties involved.
The QC learning slope is steep. It will not be possible, especially in the earlier stages of commercialization, for inexperienced users simply to do nothing until the technology matures then buy access to quantum processing power and immediately expect to make transformational changes in their business operations. On the other hand, those companies that have already explored potential use cases, understand the sort of algorithmic solutions that could be applied, and have already developed partnerships and relationships in the QC ecosystem, can expect to achieve major competitive advantage.
Another key consideration is how the QC industry will evolve as the technology matures. Currently, there is quite a high degree of willingness to share data and intelligence across competing players, partly because at this stage of maturity it is broadly in everyone’s interests to be open. However, this might change once quantum technology reaches the scale-up stage, when key players will need to safeguard competitive advantage and maximize their returns on investment. A further key issue is the extent to which geopolitics may play a role, especially in light of the critical importance of quantum technology for cryptosecurity and defense. Those companies that lack any internal knowledge and capability could find themselves additionally disadvantaged in such scenarios.
Businesses should therefore take steps now to ensure that they are not left behind if and when breakthroughs occur. Following the four steps in Figure 11 will position an organization to reap the future benefits of QC.
1. Explore applicability
Start by understanding how QC could benefit your business and in which areas it could be applied. Look at your operating model and identify activities, processes, or functions for which simulation, optimization, AI/ML, and cryptography are potentially important. Create a list of specific intractable problems that cannot be solved by conventional computing, prioritizing those that would provide significant, disruptive advantage if they were solved. Focus on answering three questions:
- Where could quantum applications in simulation, optimization, AI/ML, and cryptography deliver advantage in your current business operations (e.g., in terms of cost, speed, performance, efficiency, or quality)?
- Where do you currently use or plan to use high-performance computers but struggle with their limitations?
- Where could you deliver real disruption to your business model or industry by solving intractable problems, or problems that you never before considered solvable?
Being creative is an important aspect of this exercise and involves identifying new radical challenges and opportunities, not just addressing current known challenges and opportunities faster.
Once identified, you can screen and rank these potential opportunities in terms of, for example, scale of business impact, maturity, and likely timescale ranges in the normal way to help inform your strategy.
2. Monitor technology developments
QC is evolving rapidly, with a large volume of new innovations, roadmaps, and research being released. As with any emerging technology, you should ensure you are on top of developments by putting in place a suitable technology monitoring process. This process needs to be of a scale appropriate for your business; for many organizations, a light-touch approach currently may be sufficient.
Any technology-monitoring process has several basic elements:
- Scanning. Flagging areas of interest as they arise using both targeted search tools and public data sources.
- Monitoring. Following identified trends in a systematic way, for example by subscribing to forums, conference attendance, and regular landscape reviews.
- Scouting. Actively engaging with relevant players and encouraging inputs from others.
- Evaluating. Assessing the specific relevance and potential of emerging developments, and sharing tailored intelligence internally within the business.
This means developing or acquiring the capability to understand the technology, setting up the channels to stay abreast of relevant developments, and rapidly evaluating their potential and limitations. An important aspects of this is screening ongoing work on quantum algorithm development targeted at your industry verticals.
3. Engage in the ecosystem
Ecosystem thinking is fundamental to driving effective innovation success. Given the complex, fast-moving nature of the QC market, building collaborations and partnerships with other players is critical. Start by understanding the roles, activities, and level of involvement of different ecosystem players, including government, academia, research institutes, vendors, startups, and investors. Identify those players that are focusing on your sector and establish how your business could become involved in some way — for example, through pilots, cofunding initiatives, staff secondments, or sponsorship of PhD projects. Vendors are looking to collaborate with potential end customers to understand and meet use case needs in the future. Consider working with them on relevant projects to test algorithms, even if performance is not yet powerful enough to deliver breakthrough results. For example, algorithms can already be tested via the cloud with available universal quantum computers, a quantum annealer, or with an emulator.
4. Build knowledge & capability
As part of digital transformation initiatives, most large organizations have built or recruited new capabilities and programming skills, particularly in leading-edge areas such as AI and ML. Based on the significance of the area to your business, invest in educating and training at least some of these individuals in quantum technologies, quantum programming, and quantum algorithms, even if this is at a basic level. This will enable them (and you) to participate effectively in the ecosystem and monitor and evaluate developments. This is especially important for quantum technologies due to their complexity, reliance on specialist expertise, and the level of hype in published media.
In some cases, you might also consider some specialist recruitment. However, you will need to be realistic about the limited availability of specialist QC experts, and typically you may not always need to recruit PhDs in quantum science anyway. It could be argued that you need “quantum application engineers” rather than scientists, a profession that does not yet exist. Hence, a lot can be achieved through education and experience building from within. Creating a motivated and enthused community internally is one of the best ways to ensure that you stay abreast of developments in a natural, rather than wholly process-driven, manner.
Many large potential user companies, especially in the more technology-intensive sectors, have already taken these steps. With the rapid acceleration in research and development activity we are now seeing, if companies have not already started along the path, they do indeed run the risk of being left behind if and when a breakthrough occurs.
Notes
[1] “Overview on Quantum Initiatives Worldwide — Update Mid 2021.” Qureca, July 2021, and ADL analysis.
[2] A logical qubit is a physical assembly of a much great number of physical qubits. Physical qubits are the quantum objects that exhibit the mathematical properties of a qubit, which could be based on, for example, atoms, ions, or photons.
[3] Random access memory (RAM) is the computer memory used to store data and code. Quantum memory is the equivalent function on a quantum computer.
[4] Temkin, Marina. “Investors Bet on the Technologically Unproven Field of Quantum Computing.” Pitchbook, 13 September 2021.
Appendix — Further observations on the second quantum revolution and developing technologies from Professor Alain Aspect
The founding fathers of quantum mechanics doubted that it would be possible to isolate, observe, and manipulate individual quantum objects such as electrons, ions, atoms, and photons. As Erwin Schrödinger expressed, writing in 1952: “We never experiment with just one electron or atom or (small) molecule. In thought experiments we sometimes assume that we do; this invariably entails ridiculous consequences … it is fair to state that we are not experimenting with single particles, any more than we can raise Ichthyosauria in the zoo.”[1] But starting in the 1970s, physicists developed new methods to experiment with single elementary quantum objects: electrons, ions, photons, atoms. And they developed new devices based on superconductors behaving as single quantum objects.
The recently developed quantum technologies that belong in the second quantum revolution rely on control and detection of single quantum objects, as well as on the ability to entangle them. Moreover, in all the schemes envisaged today, one must be able to identify and work exclusively with two states of each object, the result being a qubit evolving in a two-dimensional Hilbert space.
By making two qubits interact, one can entangle them. Experimental tests of Bell’s inequalities with photons demonstrated that entanglement does exist as described in the formalism of the theory, with its mysterious non-locality. These experiments were possible only because we could produce individual pairs of entangled photons and detect individually each photon of the pair.
As I mentioned in the Preface, it is likely that there will not be a single qubit better than others for all functions we want to realize. Take, for instance, a photon, whose states of polarization are described in a two-dimensional Hilbert space; it is almost ideal since it is insensitive to most perturbations, such as stray magnetic fields. But you cannot stop it, so in addition to such flying qubits, we also need static qubits that can store the quantum information until it is processed. At the moment, the best natural static qubits are ions and neutral atoms, which are quantum objects that are absolutely identical and obey elementary physics laws, so we can describe their behavior in detail. On the other hand, devices based on superconductors are artificial qubits produced by nanotechnologies, and one cannot be sure that they are all alike. Their qualities may vary depending on the fabrication process.
To evaluate the potential of the various qubits, a key feature to consider is scalability; in essence, the possibility to increase their number without deteriorating the quantum behaviors of the ensemble. The dimensionality of the ensemble of qubits obviously plays a role, and the scalability is better when the dimension is higher. Ion traps are linear, so the size of the system increases linearly with the number of qubits, although there are some prospects to develop 2D ion traps. Neutral atoms, either in optical lattices or in multiple tweezers, are naturally 2D, so the size increases as the square root of the number of qubits. For a number of several hundreds, this is a killer advantage. I do not know enough about superconducting devices, but it seems that their big advantage is the possibility to use the already much-developed techniques used for micro-electronics, so scaling is natural. One must be aware, however, that to achieve useful scaling, it must be accompanied by a simultaneous increase in the quality of the qubits. This is no small problem.
As a last point on elementary devices, I want to stress the importance of developing good quantum memories, able to store the states of qubits and to restitute them on demand. These quantum memories will be important not only as registers for quantum computers but also in quantum information transmission. For the latter, it would be very important to have quantum memories able to store the quantum state of photons. I think there are true market opportunities here, because many quantum technologies already demonstrated — such as QKD or quantum teleportation — will need quantum memories to be really useful. They will also be crucial for linking future quantum processors, or as an element of an optical quantum computer.
I am more optimistic regarding quantum simulators than universal quantum computers. Quantum simulators comprise an ensemble of qubits with interactions designed to produce an entangled system whose fundamental state is hard to compute with a classical high-powered computer. As mentioned in the Report, the first successes of such simulators have been about physics questions, but the ensemble of qubits could be prepared in a state that will evolve toward a steady state that represents the solution of a hard optimization problem, such as the traveling salesman problem.
This is only one of the many possibilities quantum simulators offer, and we will know soon if these hopes become a reality. Personally, I think that there is a good chance to obtain useful results, for instance regarding optimization of the electric grid. It seems also that interesting results may be expected in the quantum description of molecules of biological or medical interest. Moreover, the most advanced simulators of today are platforms that have the potential to be converted into universal quantum computers. Meanwhile, I want to stress that such simulators have already demonstrated a quantum advantage in the resolution of the Ising problem, which is a physics problem that has been open for decades.
Finally, in my opinion, true quantum advantage can be claimed only if it applies to a problem that was open before the quantum simulator was set, and for which one can explore a range of parameters. The Ising problem is such an example, and currently there is a race between the results obtained by experimentalists and those of the experts in classical computing, who develop approximations that allow them to obtain results in a reasonable time. Today’s simulators have already demonstrated a quantum advantage in the problem’s resolution. At the moment, the quantum simulator is ahead but classical computing catches up regularly. The good news is that their results coincide, which is a valuable cross check of the two approaches.
Cat qubit — Cat states are quantum superpositions of coherent qubit states with opposite phases. They have been postulated, notably by Alice & Bob and Amazon, as part of a novel approach to quantum error correction. They enable the creation of qubits that are natively flip-error-proof. Fault-tolerance is implemented using quantum error correction only for phase flips, which reduces the number of qubits required.
Decoherence — Quantum coherence refers to the ability of a quantum state to maintain its entanglement and superposition. Decoherence refers to the loss of coherence.
Entanglement — Quantum entanglement is the physical phenomenon that occurs when a group of particles is generated, interacts, or shares spatial proximity in a way such that the quantum state of each particle of the group cannot be described independently of the state of the others, including when the particles are separated by a large distance.
Quantum supremacy/quantum advantage — Quantum supremacy or quantum advantage refers to demonstrating that a programmable quantum device can solve a problem that no conventional computer can solve in any feasible amount of time (irrespective of the usefulness of the problem).
Quantum key distribution (QKD) — QKD is a secure communication method that implements a cryptographic protocol involving components of quantum mechanics. It enables two parties to produce a shared random secret key known only to them, which can then be used to encrypt and decrypt messages.
Superposition — The principle of quantum superposition states that any two (or more) quantum states can be added together (“superposed”) and the result will be another valid quantum state. Conversely, every quantum state can be represented as a sum of two or more other distinct states.
Quantum sensing — Quantum sensing refers to the design and engineering of quantum sources (e.g., entangled) and quantum measurements that can beat the performance of any conventional strategy in a number of technological applications, such as microscopy, positioning systems, communication technology, and electric and magnetic field sensors.
Quantum communications — Quantum communications refers to a wide range of usually photon-based telecommunications technologies at different levels of maturity that take advantage of the laws of quantum mechanics to enable, for example, cryptographic security.
Qubit — A qubit or quantum bit is the quantum version of the classic binary bit physically realized with a two-state device. A qubit is a two-state (or two-level) quantum-mechanical system. Physical examples include the spin of the electron in which the two levels can be taken as spin up and spin down; or the polarization of a single photon in which the two states can be taken to be the vertical polarization and the horizontal polarization.
Technology assessment
As part of this Report, we have assessed the seven key competing core qubit technologies in terms of maturity, pace, and disruption potential. Maturity is evaluated based of the established technology readiness level (TRL) scale set out in Figure 12. Pace and potential for disruption are assessed based on the framework in Table 2.
Superconducting
Superconducting is currently the most mature technology and is developing quickly. There are, however, many obstacles still to scale up.
Description — Ground stage designed by first Cooper pair box circuit produced in 1997 by Vincent Bouchiat, with the first demonstration achieved in 1999 by Yasunobu Nakamura and a first functioning version verified in 2002. A widely used qubit design with distinctive characteristics — at superconducting temperature electrons form an artificial atom with an energy level that can be controlled accurately. Three types of superconducting qubits exist: phase, flux, and charge qubits.
Key benefits — Enabling technologies already exist and the approach has good scalability in 2D architecture. Noise reduction techniques are constantly advancing. The technology is heavily backed by both major companies and large research institutions.
Key hurdles — Qubits currently suffer from a low coherence time and high noise levels, while qubit coupling is constrained. The technical complexity of the approach is high, requiring calibration and advanced cryogenic technology, and many electronic components with large-scale cabling for industrial applications.
R&D themes — Noise reduction is a major research theme to make superconducting qubits feasible for industrial applications. At the same time, cryogenic technology needs to advance to support technical complexity.
Key players — The main technology is being actively pursued by both public research institutes and large corporations, with major investments from both groups. Players include Alice & Bob, Amazon, D-Wave, Fujitsu, Google, IBM, Intel, IQM, QCI, and Rigetti, as well as physics labs in countries such as Austria, France, Germany, the Netherlands, Russia, and the US.
Maturity — TRL 4: Technology validated in limited form at lab scale.
Pace —
Disruption potential —
Electron spin
Electron spin has potential for good scalability due to miniaturized architecture and low error rates, but has only been demonstrated at small scale so far.
Description — Research began after the development of superconducting qubits and was first presented by Daniel Loss and David P. DiVincenzo in 1997. Based on the spin orientation of an electron secured in a semiconductor structure, it is a potential candidate for large-scale applications due to its miniaturized architecture and low error rates. It operates at higher working temperatures than superconducting (around 1 Kelvin/-272 Celsius). The qubit readout works via conventional electronics by measuring the electrical charge.
Key benefits — The qubit production process is similar to semiconductors. They display good scalability due to their small size, along with good quantum gate speed. The 1 Kelvin temperature requirement is less extreme than some other types and they have a 2D architecture.
Key hurdles — Currently, limited entanglement has been achieved, while qubit fidelity is average and qubit variability is currently unconfirmed. Manufacturing costs are high.
R&D themes — R&D is focusing on five variations of electron spins qubits (arsenic/gallium, silicon, germanium on silicon, atom ions implanted on silicon, and graphene structures trapping single electrons). R&D is also looking at “hot” qubits operating at higher temperatures.
Key players — Players include EeroQ, Equal1 Labs, Intel, Quantum Motion, as well as physics labs in countries such as Australia, France, Germany, Japan, the Netherlands, the UK, and the US.
Maturity — TRL 3: Proof of concept exists.
Pace —
Disruption potential —
Photon
Photon qubits have the advantage of room-temperature operation, but scalability challenges are significant and the technology is still relatively immature.
Description — Based on photons, exploiting their polarization or other physical characteristics, with single photons produced by lasers or diodes. While the development of model components started in the 1920s, the first QC applications began in the 1990s. In contrast to other qubits, photons have no mass and move at the speed of light, making them the only qubit type that can travel over long distances. They are stable qubits at ambient temperatures and can be applied in quantum computation, simulation, communication, cryptography, and sensing. However, they are not yet scalable due to the probabilistic character of quantum gates and require the implementation of specific computing techniques.
Key benefits — Stable qubits that work at room temperature. New manufacturing technology enables scalability or can be adapted from semiconductor processes. They are easier to scale due to communication between qubits and quantum communication.
Key hurdles — Scalability is currently hampered by the inefficiency of photon sources and quantum gates. Information cannot be stored on photons, leading to delays. Some cooling of enabling technologies is required, however temperatures are not critical. Quantum advantage is theoretically existent but practically not proven.
R&D themes — Improvement of manufacturing processes, research into multiple photon sources. Research into a wide field of applications and using them as an enabler for other qubits. In December 2020, a photonic quantum computer with 76 photons was demonstrated.
Key players — Players include ORCA, PsiQuantum, Quandela, and Xanadu as well as physics labs in countries such as Australia, Austria, China, France, Germany, Italy, Japan, the UK, and the US.
Maturity — TRL 3: Proof of concept exists.
Pace —
Disruption potential —
Trapped ion
Trapped-ion qubits show the best stability and error rates so far, but thus far they have been hard to scale beyond 32 qubits.
Description — The idea was first developed in the 1950s by Wolfgang Paul, later a Nobel Prize physicist, but it took until 1995 before they were first tested in a lab. Positive ionized atoms from alkaline metals (e.g., beryllium, magnesium, strontium, barium, and particularly calcium) are trapped in a limited space via magnets or electrodes close to each other. Storage is in the stable electronic states of each ion, and information proceeds through the motion of the ions. Lasers are used for coupling between the qubit states, either in single operations or for entanglement.
Key benefits — No calibration is required due to identical ions. Good stability of qubits, with best-in-class error rates and long coherence time. The entanglement of qubits on a 1D architecture and between ions and photons is possible and the process works at higher temperatures (4-10 Kelvin).
Key hurdles — Questionable scalability beyond 50 qubits, while relatively slow quantum gates could limit applicability for deep algorithms. Trapped ions need to be maintained in a vacuum, adding to complexity.
R&D themes — Research around easy handling of ions compared to other qubit types.
Key players — Players include Alpine Quantum Technologies, EleQtron, IonQ, Honeywell/Quantinuum, Oxford Ionics, as well as physics labs in countries such as Australia, Austria, Canada, China, Denmark, Finland, Germany, India, Israel, Japan, the Netherlands, Singapore, Switzerland, the UK, and the US.
Maturity — TRL 3: Proof of concept exists.
Pace —
Disruption potential —
Cold atom
Cold atom qubits have acceptable error rates and long coherence times, but potential applicability has been mainly for simulation rather than gate-based computing.
Description — Based on cold atoms of elements such as rubidium held in position with lasers rather than electrodes, cold atoms can also be used in a Rydberg configuration as a source for photons in nonlinear optics. They offer acceptable error rates and a long coherence time. Deploying required lasers and optical control technology is challenging for scaling beyond ~1,000 qubits. Overall, they are better adapted to simulation rather than to universal gate–based computing.
Key benefits — Acceptable error rates, with a long coherence time. As atoms are identical, they do not require calibration. No integrated circuits or advanced machinery are needed, while trapped ion qubit tools can also be used for cold atom readouts. They are focused most heavily on simulation use cases.
Key hurdles — Quantum gate error rates are acceptable, but not as strong as some other approaches. There is also cross talk between qubits and enabling technologies, such as lasers, and optics are currently not readily scalable.
R&D themes — Research into a wide field of applications besides QC, including cryptography, simulations, atomic clocks, and quantum memory.
Key players — Players include Atom Computing, BraneCell, ColdQuanta, PASQAL, and QuEra Computing as well as physics labs in countries such as Austria, France, Germany, the UK, and the US.
Maturity — TRL 3: Proof of concept exists.
Pace —
Disruption potential —
Nitrogen vacancy (NV) centers
NV centers show good stability and simple cryogeny, but their main applicability so far is magnetometry and sensing rather than QC.
Description — NV centers are based on the control of electron spins trapped in artificial defects of crystalline carbon structures. Initial work took place in the 1970s, but only became valid for QC applications in the early 1990s. Qubit readout is via a laser, which measures the fluorescence of the cavity, while microwaves or magnetic fields control the electron spin. A temperature of 4 Kelvins is used, making cryogeny requirements less onerous. The main application is for magnetometry and sensing rather than QC.
Key benefits — Long coherence time and requires simpler cryogenic equipment. Qubits have a strong and stable diamond architecture and can converge with other qubit types to create quantum memory. Additionally, they can be integrated into optical quantum networks.
Key hurdles — Currently, research activity is low and the practical applications of NV centers are limited for QC. Most applications lie in the field of quantum sensing. Controlling qubits via lasers is complex.
R&D themes — The NV centers qubits research field is developing in three areas: toward quantum sensing, use in precise magnetometers, and to leverage for quantum memories.
Key players — Players include Quantum Brilliance and Turing, as well as physics labs in countries including Australia, China, France, Germany, Japan, the Netherlands, and the US.
Maturity — TRL 2: No proof of concept so far.
Pace —
Disruption potential —
Topological
Topological qubits have high potential in theory but have not been demonstrated in practice.
Description — First conceived in 1997 by Microsoft researcher Alexei Kitaev, topological qubits offer a more robust alternative to superconducting qubits. They are harder to knock out of superposition, requiring lower error correction. They are based on using anyon particles and the Majorana fermion variant of anyons as qubits. Anyons are “quasi-particles” (i.e., particle representation models that describe the state of electron clouds around atoms in the superconducting regime). To date, no Majorana fermion qubit has been successfully demonstrated. However, in February 2020, John Preskill (the father of the notions of quantum supremacy and NISQ) predicted that, by 2030, we will be able to demonstrate two entangled topological qubits.
Key benefits — These are stable qubits, with a long coherence time, good scalability, and low error correction requirements. Their high gate speed enables the processing of long and complex algorithms.
Key hurdles — No Majorana fermion qubit has yet been demonstrated, making them currently purely theoretical. Topological qubit programming requires an additional software layer compared to alternative approaches. They require low cryogenic temperatures similar to superconducting qubits. Microsoft is the only potential vendor, although IBM is investigating the field.
R&D themes — Fermion particles are still the subject of basic research by various physical laboratories. In 2018, a Microsoft team predicted a fermion-based machine by 2023, but this is now highly unlikely.
Key players — Players include Microsoft as well as physics labs in countries such as China, Denmark, Finland, France, the Netherlands, and the US.
Maturity — TRL 2: No proof of concept so far.
Pace —
Disruption potential —
Appendix Note
[1] Schrödinger, Erwin. “Are There Quantum Jumps? Part II.” British Journal for the Philosophy of Science, Vol. 3, No. 11, 1952.
DOWNLOAD THE FULL REPORT
69 min read
Unleashing the business potential of quantum computing
"If quantum mechanics hasn’t profoundly shocked you — you haven’t understood it yet." — Niels Bohr
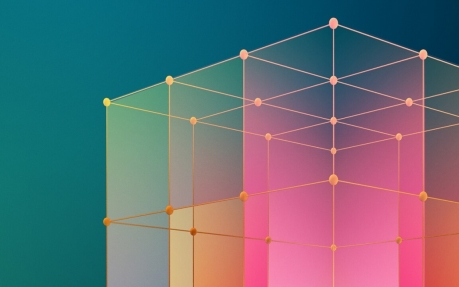
DATE
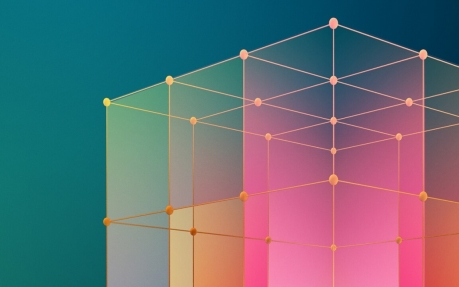
Executive Summary
Most businesses are already aware of the potentially disruptive impact that quantum computing (QC) will have if it develops successfully and becomes commercially available. It promises to transform business through its ability to tackle so-called intractable problems with exponentially increasing complexity that currently are beyond the reach of conventional high-performance computers. These types of problems can be grouped broadly into four categories: simulation, optimization, machine learning (ML), and cryptography. The use cases for business across the first three categories are almost endless, from fully digitalized drug discovery and new materials development through to logistics, supply chain management, and portfolio optimization.
Yet QC technology is extremely complex and hard for even the smartest generalist to properly understand. There is a constant stream of media stories and announcements about new developments and claimed breakthroughs. Seeing clearly through the “fog” is difficult.
In this Report from Blue Shift by Arthur D. Little (ADL), we have aimed to provide a clear overview of the state of play for business readers not familiar with the domain, sharing not just potential use cases and applications, but also technology development, including hardware and software, and highlighting key players active in the QC ecosystem. We also give our perspective on the all-important question of the likelihood and possible timescales for commercialization of QC.
Today, QC has not yet progressed beyond lab scale, even though it is integrated in some commercial offerings. There are still huge technical and engineering challenges to overcome. A fault-tolerant, large-scale, general-purpose device able to provide both technical and commercial advantage over conventional high-performance computers is still many years away, and there is still no certainty that it will be achieved. If and when quantum processing power becomes available, it will be a complement to conventional computing; it will not replace it.
However, recently we have seen a rapid acceleration of investment and activity in QC development, which will certainly continue over the next few years. Declared public investment is in excess of US $25 billion, with over 50 venture capital deals in 2021. There are more than 20 new prototype devices planned in published vendor roadmaps between now and 2030. We expect that there will be some progress in achieving so-called quantum advantage in specific limited applications during the coming years, possibly involving quantum simulators and/or hybrid solutions involving a combination of conventional and quantum processing. There could be an early breakthrough, but of course this is impossible to forecast with any certainty.
Given the current state of play, we recommend that companies that have not already done so take steps now to ensure they are not left behind. The QC learning slope is steep. It will not be possible, especially in the earlier stages of commercialization, for inexperienced users simply to do nothing until the technology matures, buy access to quantum processing power, and immediately expect to make transformational changes in their business operations. Furthermore, as the technology starts to mature, the current open exchange of intelligence and data across the global QC ecosystem may stop, driven by both commercial and geopolitical pressures. Companies that have no internal capability could be especially disadvantaged.
In this Report, we set out four steps companies should take to prepare for the future, including exploring applicability, monitoring technical developments, engaging with the ecosystem, and building knowledge and capability. It is only through taking such steps that companies will be able to see clearly through the fog and avoid being left behind when QC properly takes off.
Preface — The second quantum revolution, from concepts to technologies
There are different kinds of prefaces. Mine is from someone who is not an expert on the major aim of this Report: describing the business perspectives in quantum computing. I am an academic who has all his life worked on basic research, and my expertise in business perspective is negligible. But I am thrilled by the perspective that the purely fundamental research I did long ago on entanglement is now at the core of the second quantum revolution, including its technological sector. Therefore, I will not comment here about statements you will find within the Report. Rather, I would like to share some of my thoughts about the second quantum revolution and a few of my expectations, which stem more from intuition than cold business analysis. For those who already have some understanding of quantum computing technologies, I have provided more background and detail on my observations in the Appendix of this Report.
The first quantum revolution, whose formalism was developed in the first decades of the 20th century, was based on the concept of wave-particle duality. While the concept was expressed in relation to individual quantum particles, it was in fact applied to large ensembles of particles, such as photons in a light beam or electrons in an electric current. The measurements then bear on macroscopic quantities, such as light flux or electric current, which are classical in character but result from averaging the results of quantum calculations. Quantum physics was the key to understanding how electric current behaves in different materials, such as semiconductors, or how light is emitted by matter. Fantastic applications resulted from that understanding, including transistors and integrated circuits as well as lasers. These applications are at the root of the information and communication society. They have transformed our society as much as the heat engine did in the 19th century.
The second quantum revolution, which started in the 1980s, is based first on the understanding that entanglement, a quantum property of two or more particles, is yet more revolutionary than wave-particle duality for a single particle. To make a long story short, the trajectory of a particle, as well as the propagation of a wave, are notions that we describe without difficulty in our ordinary space time. The conceptual difficulty is to apply both notions to the same object. But two entangled objects can only be described consistently in an abstract mathematical space, which is the tensor product of the spaces of each particle. If one insists on describing the situation in our ordinary space time, then one must face surprising behaviors such as quantum non-locality; in other words, the fact that in our space time there seems to be an instantaneous interaction between spatially separated quantum objects.
The other ingredient of the second quantum revolution, crucial for the development of quantum technologies, is the experimental ability to isolate, observe, and manipulate individual quantum objects, such as electrons, ions, atoms, and photons. With such individual quantum objects, one can develop quantum bits — or qubits — which are basic elements of quantum information, much the same as ordinary bits are basic elements of classical information.
Managers who are not knowledgeable in quantum science often ask the question: “What is the best qubit to invest in massively?” I have been asked that question many times, including by European Union program deciders. The answer is: “We do not know, and it is likely that there will not be a single qubit better than the others for all the functions we want to realize.”
This Report scans the solutions explored in quantum computing and correctly distinguishes the various kinds of devices that are too often confused. Universal quantum computers may or may not exist in the future, but a minimum development time of one decade is rather on the optimistic side. So investors must be patient and open to high-risk, long-term investments. I am more optimistic regarding quantum simulators — an assembly of qubits with interactions designed to produce an entangled system whose fundamental state is hard to compute with classical high-performance computing.
Will the second quantum revolution, which is primarily a conceptual revolution, produce a technological revolution as disruptive as the first quantum revolution? I have no idea, and I doubt that anybody has a trustable answer to that question. But I have no doubt that some quantum technologies will be useful and provoke a revolution in some niches. For instance, I believe quantum random number generators based on a single photon falling on a beam splitter should overcome all other random number generators when security is at stake.
Quantum key distribution (QKD) based on entangled photons permits secure communication based on the fundamental laws of quantum physics rather than on relying on the hypothesis that an adversary has about the same level of technological or mathematical sophistication. QKD would be useful in diplomacy, where the disclosure of confidential information after a few years may cause serious problems. Quantum simulators also may be the solution to important problems such as the optimization of the electricity grid.
And it should be emphasized that quantum advantage might be something beyond making calculations faster. For example, what if a quantum simulator or a quantum computer was delivering the same result as a classical computer in a comparable time but with a much lower energy cost?
— Alain Aspect, Nobel Prize in Physics 2022
Preamble: Quantum computers are quantum
John Gribbin’s In Search of Schrödinger’s Cat is one of the first books I read on the history of physics. That was in high school, almost 30 years ago. It was also through Gribbin’s book that I first dipped my toe into quantum physics — notably with the famous analogy of Schrödinger’s cat, which is both dead and alive. The analogy is frequently used to explain certain disturbing behaviors of matter at atomic scales, such as the fact that an electron can simultaneously be in two places at the same time. Quantum physics is magical!
It wasn’t until years later, when I was doing my PhD in theoretical and numerical physics, that I heard about the quantum computer — almost 20 years ago. The quantum computer was also magical, and all the more so as popularized in the media, where QC is generally described in contrast to the classic computer, which uses bits (zeros and ones) that are combined using logic gates to perform operations. Instead, the quantum computer uses qubits. These qubits can have several values at the same time, allowing us to perform many operations at the same time. The complexity of the underlying physics and mathematics is usually obscured by the qubit’s exotic and magical properties.
In short, the subject has remained extremely mysterious to me, as is true for most people, I suppose.
The mission of Blue Shift by ADL — the forward-looking, techno-strategic think tank that I have the honor of leading — is to help executives from our industrial ecosystem to better see through the technological fog and thus better anticipate business and societal disruptions. One of the hallmarks of Blue Shift is drawing on the sometimes provocative perspectives of executives, experts, philosophers, and artists to create fresh thinking and illuminate some unexpected insights on technologies from within and beyond business. As an example, we share in this Report contributions from two artists that highlight some unexpected insights from outside the business realm.
In developing this Report, our team has been on a journey to the edge of the “Quantum Realm.” And if I had to summarize what we discovered, I would say:
The quantum computer is quantum: it does not exist, and yet it exists!
This summarizes well one of our key messages: even though full-scale quantum computers do not yet exist to solve real business problems, many prototypes do exist, and business disruptions are to be anticipated in the coming years. And because the topic is complex, the learning curve is very steep. Most companies should therefore start diving into it today!
But before diving in, I would like to share two anagrams I found for “ordinateur quantique” (quantum computer):
tout indique: “arnaquer” (everything indicates: “rip off”)
rare, quoiqu’ inatendu (rare, though unexpected)
They may sound a little pessimistic but, as always, anagrams move in mysterious ways. I wonder what noted French physicist and philosopher of science Etienne Klein would think of them. What do you think?
— Dr. Albert Meige
1
What is quantum computing?
QC is currently attracting billions of dollars in investment, both private and public, as well as extensive media attention. For those businesses not already engaged in the ecosystems that are researching and developing QC technology, navigating the future is challenging. The field is difficult to understand technically, even for smart generalists, and there are many announcements and claims of breakthroughs in the media that can be misleading.
The aim of this Report is to provide an overview of the state of play for business readers not familiar with the domain and to address the following questions:
- What is the current status of technology development in QC, including potential impacts and timescales?
- What are the potential use cases and applications for business so far?
- What should businesses be doing now to prepare for what is to come?
This Report focuses particularly on the state of play today. It covers QC specifically and makes only passing reference to other quantum technologies, such as quantum sensing and quantum communications.
Inevitably, the analysis and conclusions in this Report are at a high level given the huge scale and complexity of the QC domain.
Understanding the basics
QC is one of today’s most talked-about technologies. It is also one of the least understood, in part because of the complexity of quantum mechanics itself. A quantum computer operates in a completely different manner to a conventional computer, requiring a deep knowledge of physics to really understand how it works.
Rather than using bits, which can have a value of 0 or 1, quantum computers use qubits, which can be both 0 and 1 simultaneously. This phenomenon is known as superposition (see Appendix for a glossary of QC terms), and the value of its readout is probabilistic rather than deterministic. When linking multiple qubits through the process of quantum entanglement, every added qubit theoretically doubles the processing power. Placing a number of entangled qubits in an array and applying gate logic operations to them allows computing power to be harnessed. Figure 1 provides a very high-level schematic of the principles behind how a quantum computer operates.
This exponential growth in calculation capacity as qubits are added means that quantum computers can solve certain types of problems beyond the power of conventional computers. These types of problems are referred to as “intractable problems,” and they involve complex systems with multiple elements, variables, and interactions, where the number of potential outcomes scales up exponentially. There are many such intractable problems in the natural and human worlds, such as optimizing logistics operations between multiple nodes, or predicting weather patterns. In general, the applications can be split into four categories:
- Simulation. Complex physical and molecular simulations, such as so-called in-silico simulations, which could enable digital development of new materials, chemical compounds, and drugs without the need for lab experimentation.
- Optimization. Optimization of complex systems, such as logistics planning, distribution operations, energy grid systems, financial systems, telecoms networks, and many others.
- ML. Artificial intelligence (AI) and ML applications require very high processing power, stretching the limits of conventional computing. The applicability is large but the “quantum advantage” for these types of applications is much less obvious in this field, particularly since ML usually involves training with a lot of data.
- Cryptography. A quantum algorithm has already been developed to conduct rapid integer factoring, which poses a disruptive threat to conventional cryptographic protection approaches for IT, finance, and so on. Quantum technologies will be critically important for future cryptographic solutions. Quantum cryptography can be used to secure secret key distribution but does not rely on a quantum computer. Post-quantum cryptography is a new breed of classical key distribution that is resilient to quantum computer–based key breakings.
Quantum computers are unlikely to ever replace conventional computers for the majority of applications. However, assuming they reach maturity, they will still have a transformative effect on our ability to solve these types of intractable problems, either stand-alone or in combination with conventional computing systems. We will consider applications further in the chapter “Understanding QC applications across sectors.”
Navigating the QC hype
The huge potential of quantum computers, together with a recent acceleration of development activity and announcements of large-scale investments, has ensured a wave of interest, especially over the last few years. Countries, technology players, academia, and startups have all announced QC plans and strategies, with declared public investment alone in excess of $25 billion.[1]
All this noise may lead casual observers to believe that quantum computers will appear, fully working, in a short time frame and available for use across the board, which is certainly not the case. This misunderstanding is due to three issues:
- The difficulty of understanding QC concepts, which makes it hard for nonspecialists to properly analyze current development activity and announcements.
- The sheer volume of announcements of “breakthroughs” from players. Although the main players declare that they are anxious to avoid overhype, to avoid a “quantum winter” or “trough of disillusionment” if progress slows down, they nevertheless need to attract investment and position themselves favorably, and this inevitably leads to breakthrough claims being given a positive spin.
- Linked to the difficulty of understanding the complexity of QC, many media news stories are inaccurate or oversimplified, giving a false impression of current progress.
What is true is that the coming few years and beyond promise many new prototypes and developments that could provide a breakthrough (see also Chapter 4, “Understanding QC software algorithms”). That said, there are still some major challenges to be addressed.
The challenges to QC emergence
Unlocking the potential power of QC will require overcoming three large-scale challenges in the areas of physics, engineering, and algorithms. Solving these issues is not easy and in many cases will require significant work at a fundamental level.
Physics challenges
Several theoretical physics challenges remain. Currently, it is difficult to create scalable quantum computers with low enough error rates, due to the problem of noise affecting qubit stability, causing a phenomenon called “decoherence.” Errors in each gate operation multiply up, so a 0.005% error rate can quickly rise to more than 50% with multiple gate operations. This greatly limits the ability of quantum computers to solve complex algorithms. Physical qubits can be combined to provide error correction, although very large numbers of physical qubits are needed — currently at a ratio of up to 1,000 physical qubits for each logical qubit.[2] Finally, some required concepts, such as “quantum memory” (the equivalent of RAM on a conventional computer)[3] do not yet exist.
Engineering challenges
Building quantum computers is complicated and costly. To begin, to overcome decoherence qubits require isolation, meaning they need very low temperatures, delivered through expensive engineering solutions. These enabling technologies are currently complex and require significant investment (e.g., superconducting cabling, microwave generation, and readout systems).
Algorithm challenges
Ensuring that quantum computers actually deliver operationally is also a hurdle. Harnessing the power of quantum computes requires specific algorithms. These algorithms can be tested on quantum computing emulators running on conventional computers or on the limited quantum computers now available. However, this testing is restricted by the constraints of conventional computing power, making it difficult to show concrete, real-life applications that solve otherwise intractable problems.
Additionally, there are practical problems to overcome in achieving true exponential speedup versus conventional computing, such as the difficulty of data preparation and loading and the real costs of quantum error correction. Lack of available QC hardware of sufficient scale means that practical testing of algorithms is often impossible.
Nevertheless, despite these challenges and concerns over industry hype, businesses cannot afford to ignore QC technology. Otherwise, they risk being left behind if and when a breakthrough occurs, since the adoption rate post-breakthrough could be extremely rapid, and the learning curve is daunting for those with little or no knowledge and experience. QC has the potential to transform operations for specific businesses in specific areas, delivering enormous potential competitive advantage.
In the next two chapters, we will focus on the state of play of development on both the hardware and software sides, and how some of the major challenges are being addressed.
2
Understanding QC hardware
QC architecture requires extremely complex and expensive hardware, both for the processors themselves and for their enabling infrastructure. Many of these technologies are still immature, unproven, or even at a theoretical stage. However, given the current wave of investment and focus on QC, the likelihood of a commercial-scale machine entering the market in the next five to 15 years has significantly increased.
In this chapter, we explore the categories of quantum computer that exist today, the hardware side of a quantum computer, and the multiple approaches being pursued for generating qubits suitable for use in computer processing.
Various computing paradigms
Conventional computer architecture is based on a central processing unit with short-term memory for rapid data access. A quantum computer’s architecture is fundamentally different. There are several categories of quantum computer (or several computing paradigms), as illustrated in Figure 2.
Starting on the left-hand side of Figure 2, quantum emulators are conventional supercomputers that are used to test, debug, and verify quantum algorithms. They are not quantum computers themselves but are still an important tool in the development path.
Next are two categories of analog quantum computer: annealers and simulators. Annealers use low-quality qubits that are set to converge toward the solution, often the result of a search for minimal energy. The main applicability for annealers is for optimization and simulation problems, although there are major challenges with scalability to tackle complex algorithms. The vendor D-Wave leads work in this category, and quantum annealers are already accessible to users.
Quantum simulators refer to a broader category of analog device designed to model complex quantum mechanical systems. Up to now, they have been applied mainly to address physics challenges in the laboratory, as they have only been suitable for addressing certain types of problem. No major commercial vendors are involved in the development of quantum simulators. However, for certain specific optimization and simulation problems, they may actually achieve quantum advantage sooner than gate-based computers.
Universal gate–based quantum computers, which use logic gates like classical computers, have the broadest general-purpose application. They are therefore the primary focus for investment and development. For the remainder of this Report, we focus on this type of quantum computers.
The overall aim is to develop large-scale quantum computers (LSQC). These are fault-tolerant devices with high levels of error correction that can tackle much more complex algorithms at scale by having a high number of stable qubits. However, these are not expected to be available for at least a decade, meaning that current roadmaps focus on so-called noisy intermediate scale quantum (NISQ) devices. As these have a limited number of noisy (as opposed to stable) qubits and no error correction, they only outperform supercomputers for certain algorithms that do not require large numbers of instructions.
There are also hybrid QC concepts in which the quantum computer is used as an accelerator to a conventional supercomputer. These are described in more detail in the section “Taking a hybrid approach.”
Inside the quantum computer — how it operates
Gate-based quantum computers, sometimes referred to as universal quantum computers, use logic gates to perform calculations. This is analogous to the AND/OR/NOT gates in conventional computers, although quantum gates operate differently.
In a typical universal gate–based QC setup, a conventional computer acts as a host to control QC operations, providing its input and output interface, using control and readout electronics. The seven key elements of a basic gate-based quantum computer using superconducting qubits (shown in Figure 3) are as follows:
- Qubit register — collections of qubits that store the information manipulated in the computer. To make a parallel with classical computing, this could be considered as memory, but with the big difference that processing is also done directly in the quantum register.
- Quantum gate controller — physical devices that act on the quantum register qubits, both to initialize them and to perform quantum gates on them, according to the algorithms to be executed. They can also be used to manage error correction codes.
- Error correction — implemented with special code operating on a large number of logical qubits (see “Algorithm challenges,” above, for background on logical and physical qubits). As of 2021, no quantum computer is large enough to accommodate logical qubits, as the ratio of physical to logical qubits exceeds the maximum number of qubits currently available. This is a major challenge for scale-up.
- Qubit measurement — the process used to obtain the result at the end of the execution of an algorithm’s quantum gates and to evaluate error syndromes during quantum error correction. This cycle of initialization, calculation, and measurement is usually applied several times to evaluate an algorithm result, and the result is then averaged to a value between 0 and 1 for each qubit. The values read by the physical reading devices are then converted into digital values and transmitted to the conventional computer.
- The quantum chipset — normally includes registers, gate controls, and measuring devices. It is fed by microwaves, lasers, and the like to manipulate the qubits, depending on their type. The chipset for superconducting and electron spin qubits is small, measuring just a few square centimeters in size. It is usually integrated in a purified oxygen-free package.
- Qubit control electronics — drive the physical devices used to initialize, modify, and read the qubit status. In superconducting qubits, quantum gates are activated with microwave generators.
- Cryogeny — keeps the inside of the computer at a temperature close to absolute zero for most qubit types. Room temperature operation is a key development objective, but practical architectures for this are not yet operational.
A range of qubit approaches
Creating qubits and keeping them stable is an enormous technical challenge. They are inherently unstable and tend to “decohere,” which destroys the properties of superposition and entanglement, removing their ability to provide computing power. Error correction is possible, but this requires many additional physical qubits, as described earlier.
There are seven major approaches to creating qubits currently under research and development, with varying degrees of maturity. Each approach uses a different physical phenomenon to create a qubit, based on a variety of particles from superconducting electrons through to ions, photons, and atoms. These are outlined in Figure 4, with further details and explanation of technical maturity included in the Appendix:
- Superconducting qubits are based on electrons at superconducting temperatures (i.e., close to absolute zero). Under such conditions, electrons collectively behave like a single particle with an energy level that can be controlled accurately.
- Electron spin qubits are based on the spin orientation of an individual electron trapped in a semiconductor potential well.
- Photons can be used as qubits, exploiting their polarization orother physical characteristics.
- Trapped ion qubits are based on ionized atoms electromagnetically trapped in a limited space and controlled by lasers or microwaves.
- Cold atom qubits are based on cold atoms held in position of vacuum with lasers.
- Nitrogen vacancy (NV) centers are based on the control of electron spins trapped in artificial defects of crystalline carbon structures.
- Topological qubits are based on so-called anyon particles, which are “quasi-particles” (i.e., particle representation models that describe the state of electron clouds around atoms) in the superconducting regime. The concept involves engineering their topology to provide additional resistance to interference (there is no working prototype so far).
In addition, so-called Flying Electron qubits, based on electron transport circulating on wave guide nanostructures built on semi-conductor circuits, are being pursued at the basic research level only, with no prototypes or vendors involved at this stage.
Currently, superconducting technology is the most mature approach for creating qubits and consequently seems likely to become commercially available for practical use in the next decade. However, at this early stage in QC development and with the enormous complexity involved, it is too early to pick a winner. Other technologies could achieve breakthroughs and draw level with — or overtake — superconducting, meaning it is vital to keep a constant eye on R&D progress. Each technology has its own benefits and drawbacks. For example, superconducting technology has good scalability, but noise levels are high, while cold atom technology has longer qubit coherence times, but there are challenges in scalability of the required enabling technologies, and applicability has mainly been for simulators thus far. Photon technology has the advantage of room-temperature operation, but also has scalability challenges. Trapped-ion technology has achieved the best stability and error rates, but has not yet been scaled beyond 32 qubits. Electron spin has the potential for good scalability, leveraging existing silicon semiconductor manufacturing expertise, but has only been demonstrated at small scale and manufacturing costs could be high.
Complex enabling infrastructure
As explained above, ensuring that qubits remain stable is a major technical challenge. Most, but not all, approaches require very low operating temperatures (close to absolute zero). This means that supporting infrastructure is usually complex and costly, including equipment such as cryogenic refrigeration units, superconducting cables with complicated wiring, microwave generation and readout systems, low-temperature thermometers, and lasers, as described in Table 1.
The state of hardware research
Despite the excitement, none of the approaches explained above has matured beyond being demonstrated at a proof-of-concept stage at lab scale. However, companies have published extensive roadmaps for creating new prototype quantum computers, with more than 20 devices promised in the run-up to 2030 (see Figure 5). Many timescales seem overly optimistic given current technical challenges and uncertainties, although breakthrough innovations could change this. Instead, it is perhaps more realistic to assume that the milestone of creating a fault-tolerant LSQC will occur between 2030 and 2040.
Qubit numbers quoted in vendor roadmaps should be interpreted carefully. Generally, vendors quote numbers of physical qubits. However, the relationship between physical qubits and logical or “usable” qubits that take into account error correction varies considerably. For example, IonQ claims a much lower ratio of physical to logical qubits than other vendors. It is also important to be aware that development is not just about making devices with increasing numbers of qubits. It is also about addressing a range of technical and engineering challenges in both the core and enabling technologies.
The challenges of scaling up
As we mentioned, achieving the necessary scale-up for commercial operation is one of the greatest challenges developers face. As well as aiming to make devices with ever-increasing numbers of qubits as shown in the roadmap, it is also worth mentioning the potential of networked quantum computers, which is another area of intensive research. In theory, if we were able via the cloud to seamlessly access a network of quantum computers to perform a computing task, this could be another way to achieve scale (i.e., scale out rather than scale up). This could also, in theory, enable different types of quantum computer to perform different tasks for which they are best suited (e.g., combining superconducting with cold atom). This would naturally also include conventional high-performance computers (HPCs) working in combination in a hybrid mode. There are, however, still major technical challenges to achieving such a network in practice (e.g., in terms of interconnectors and repeaters). The existence of new standards and specifications for interoperability would also be a key prerequisite.
Interlude #1 — Quantum chandeliers
In the collective imagination, the quantum computer is a machine from science fiction, with mysterious ways of working and extraordinary powers.
The illustration is composed as a triptych. In the center are individuals dehumanized by quantum supremacy, which appears like a dream — or a nightmare. On either side, quantum chandeliers remind us that this is a machine. It is not a dream but an imminent technological reality. The quantum computer is no longer just a fad fed by fantasies from popular culture. It is a promising, heavily funded research project.
— Samuel Babinet, artist
3
Understanding QC software algorithms
Just as with conventional computers, quantum machines require software to run on top of their hardware to generate results. However, gate-based quantum computers require the use of quantum algorithms. These use very different concepts than traditional programming, making their creation a specialist field.
Research into quantum algorithms can, to some extent, be uncoupled from quantum hardware and run on conventional computers acting as emulators. This means that progress to date on algorithms has preceded hardware development. Mathematicians have been able to work since the mid- and late 1980s on creating algorithms for quantum computers and emulators. This led to the first quantum algorithms that were published in the early 1990s. Today there may be more than 450 known quantum algorithms in existence, and constant new activity adds to this total.
The categories and uses of quantum algorithms
Forming the basis of all applications currently being considered are six broad categories of quantum algorithms, based on their use case:
- Search algorithms that speed up the search for structured or unstructured elements in a database or big data implementations.
- Complex optimization algorithms find solutions to combinatorial optimization problems, such as route or supply chain optimization, financial portfolio optimization, and insurance risk assessment.
- Quantum ML aims to improve ML and deep learning models in areas such as autonomous driving, customer segmentation, and the automatic detection of diseases.
- Quantum physics simulations, covering the optimization of inorganic/organic chemical processes, biochemical process simulation, and the design of new materials.
- Biological molecule simulation, for drug development and atom/molecule simulation.
- Cryptography algorithms that can break current encryption standards but also provide the ability to create new, stronger encryption protection.
Limitations to current algorithms
Being able to speed up quantum algorithms is crucial to proving that they can solve intractable problems and outperform conventional computers. However, while quantum algorithms are available now, their application remains practically limited by hardware considerations. Factors such as gate dependency, the number of times the algorithm must be run (the shot count), the number of qubits available, data preparation time, and error correction all impact speed, and therefore results. Data preparation is a particular, often-overlooked issue as data loading can have a significant time cost, currently making QC inadequate when carrying out big data computations.
All of this means that demonstrating speedup compared to conventional algorithms is not straightforward. This was highlighted by Google’s 2019 announcement in which the company famously announced the achievement of “quantum supremacy” (i.e., solving a problem faster than a conventional computer could in any feasible amount of time). However, the chosen task was difficult to compare with any known conventional algorithm, and since then others have managed to solve the problem relatively fast (less than a day) and with fewer errors using conventional computing methods.
Current hardware constraints mean that in many cases relatively simple algorithms can be run, but true benefits will require fault-tolerant, large-scale quantum computers that are unlikely to be ready until the 2030s at the earliest. For example, emerging NISQ computers cannot run “deep” algorithms due to error rates, meaning they can only use algorithms that chain together a small number of quantum gates, limiting their application.
Taking a hybrid approach
In the short term, hybrid algorithms provide a promising, alternative approach to achieving quantum advantage. As the name suggests, these combine conventional and quantum approaches, with the computation broken down into a smaller quantum component and a larger conventional computation component. A typical hybrid setup involves loop iteration between the conventional and quantum processing units (see Figure 6).
Essentially, this hybrid setup means that the quantum computer is focused on the part of the computation that plays to the strengths of current quantum machines, while the conventional machine handles everything else. This approach accelerates performance and potentially overcomes the limitations of NISQs, whose error rates limit their practical processing capacity, enabling applications to scale. Hybrid algorithms are not restricted to certain qubit types and are claimed already to be feasible on machines of between 20 and 1,000 qubits processing power. However, tight design integration between the different parts of the hybrid quantum computer is required if each element is to perform effectively.
There are many current algorithm providers, for example:
- Avanetix — supply chain issues in automotive and logistics.
- Groovenauts — retail planning optimization and others.
- AI — combines ML with quantum algorithms to simulate organic chemistry and design enzymes, peptides, and proteins.
4
Key players and activities
The last few years have seen a major acceleration of investment in QC from players across the ecosystem, including countries, research institutions, major technology companies, and startups. Venture capital funding increased by 50% between 2020 and 2021, reaching over $1 billion.[4] The landscape is very extensive, as Figure 7 illustrates.
Each group of players has its own motivations for quantum research and is targeting distinct positions in the ecosystem. Many players are aligned with only one or two of the core qubit technologies.
Government-level research
Countries see building strengths in QC as delivering a strategic and technical advantage. Not only does it underpin specific, highly complex use cases, but QC also has significant defense and security implications. For example, current public cryptographic keys are highly vulnerable to be cracked through QC algorithms, meaning that creating new capabilities is vital for protecting national infrastructure (and potentially disrupting the infrastructure of rivals).
Consequently, more than 20 countries have significant QC R&D programs, ranging from New Zealand to Singapore (see Figure 8). R&D activity is distributed globally, with strengths across US, Europe, China, and the rest of Asia.
The US remains the leading country in quantum development, through its combination of high public investment in basic research, global tech giants, and a well-funded startup ecosystem. China is aiming to leapfrog America and has claimed it will invest $10 billion in the period up to 2030, although this total is open to question. China also suffers from a current lack of basic research strengths. Overall, it can be difficult to correctly measure exact government investment given differences in splitting QC from related technology spend, coupled with a lack of data on defense-related investment. The real level of funding for the US, for example, is likely to be higher than reported in Figure 8.
Examples of national/regional programs include:
- China — a major focus on quantum communication, with large investment in the complete range of quantum technologies.
- Germany — diverse areas of research and strong collaboration, with a focus on creating industrial applications in key sectors.
- France — building strong ecosystems between industry players, startups, and researchers with a focus on both quantum computers and enabling technologies.
- UK — targets computing, security, and sensing with a strong focus on medical imaging and has the third highest number of startups globally.
- US — focus on error reduction, techniques for interconnecting cubits, new quantum algorithms, and quantum networking/telecoms to counter Chinese investment in this area.
- Canada — aims to drive innovation through cooperation between universities and private companies, such as QC pioneer D-Wave Systems.
- EU — the European Quantum Flagship involves over 5,000 researchers from France, Germany, Italy, the Netherlands, Spain, and Switzerland. The long-term goal is to develop a “quantum web” of computers, simulators, and sensors.
- Japan — startups specialized in software, with major players focusing on quantum telecoms and cryptography.
Research institutes & universities
Given their relative size, most research institutes are focused on specific areas within QC, often working closely with national programs, industry players, and startups. A nonexhaustive list of examples includes:
- Massachusetts Institute of Technology (US) — research into quantum sensing with diamond, trapped-ion qubits, quantum photonics, and computational algorithms.
- University of Maryland (US) — focuses on QC architectures, algorithms, and complexity theories.
- Yale (US) — research includes quantum sensing and communications, superconducting, and photonic qubits.
- University of Science and Technology of China (USTC) National Laboratory for Quantum Information Sciences (China) — focuses on QC and metrology for military and civilian applications.
- NLQIS National Laboratory for Quantum Information Sciences (China) — recently launched laboratory focused on QC and metrology for military and civilian applications.
- Max Planck Institute (Germany) — research into quantum optics and fundamental research on quantum simulator and cold atoms in optical lattices.
- Fraunhofer Institute (Germany) — focuses on application-oriented research and operates the most powerful quantum computer in Europe with IBM.
- Commissariat à l’Energie Atomique et aux Energies Alternatives (CEA)-Leti (France) — leading laboratory for applied research on electron spin and photonic qubits and their fabrication.
- CEA-List (France) — research in software engineering and cryoelectronics.
- CEA-Direction de la Recherche Fondamentale (DRF) (France) — research in superconducting technologies.
- Centre national de la recherche scientifique (France) — fundamental research in physics, mathematics, and algorithms.
- National Institute for Research in Digital Science and Technology (Inria) (France) — research on quantum technologies, quantum error correction, cryptography, and quantum algorithms.
- University of Cambridge (UK) — research focused on quantum algorithms, simulations, metrology, and cryptography, alongside the foundations of quantum mechanics.
- University of Oxford (UK) — leads the UK’s QC & Simulation Hub, working on creating a network of trapped-ion quantum computers.
- University of Bristol (UK) — dedicated research group on photonic QC.
- University of Waterloo (Canada) — research focused on error correction and fault tolerance, quantum algorithms, ultracold atoms and trapped ions, spin-based quantum information processing, and cryptography.
- Institut Quantique (Canada) — focused on process/control of quantum information in low-temperature environments and theoretical research in design of qubit-based systems and algorithms.
Global technology companies
Most technology companies are focusing heavily on superconducting QC technologies, although some (e.g., Microsoft and Honeywell/Quantinuum) have also embraced other approaches:
- Amazon — entered the quantum cloud market at the end of 2019, and its cloud offering currently covers quantum computers from D-Wave, Rigetti, and IonQ. It is currently developing an in-house quantum computer using so-called cat-qubits (superpositions of two coherent sets of fluctuations with opposite phases in the same oscillator), which are said to be more resistant to some forms of error, such as environmental noise.
- Google — focusing on superconducting qubits, Google has the ambition to build an error-corrected quantum computer within the next decade and create a machine with 1 million qubits by 2029.
- Honeywell/Quantinuum — Honeywell created Quantinuum as a standalone business together with Cambridge Quantum in late 2021. It is building a trapped-ion quantum charge-coupled device (QCCD), using ytterbium-based ions coupled with barium ions to provide cooling.
- IBM — a main focus is on superconducting qubits, with additional fundamental-level research on electron spins and Majorana fermions qubits. It launched its 127-qubit Quantum Eagle processor in late 2021 and plans to release its Condor 1,121-qubit machine inside a large dilution “super fridge” in 2023.
- Intel — main focus is research and development on silicon qubits. The company produced a wafer with 26-qubit chipsets in 2017 and has made some progress in manufacturing quality and cryogenic control electronics since then.
- Microsoft — R&D focus is on the development of the topological qubit, which has not yet been demonstrated practically. On the cloud side it introduced Azure Quantum, a full-stack, open cloud ecosystem in 2019.
- Thales — working with academic partners in France and Singapore, Thales is focusing on quantum sensors, quantum communications, and post-quantum cryptography, using cold atom technology, NV centers, and superconducting devices.
- Alibaba — invested with USTC to start the Alibaba Quantum Computing Laboratory in Shanghai, focusing on quantum cryptography and QC. They develop superconducting fluxionium qubits.
- Tencent — launched a Quantum Lab in 2018 based in Shenzhen, offering QC resources in the cloud as well as work in quantum simulation and ML algorithms.
There are an estimated 450 startups and small businesses globally focused on quantum technologies, some of which are very well funded. They play key roles in prototype development and commercialization of specific QC technologies. Notable startups include D-Wave, creators of one of the first commercially available quantum simulators, as well as Rigetti, IonQ, and QCI. Some other significant startups have also been mentioned in the highly selective list below:
- Alice & Bob — focused on developing self-correcting qubits to develop fault-tolerant and universal gate–based QC. Alice & Bob has created a qubit immune to quantum state reversal, one of the two main types of errors quantum computers suffers, and has raised over €27 million.
- ColdQuanta — founded in 2007 in the US, ColdQuanta develops laser-based solutions for cooling cold atoms and is also designing a cold atom-based computer.
- D-Wave — the main commercial player in quantum annealing, it has developed an end-to-end quantum annealing computer solution. The company has installed more than a half-dozen quantum computers at customer sites and operates more than 30 in its own facilities, with more than half of them dedicated to cloud access. Over 250 early quantum applications have been developed through D-Wave, its customers, and partners, although only a few of them are production-grade.
- IonQ — offers full stack software adapted to its ytterbium (and now barium) trapped-ion quantum computer. It plans to make rack-mounted quantum computers small enough to connect in a data center by 2023.
- Quantum Computing Inc. (QCI) — created Qatalyst, a high-level software development and cloud provisioning tool. This enables developers to solve constrained optimization problems using gate-based accelerators, quantum annealers, or conventional computers.
- Quantum Motion — created in 2017 as an Oxford University spin-off, focusing on developing high-density silicon quantum computers.
- PASQAL— a spin-off from the French Institut d’Optique, PASQAL is working on quantum processing units made up of hundreds of atomic qubits in 2D and 3D arrays, combined with a programming environment to address customer needs in computing and simulation of quantum systems. It delivered its first 100-qubit system in 2022, running in the cloud through OVHcloud and soon, on Microsoft Quantum Azure cloud.
- PsiQuantum — founded in 2016 by Jeremy O’Brien, a former Stanford and Bristol University researcher, focusing on creating a photon-based quantum processor in complementary metal–oxide–semiconductor (CMOS) silicon technology.
- ORCA Computing — an Oxford University spinout aimed at developing scalable photon-based quantum computer systems based on the concept that “short-term” quantum memories could synchronize photonic operations.
- Rigetti — launched the world’s first multi-chip quantum processor, the 80-qubit Aspen-M system. It operates along the entire technology stack, designing and fabricating quantum chips and processors, managing the QC control system, and providing cloud-based services and software tools.
- QuantumCTek — a Chinese company focused on quantum-enabled ICT security products and services.
Remarks on the QC market
The QC market does exhibit some noteworthy characteristics:
- The scale of activity is already significant, yet we are still a long way from commercialization. QC devices are already available for use via the cloud (e.g., D-Wave’s quantum annealer and IBM’s 127-qubit machine), but customers are still almost entirely from the academic and corporate R&D communities.
- The QC market is somewhat unique in that it has been born essentially “cloud-native,” meaning that from its earliest stages it has been developed to be accessed via the cloud. It seems likely that this will continue to be overwhelmingly the case, although there may in future be some customer segments, e.g., governments and some industrials that would have different requirements.
- So far, there is a relatively high degree of openness from the key players in communicating their R&D results and roadmaps. At this stage of maturity, it is in everyone’s interest to be open, and extended partner ecosystems are essential to tackle the complex challenges involved. There may well be a stage further down the development and commercialization path where this changes, but the ecosystem currently is fairly open and transparent.
- The market can be expected to continue to expand if perceived progress is rapid enough to meet current expectations. What is needed for commercialization is a match of supply and demand: the availability of devices that can provide adequate performance and the availability of customers with requirements that match the performance on offer. This is another important reason for potential customers to become engaged in the market sooner rather than later.
5
Understanding QC applications across sectors
According to vendor roadmaps, the first commercial 1,000-qubit quantum computer could be available in 2023, with a 1 million-qubit machine launched by 2030. However, as discussed previously, these estimates should be treated with caution, and timescales of 2030-2040 are more realistic for the availability of large-scale quantum computers.
This does not mean that users need to wait until the next decade to start with QC. While it is a non-gate-based machine, the D-Wave analog quantum annealer is already available on the market, with customers either buying a machine for an estimated $15 million or accessing quantum capacity as a service from D-Wave or Amazon. Some customers are paying up to $200,000 per year for usage. IBM devices are also available via the cloud.
Given that the physical number of quantum computers is likely to remain small and purchase prices high, this cloud-based model of access probably will be the predominant way of accessing QC capacity, with the possible exception of, say, government or large corporations that might have the necessary resources to acquire and maintain their own devices. Typically, vendors will offer QC alongside existing supercomputer capacity to meet customer needs.
The quantum annealer and other forthcoming machines can handle simpler algorithms such as material design or small-scale protein folding and have already benefited some customers.
Ultimately, the goal of the cloud access providers is to offer a seamless service that could integrate networks of quantum and conventional devices to match the needs of the problem to be solved.
It is important to remember that simply demonstrating quantum advantage may not be enough to make a QC application attractive to an industrial customer. Customers will be concerned with the overall benefits, typically involving a trade-off between costs and performance. For example, in some applications, speed of processing may not be the most important criterion.
As QC technology advances, more complex algorithms and problems will be solvable via devices. Developers and end users are increasingly collaborating to create specific applications. We can reasonably expect some applications to be available sooner than others, given the differing complexities involved (see Figure 9).
For example, some successes have already been achieved in demonstrating the feasibility of modeling small-scale protein folding. In general, many observers expect that small-scale simulation applications will be among the earliest to become available.
Overall, there are many potential applications for QC across industry sectors, varying in terms of their relative complexity and timescales for becoming feasible. We have summarized these for selected key sectors below.
Healthcare
Given the high cost and complexity of creating new drugs, QC offers enormous potential rewards to healthcare and pharmaceutical companies, making it a particularly active market. QC can dramatically shorten drug discovery timescales by modeling/simulating drug-to-target interactions and molecular docking, providing better insight into a drug’s performance and viability before entering the lab and performing in vitro testing. This in-silico large-scale drug discovery is still some way (10+ years) off, given the complexity of the problem and currently available QC power.
Other application areas of interest include improving AI and ML for clinical trial planning, optimization of global supply routes, optimizing lab usage, assessing combinations of different treatment options, and supporting analytics for digital health systems.
However, some small-scale applications have already been developed, many using hybrid QC solutions:
- GlaxoSmithKline (GSK), using the D-Wave Advantage annealer and its Leap Hybrid Solver, solved a mRNA codon optimization problem, which worked for 30 amino acids but could be scaled to 1,000.
- Cambridge Quantum is applying EUMEN, a commercially available quantum chemistry application, to Alzheimer’s drug research.
- D-Wave has carried out experiments on minimizing radiotherapy patient exposures to X-rays by simulating the diffusion of electromagnetic waves in the human body.
Protein folding is another area of interest that is key to creating drugs and therapies for destructive diseases, as well as for the wider field of bioengineering. Conventional algorithms cannot handle the intrinsic problem of predicting the three-dimensional structure of proteins, but QC can. IBM has successfully folded a seven-amino acid neuropeptide using a nine-qubit machine and the 10-amino acid chain Angiotensin using a 22-qubit device. Biogen, 1QBit, and Accenture are aiming to retarget therapeutic molecules in existing treatments for neurodegenerative or inflammatory diseases.
Chemicals
Chemicals applications include in-silico molecular simulation, as well as catalyst design and production/supply chain optimization.
Molecular simulation may be used for chemical process design/improvement, new material development, and catalyst design. It gives insight into the physical and energetic behaviors of different processes and reactions and is difficult to model using conventional algorithms due to the requirement for exponential processing power growth.
Quantum simulations of molecules (in-silico) could reduce or even replace laboratory R&D and physical testing, reducing costs and improving process and material design effectiveness. As these simulations are generally less complex than in healthcare, they should be available to run on quantum hardware relatively quickly, although simulating larger chemical reactions will require a greater number of qubits to deliver results.
Production and supply chains involve multiple players and factors, which all interrelate. Unforeseen variables, such as geopolitical or supply chain disruptions, can all impact production. Use of QC in the chemicals industry should therefore help companies to better model and optimize these complex, fast-moving, and interrelated supply chains.
QC has already been applied at a proof-of-concept stage by multiple companies, including:
- 1QBit and Dow demonstrated a novel approach to achieving accurate and efficient simulation of electronic structures using an IonQ trapped-ion quantum computer.
- IBM, using a 16-qubit superconducting quantum computer, simulated a set of beryllium hydride molecules and their minimum energy balance.
- Microsoft and TotalEnergies simulated carbon capture at a molecular level to store in new complex materials.
- Airbus is using QC for the analysis of aerial photography and design of new materials.
- Utilizing an IBM quantum computer, Mitsubishi simulated the initial steps of the reaction mechanism between lithium and oxygen in lithium-air (Li-air) batteries.
Energy
The energy sector is transforming to achieve net-zero targets. The development of renewables (including batteries for electric vehicles and energy storage) and the deployment of smart grids will pose significant design and management challenges, which quantum computers could help address.
In the case of battery design, digital simulation and modeling of battery chemical and physical processes quickly exceeds the capacity of conventional computers. Quantum computers could provide a solution and help to enable a new era of highly efficient post-lithium batteries.
The increasing deployment of smart grids and distributed energy generation will greatly increase the complexity of power management. Quantum computers could be key to managing this complexity, successfully forecasting, planning, and optimizing power grids. Currently, quantum annealing algorithms for power grid optimization show promise on small problems but lack sufficient scalability to be applied to larger areas.
Examples of QC in the energy sector include the following:
- AMTE Power is using NV center-based magnetic quantum sensing to evaluate the battery aging process and develop lithium-based battery manufacturing solutions.
- BP is designing algorithms for optimizing oil exploration using data from various sensors, particularly seismic sensors, to consolidate models for simulating geological layers.
- The Dubai Electricity and Water Authority is collaborating with Microsoft to develop quantum solutions for energy optimization.
- Samsung is partnering with Quantinuum and Imperial College London to study battery development using QC.
Telecommunications
Telecom QC use cases revolve around cryptography and network optimization. Quantum computers will have the ability to break many of the currently used cryptography systems that protect the confidentiality and integrity of digital communication. To prepare for the almost-inevitable advance of QC power, the US National Institute of Standards and Technology is in the process of establishing standardized quantum-resistant cryptographic algorithms to be available in 2024. By developing and standardizing algorithms for post-quantum cryptography before quantum computers develop their cryptography-breaking capabilities, both governments and businesses will be better prepared to defend their digital services against quantum attacks.
Additionally, in terms of optimization, operators will be able to better plan and optimize their networks using QC. This includes understanding where best to deploy base stations and cells to maximize performance while ensuring financial efficiency.
Several use case implementations have already taken place among telecoms, with larger deployments possible in the future as quantum computer power increases and algorithms develop:
- BT and Toshiba have deployed a pilot 6-km quantum key distribution (QKD; a secure communication method for sending keys) to connect several industrial sites as part of the Agile Quantum Safe Communication program.
- Verizon has launched a three-site QKD trial in Washington, DC, and tested a virtual private network using a post-quantum cryptography solution to connect two sites in the US and the UK.
- TIM has optimized the setup of 4G/5G radio cell frequencies using a D-Wave QUBO-based algorithm.
- SK Telecom has secured its 5G network using quantum random number generator technology to the subscriber authentication center.
Transport & logistics
Transport and logistics quantum applications focus on fleet optimization, supply chain optimization, and traffic planning and management. This includes the routing of vehicles, such as the navigation of autonomous cars.
Supply chain optimization is a complex problem with multiple parties, processes, variables, and constraints involved. For rail, road, and air transportation networks, maximizing the efficiency of fleets, managing large-scale traffic systems, optimizing routes, and improving asset utilization are all key issues. For example, Deutsche Bahn has pointed out that even a simple 20-station railway route optimization problem would take a conventional computer two years to calculate. Yet the company operates a network of 5,500 stations in Germany. This exponentially growing complexity means that QC solutions could be transformative for the transport and logistics industry, and even more so when autonomous vehicles are deployed.
Already, some hybrid algorithms have been developed for flight optimization at a small scale. However, logistics route optimization at more granular levels will require more qubits and hardware stabilization against noise and errors if it is going to scale.
Use case examples in transport and logistics include:
- Sumitomo launched a pilot experiment for optimizing flight routes for urban air mobile vehicles in June 2021.
- Working with D-Wave, Ferrovie dello Stato Italia is working to optimize train arrivals in railway stations and minimize passengers’ connection times.
- Volkswagen has partnered with D-Wave to optimize the routes of a 10,000+ cab fleet in Beijing.
- Toyota will use Kawasaki Business Incubation Center’s IBM computer to analyze big data taken from previous car trips, which can be used to guide vehicles along specific routes to avoid traffic jams.
Finance & insurance
Finance and insurance applications center on improving on supercomputer performance in areas such as portfolio optimization, forecasting, risk modeling, and high-frequency trading, as well as increasing the accuracy of automated fraud detection.
In the financial sector, forecasting, modeling, and optimizing the risk associated with an investment portfolio is crucial. The most commonly used method of achieving this, Monte Carlo simulations, require a very long computational time, often running for days. This currently forces a tradeoff between ensuring problems are complex enough to capture the nuances of the real world without being so complex that they are too computationally demanding for current conventional supercomputers. In theory, the quadratic speedup QC provides can reduce calculations to make them almost real time, allowing enormous improvements in risk assessment. Similarly, rapid execution of complex quantitative buy-sell strategies (e.g., in the domain of high-frequency trading) will improve financial firms’ abilities to generate greater returns while controlling risk.
To spot potential fraud and determine the credit worthiness of applicants, financial services organizations need to analyze enormous data sets that can have millions of entries, each with thousands of different properties. Quantum computers can drastically reduce the cost and time of processing this data, ultimately enabling real-time analysis and response.
However, activities to date are mainly proofs of concept and trials. This is because the quality and fidelity of qubits need to be increased for certain financial algorithms to attain quantum supremacy. Current examples in the financial and insurance sectors include:
- NatWest has used quantum-inspired algorithms running on traditional computers to optimize its investment portfolios.
- Microsoft has devised a method to make stock value predictions using topological computing.
- IBM, JPMorgan Chase, and Barclays have partnered to study the uses of quantum in trade-strategy optimization, investment-portfolio optimization, pricing, and risk analysis.
- CaixaBank has used D-Wave’s quantum cloud and hybrid solver services for its portfolio hedging calculations. It reported a 90% decrease in time-to-solution, while optimizing bond portfolio internal rate of return by up to 10%.
- PayPal partnered with IBM in 2020 to apply QC to improve its fraud detection systems and overall digital security integrity.
Defense & aerospace
Defense and aerospace applications are huge in scale and diversity, ranging across sensing, cryptography, communications, optimization, and simulation. Many actual use cases remain secret, but others have been announced, such as around long-range weather forecasting and optimization of new materials. However, the complexity of many of these applications means that quantum technology will need to develop further before they can be successfully realized.
Current use case examples in the defense and aerospace industry include:
- Lockheed Martin partnered with Google and NASA to test D-Wave annealers in 2014 to develop a solution for formal proof-of-software operation.
- Airbus is using QC for the analysis of aerial photography and design of new materials.
- The US Air Force is aiming to utilize quantum timing, quantum sensing, quantum communications/networking, and QC in a variety of applications.
- The European Center for Medium-Range Weather Forecasts has partnered with Atos to develop a Center of Excellence, where they have access to Atos’ Quantum Learning Machine to research the potential of QC in weather and climate forecasting.
Interlude #2 — Quantum photography
When photography took off at the end of the 19th century, painters found themselves without a means of subsistence. Portraits, which used to be the privilege of rich families, became accessible to the bourgeoisie, and painters had to reinvent their art in order to survive. This turn of events gave birth to a radical evolution in painting with the advent of pictorial movements like Impressionism, Pointillism, or Fauvism. With Cubism, painters even drew their inspiration from cutting-edge science of their time to rethink the theories of representation and developed new ways to visualize three-dimensional objects on a two-dimensional canvas.
Today, photography is about to suffer a shake-up of similar magnitude. Smartphones are everywhere, and figurative photographs are quickly losing both their visual appeal and business value, forcing photographers to rethink their art entirely. While a significant part of the photographic community is returning to the roots with a renewed interest in the many techniques that were developed at the end of the 19th century to produce images from light, another part of the community is considering the digital aspect of modern photography and videography as a core element of their art, with no obligation whatsoever to emulate silver prints.
Quantum photography and quantum video find their roots in the second trend. Instead of considering the artifacts resulting from the pixelation of images as a necessary evil that must be hidden at all costs by a never-ending increase in the resolution of camera sensors, quantum photography proposes to take advantage of phenomena of quantum essence so that these “defects” serve as a basis for the development of an entirely new type of creative process. Quantum photography always starts with a digital photo or video. This approach differs from that of digital artists who synthesize images from scratch using AI. In a sense, quantum photography serves as a missing link between traditional photography and more abstract or conceptual visual arts.
Quantum photography produces a new breed of hybrid images, both figurative and abstract, evolving freely between photography and painting. This duality is reminiscent of the wave (smooth) and particle (granular) models of matter in quantum mechanics; from a visual standpoint, quantum photographs simultaneously show two complementary aspects of our world: the “granular texture” of the microscopic world at the atomic scale and the “smoothness” of the world at our own scale.
Beyond its marketing appeal, the name “Quantum Photography” itself thus refers to several key characteristics of quantum mechanics, such as:
- Particle-wave duality, as explained above (see Fig A)
- Quantification of both space (pixels) and time (video)
- Interférence phenomena (moiré effects for instance)
In addition to physics analogies proper, Quantum Photography also makes use of other global mathematical manipulations of images such as Mathematical Morphology, leading to images that have never been seen before (Fig B, C, and D above) or sometimes look like paintings (e.g., Fig E, which looks like a Cubist portrait). In that respect, the nascent field of Quantum Mathematical Morphology (quantum digital image-processing algorithms based on quantum measurement) may use quantum computers to speed up computations that quickly become prohibitive when processing quantum videos, as such processing is performed on an image-by-image basis, and computations for a single image, even using the best concurrent programming techniques, can sometimes requires tens of seconds of processing time on the most recent desktop computer.
— François Bourdoncle, artist
6
Conclusion: Readying your business for the quantum future
Going beyond the hype, QC clearly offers disruptive potential to solve specific, high-value problems that are beyond the effective capabilities of today’s supercomputers. When these machines will be available is an open question — there remain significant challenges at the physics, engineering, and algorithm levels that need to be overcome to enable success. At best, most current breakthroughs are at the lab scale proof-of-concept stage.
However, as with other emerging technologies, development is not linear. Breakthroughs may unlock and accelerate progress in key areas, with commercialization potentially following relatively swiftly. Consequently, while market growth predictions vary wildly (see Figure 10), they could reach $65 billion by 2030, although the consensus is closer to $20 billion. However, these are more assumptions than predictions given the uncertainties involved.
The QC learning slope is steep. It will not be possible, especially in the earlier stages of commercialization, for inexperienced users simply to do nothing until the technology matures then buy access to quantum processing power and immediately expect to make transformational changes in their business operations. On the other hand, those companies that have already explored potential use cases, understand the sort of algorithmic solutions that could be applied, and have already developed partnerships and relationships in the QC ecosystem, can expect to achieve major competitive advantage.
Another key consideration is how the QC industry will evolve as the technology matures. Currently, there is quite a high degree of willingness to share data and intelligence across competing players, partly because at this stage of maturity it is broadly in everyone’s interests to be open. However, this might change once quantum technology reaches the scale-up stage, when key players will need to safeguard competitive advantage and maximize their returns on investment. A further key issue is the extent to which geopolitics may play a role, especially in light of the critical importance of quantum technology for cryptosecurity and defense. Those companies that lack any internal knowledge and capability could find themselves additionally disadvantaged in such scenarios.
Businesses should therefore take steps now to ensure that they are not left behind if and when breakthroughs occur. Following the four steps in Figure 11 will position an organization to reap the future benefits of QC.
1. Explore applicability
Start by understanding how QC could benefit your business and in which areas it could be applied. Look at your operating model and identify activities, processes, or functions for which simulation, optimization, AI/ML, and cryptography are potentially important. Create a list of specific intractable problems that cannot be solved by conventional computing, prioritizing those that would provide significant, disruptive advantage if they were solved. Focus on answering three questions:
- Where could quantum applications in simulation, optimization, AI/ML, and cryptography deliver advantage in your current business operations (e.g., in terms of cost, speed, performance, efficiency, or quality)?
- Where do you currently use or plan to use high-performance computers but struggle with their limitations?
- Where could you deliver real disruption to your business model or industry by solving intractable problems, or problems that you never before considered solvable?
Being creative is an important aspect of this exercise and involves identifying new radical challenges and opportunities, not just addressing current known challenges and opportunities faster.
Once identified, you can screen and rank these potential opportunities in terms of, for example, scale of business impact, maturity, and likely timescale ranges in the normal way to help inform your strategy.
2. Monitor technology developments
QC is evolving rapidly, with a large volume of new innovations, roadmaps, and research being released. As with any emerging technology, you should ensure you are on top of developments by putting in place a suitable technology monitoring process. This process needs to be of a scale appropriate for your business; for many organizations, a light-touch approach currently may be sufficient.
Any technology-monitoring process has several basic elements:
- Scanning. Flagging areas of interest as they arise using both targeted search tools and public data sources.
- Monitoring. Following identified trends in a systematic way, for example by subscribing to forums, conference attendance, and regular landscape reviews.
- Scouting. Actively engaging with relevant players and encouraging inputs from others.
- Evaluating. Assessing the specific relevance and potential of emerging developments, and sharing tailored intelligence internally within the business.
This means developing or acquiring the capability to understand the technology, setting up the channels to stay abreast of relevant developments, and rapidly evaluating their potential and limitations. An important aspects of this is screening ongoing work on quantum algorithm development targeted at your industry verticals.
3. Engage in the ecosystem
Ecosystem thinking is fundamental to driving effective innovation success. Given the complex, fast-moving nature of the QC market, building collaborations and partnerships with other players is critical. Start by understanding the roles, activities, and level of involvement of different ecosystem players, including government, academia, research institutes, vendors, startups, and investors. Identify those players that are focusing on your sector and establish how your business could become involved in some way — for example, through pilots, cofunding initiatives, staff secondments, or sponsorship of PhD projects. Vendors are looking to collaborate with potential end customers to understand and meet use case needs in the future. Consider working with them on relevant projects to test algorithms, even if performance is not yet powerful enough to deliver breakthrough results. For example, algorithms can already be tested via the cloud with available universal quantum computers, a quantum annealer, or with an emulator.
4. Build knowledge & capability
As part of digital transformation initiatives, most large organizations have built or recruited new capabilities and programming skills, particularly in leading-edge areas such as AI and ML. Based on the significance of the area to your business, invest in educating and training at least some of these individuals in quantum technologies, quantum programming, and quantum algorithms, even if this is at a basic level. This will enable them (and you) to participate effectively in the ecosystem and monitor and evaluate developments. This is especially important for quantum technologies due to their complexity, reliance on specialist expertise, and the level of hype in published media.
In some cases, you might also consider some specialist recruitment. However, you will need to be realistic about the limited availability of specialist QC experts, and typically you may not always need to recruit PhDs in quantum science anyway. It could be argued that you need “quantum application engineers” rather than scientists, a profession that does not yet exist. Hence, a lot can be achieved through education and experience building from within. Creating a motivated and enthused community internally is one of the best ways to ensure that you stay abreast of developments in a natural, rather than wholly process-driven, manner.
Many large potential user companies, especially in the more technology-intensive sectors, have already taken these steps. With the rapid acceleration in research and development activity we are now seeing, if companies have not already started along the path, they do indeed run the risk of being left behind if and when a breakthrough occurs.
Notes
[1] “Overview on Quantum Initiatives Worldwide — Update Mid 2021.” Qureca, July 2021, and ADL analysis.
[2] A logical qubit is a physical assembly of a much great number of physical qubits. Physical qubits are the quantum objects that exhibit the mathematical properties of a qubit, which could be based on, for example, atoms, ions, or photons.
[3] Random access memory (RAM) is the computer memory used to store data and code. Quantum memory is the equivalent function on a quantum computer.
[4] Temkin, Marina. “Investors Bet on the Technologically Unproven Field of Quantum Computing.” Pitchbook, 13 September 2021.
Appendix — Further observations on the second quantum revolution and developing technologies from Professor Alain Aspect
The founding fathers of quantum mechanics doubted that it would be possible to isolate, observe, and manipulate individual quantum objects such as electrons, ions, atoms, and photons. As Erwin Schrödinger expressed, writing in 1952: “We never experiment with just one electron or atom or (small) molecule. In thought experiments we sometimes assume that we do; this invariably entails ridiculous consequences … it is fair to state that we are not experimenting with single particles, any more than we can raise Ichthyosauria in the zoo.”[1] But starting in the 1970s, physicists developed new methods to experiment with single elementary quantum objects: electrons, ions, photons, atoms. And they developed new devices based on superconductors behaving as single quantum objects.
The recently developed quantum technologies that belong in the second quantum revolution rely on control and detection of single quantum objects, as well as on the ability to entangle them. Moreover, in all the schemes envisaged today, one must be able to identify and work exclusively with two states of each object, the result being a qubit evolving in a two-dimensional Hilbert space.
By making two qubits interact, one can entangle them. Experimental tests of Bell’s inequalities with photons demonstrated that entanglement does exist as described in the formalism of the theory, with its mysterious non-locality. These experiments were possible only because we could produce individual pairs of entangled photons and detect individually each photon of the pair.
As I mentioned in the Preface, it is likely that there will not be a single qubit better than others for all functions we want to realize. Take, for instance, a photon, whose states of polarization are described in a two-dimensional Hilbert space; it is almost ideal since it is insensitive to most perturbations, such as stray magnetic fields. But you cannot stop it, so in addition to such flying qubits, we also need static qubits that can store the quantum information until it is processed. At the moment, the best natural static qubits are ions and neutral atoms, which are quantum objects that are absolutely identical and obey elementary physics laws, so we can describe their behavior in detail. On the other hand, devices based on superconductors are artificial qubits produced by nanotechnologies, and one cannot be sure that they are all alike. Their qualities may vary depending on the fabrication process.
To evaluate the potential of the various qubits, a key feature to consider is scalability; in essence, the possibility to increase their number without deteriorating the quantum behaviors of the ensemble. The dimensionality of the ensemble of qubits obviously plays a role, and the scalability is better when the dimension is higher. Ion traps are linear, so the size of the system increases linearly with the number of qubits, although there are some prospects to develop 2D ion traps. Neutral atoms, either in optical lattices or in multiple tweezers, are naturally 2D, so the size increases as the square root of the number of qubits. For a number of several hundreds, this is a killer advantage. I do not know enough about superconducting devices, but it seems that their big advantage is the possibility to use the already much-developed techniques used for micro-electronics, so scaling is natural. One must be aware, however, that to achieve useful scaling, it must be accompanied by a simultaneous increase in the quality of the qubits. This is no small problem.
As a last point on elementary devices, I want to stress the importance of developing good quantum memories, able to store the states of qubits and to restitute them on demand. These quantum memories will be important not only as registers for quantum computers but also in quantum information transmission. For the latter, it would be very important to have quantum memories able to store the quantum state of photons. I think there are true market opportunities here, because many quantum technologies already demonstrated — such as QKD or quantum teleportation — will need quantum memories to be really useful. They will also be crucial for linking future quantum processors, or as an element of an optical quantum computer.
I am more optimistic regarding quantum simulators than universal quantum computers. Quantum simulators comprise an ensemble of qubits with interactions designed to produce an entangled system whose fundamental state is hard to compute with a classical high-powered computer. As mentioned in the Report, the first successes of such simulators have been about physics questions, but the ensemble of qubits could be prepared in a state that will evolve toward a steady state that represents the solution of a hard optimization problem, such as the traveling salesman problem.
This is only one of the many possibilities quantum simulators offer, and we will know soon if these hopes become a reality. Personally, I think that there is a good chance to obtain useful results, for instance regarding optimization of the electric grid. It seems also that interesting results may be expected in the quantum description of molecules of biological or medical interest. Moreover, the most advanced simulators of today are platforms that have the potential to be converted into universal quantum computers. Meanwhile, I want to stress that such simulators have already demonstrated a quantum advantage in the resolution of the Ising problem, which is a physics problem that has been open for decades.
Finally, in my opinion, true quantum advantage can be claimed only if it applies to a problem that was open before the quantum simulator was set, and for which one can explore a range of parameters. The Ising problem is such an example, and currently there is a race between the results obtained by experimentalists and those of the experts in classical computing, who develop approximations that allow them to obtain results in a reasonable time. Today’s simulators have already demonstrated a quantum advantage in the problem’s resolution. At the moment, the quantum simulator is ahead but classical computing catches up regularly. The good news is that their results coincide, which is a valuable cross check of the two approaches.
Cat qubit — Cat states are quantum superpositions of coherent qubit states with opposite phases. They have been postulated, notably by Alice & Bob and Amazon, as part of a novel approach to quantum error correction. They enable the creation of qubits that are natively flip-error-proof. Fault-tolerance is implemented using quantum error correction only for phase flips, which reduces the number of qubits required.
Decoherence — Quantum coherence refers to the ability of a quantum state to maintain its entanglement and superposition. Decoherence refers to the loss of coherence.
Entanglement — Quantum entanglement is the physical phenomenon that occurs when a group of particles is generated, interacts, or shares spatial proximity in a way such that the quantum state of each particle of the group cannot be described independently of the state of the others, including when the particles are separated by a large distance.
Quantum supremacy/quantum advantage — Quantum supremacy or quantum advantage refers to demonstrating that a programmable quantum device can solve a problem that no conventional computer can solve in any feasible amount of time (irrespective of the usefulness of the problem).
Quantum key distribution (QKD) — QKD is a secure communication method that implements a cryptographic protocol involving components of quantum mechanics. It enables two parties to produce a shared random secret key known only to them, which can then be used to encrypt and decrypt messages.
Superposition — The principle of quantum superposition states that any two (or more) quantum states can be added together (“superposed”) and the result will be another valid quantum state. Conversely, every quantum state can be represented as a sum of two or more other distinct states.
Quantum sensing — Quantum sensing refers to the design and engineering of quantum sources (e.g., entangled) and quantum measurements that can beat the performance of any conventional strategy in a number of technological applications, such as microscopy, positioning systems, communication technology, and electric and magnetic field sensors.
Quantum communications — Quantum communications refers to a wide range of usually photon-based telecommunications technologies at different levels of maturity that take advantage of the laws of quantum mechanics to enable, for example, cryptographic security.
Qubit — A qubit or quantum bit is the quantum version of the classic binary bit physically realized with a two-state device. A qubit is a two-state (or two-level) quantum-mechanical system. Physical examples include the spin of the electron in which the two levels can be taken as spin up and spin down; or the polarization of a single photon in which the two states can be taken to be the vertical polarization and the horizontal polarization.
Technology assessment
As part of this Report, we have assessed the seven key competing core qubit technologies in terms of maturity, pace, and disruption potential. Maturity is evaluated based of the established technology readiness level (TRL) scale set out in Figure 12. Pace and potential for disruption are assessed based on the framework in Table 2.
Superconducting
Superconducting is currently the most mature technology and is developing quickly. There are, however, many obstacles still to scale up.
Description — Ground stage designed by first Cooper pair box circuit produced in 1997 by Vincent Bouchiat, with the first demonstration achieved in 1999 by Yasunobu Nakamura and a first functioning version verified in 2002. A widely used qubit design with distinctive characteristics — at superconducting temperature electrons form an artificial atom with an energy level that can be controlled accurately. Three types of superconducting qubits exist: phase, flux, and charge qubits.
Key benefits — Enabling technologies already exist and the approach has good scalability in 2D architecture. Noise reduction techniques are constantly advancing. The technology is heavily backed by both major companies and large research institutions.
Key hurdles — Qubits currently suffer from a low coherence time and high noise levels, while qubit coupling is constrained. The technical complexity of the approach is high, requiring calibration and advanced cryogenic technology, and many electronic components with large-scale cabling for industrial applications.
R&D themes — Noise reduction is a major research theme to make superconducting qubits feasible for industrial applications. At the same time, cryogenic technology needs to advance to support technical complexity.
Key players — The main technology is being actively pursued by both public research institutes and large corporations, with major investments from both groups. Players include Alice & Bob, Amazon, D-Wave, Fujitsu, Google, IBM, Intel, IQM, QCI, and Rigetti, as well as physics labs in countries such as Austria, France, Germany, the Netherlands, Russia, and the US.
Maturity — TRL 4: Technology validated in limited form at lab scale.
Pace —
Disruption potential —
Electron spin
Electron spin has potential for good scalability due to miniaturized architecture and low error rates, but has only been demonstrated at small scale so far.
Description — Research began after the development of superconducting qubits and was first presented by Daniel Loss and David P. DiVincenzo in 1997. Based on the spin orientation of an electron secured in a semiconductor structure, it is a potential candidate for large-scale applications due to its miniaturized architecture and low error rates. It operates at higher working temperatures than superconducting (around 1 Kelvin/-272 Celsius). The qubit readout works via conventional electronics by measuring the electrical charge.
Key benefits — The qubit production process is similar to semiconductors. They display good scalability due to their small size, along with good quantum gate speed. The 1 Kelvin temperature requirement is less extreme than some other types and they have a 2D architecture.
Key hurdles — Currently, limited entanglement has been achieved, while qubit fidelity is average and qubit variability is currently unconfirmed. Manufacturing costs are high.
R&D themes — R&D is focusing on five variations of electron spins qubits (arsenic/gallium, silicon, germanium on silicon, atom ions implanted on silicon, and graphene structures trapping single electrons). R&D is also looking at “hot” qubits operating at higher temperatures.
Key players — Players include EeroQ, Equal1 Labs, Intel, Quantum Motion, as well as physics labs in countries such as Australia, France, Germany, Japan, the Netherlands, the UK, and the US.
Maturity — TRL 3: Proof of concept exists.
Pace —
Disruption potential —
Photon
Photon qubits have the advantage of room-temperature operation, but scalability challenges are significant and the technology is still relatively immature.
Description — Based on photons, exploiting their polarization or other physical characteristics, with single photons produced by lasers or diodes. While the development of model components started in the 1920s, the first QC applications began in the 1990s. In contrast to other qubits, photons have no mass and move at the speed of light, making them the only qubit type that can travel over long distances. They are stable qubits at ambient temperatures and can be applied in quantum computation, simulation, communication, cryptography, and sensing. However, they are not yet scalable due to the probabilistic character of quantum gates and require the implementation of specific computing techniques.
Key benefits — Stable qubits that work at room temperature. New manufacturing technology enables scalability or can be adapted from semiconductor processes. They are easier to scale due to communication between qubits and quantum communication.
Key hurdles — Scalability is currently hampered by the inefficiency of photon sources and quantum gates. Information cannot be stored on photons, leading to delays. Some cooling of enabling technologies is required, however temperatures are not critical. Quantum advantage is theoretically existent but practically not proven.
R&D themes — Improvement of manufacturing processes, research into multiple photon sources. Research into a wide field of applications and using them as an enabler for other qubits. In December 2020, a photonic quantum computer with 76 photons was demonstrated.
Key players — Players include ORCA, PsiQuantum, Quandela, and Xanadu as well as physics labs in countries such as Australia, Austria, China, France, Germany, Italy, Japan, the UK, and the US.
Maturity — TRL 3: Proof of concept exists.
Pace —
Disruption potential —
Trapped ion
Trapped-ion qubits show the best stability and error rates so far, but thus far they have been hard to scale beyond 32 qubits.
Description — The idea was first developed in the 1950s by Wolfgang Paul, later a Nobel Prize physicist, but it took until 1995 before they were first tested in a lab. Positive ionized atoms from alkaline metals (e.g., beryllium, magnesium, strontium, barium, and particularly calcium) are trapped in a limited space via magnets or electrodes close to each other. Storage is in the stable electronic states of each ion, and information proceeds through the motion of the ions. Lasers are used for coupling between the qubit states, either in single operations or for entanglement.
Key benefits — No calibration is required due to identical ions. Good stability of qubits, with best-in-class error rates and long coherence time. The entanglement of qubits on a 1D architecture and between ions and photons is possible and the process works at higher temperatures (4-10 Kelvin).
Key hurdles — Questionable scalability beyond 50 qubits, while relatively slow quantum gates could limit applicability for deep algorithms. Trapped ions need to be maintained in a vacuum, adding to complexity.
R&D themes — Research around easy handling of ions compared to other qubit types.
Key players — Players include Alpine Quantum Technologies, EleQtron, IonQ, Honeywell/Quantinuum, Oxford Ionics, as well as physics labs in countries such as Australia, Austria, Canada, China, Denmark, Finland, Germany, India, Israel, Japan, the Netherlands, Singapore, Switzerland, the UK, and the US.
Maturity — TRL 3: Proof of concept exists.
Pace —
Disruption potential —
Cold atom
Cold atom qubits have acceptable error rates and long coherence times, but potential applicability has been mainly for simulation rather than gate-based computing.
Description — Based on cold atoms of elements such as rubidium held in position with lasers rather than electrodes, cold atoms can also be used in a Rydberg configuration as a source for photons in nonlinear optics. They offer acceptable error rates and a long coherence time. Deploying required lasers and optical control technology is challenging for scaling beyond ~1,000 qubits. Overall, they are better adapted to simulation rather than to universal gate–based computing.
Key benefits — Acceptable error rates, with a long coherence time. As atoms are identical, they do not require calibration. No integrated circuits or advanced machinery are needed, while trapped ion qubit tools can also be used for cold atom readouts. They are focused most heavily on simulation use cases.
Key hurdles — Quantum gate error rates are acceptable, but not as strong as some other approaches. There is also cross talk between qubits and enabling technologies, such as lasers, and optics are currently not readily scalable.
R&D themes — Research into a wide field of applications besides QC, including cryptography, simulations, atomic clocks, and quantum memory.
Key players — Players include Atom Computing, BraneCell, ColdQuanta, PASQAL, and QuEra Computing as well as physics labs in countries such as Austria, France, Germany, the UK, and the US.
Maturity — TRL 3: Proof of concept exists.
Pace —
Disruption potential —
Nitrogen vacancy (NV) centers
NV centers show good stability and simple cryogeny, but their main applicability so far is magnetometry and sensing rather than QC.
Description — NV centers are based on the control of electron spins trapped in artificial defects of crystalline carbon structures. Initial work took place in the 1970s, but only became valid for QC applications in the early 1990s. Qubit readout is via a laser, which measures the fluorescence of the cavity, while microwaves or magnetic fields control the electron spin. A temperature of 4 Kelvins is used, making cryogeny requirements less onerous. The main application is for magnetometry and sensing rather than QC.
Key benefits — Long coherence time and requires simpler cryogenic equipment. Qubits have a strong and stable diamond architecture and can converge with other qubit types to create quantum memory. Additionally, they can be integrated into optical quantum networks.
Key hurdles — Currently, research activity is low and the practical applications of NV centers are limited for QC. Most applications lie in the field of quantum sensing. Controlling qubits via lasers is complex.
R&D themes — The NV centers qubits research field is developing in three areas: toward quantum sensing, use in precise magnetometers, and to leverage for quantum memories.
Key players — Players include Quantum Brilliance and Turing, as well as physics labs in countries including Australia, China, France, Germany, Japan, the Netherlands, and the US.
Maturity — TRL 2: No proof of concept so far.
Pace —
Disruption potential —
Topological
Topological qubits have high potential in theory but have not been demonstrated in practice.
Description — First conceived in 1997 by Microsoft researcher Alexei Kitaev, topological qubits offer a more robust alternative to superconducting qubits. They are harder to knock out of superposition, requiring lower error correction. They are based on using anyon particles and the Majorana fermion variant of anyons as qubits. Anyons are “quasi-particles” (i.e., particle representation models that describe the state of electron clouds around atoms in the superconducting regime). To date, no Majorana fermion qubit has been successfully demonstrated. However, in February 2020, John Preskill (the father of the notions of quantum supremacy and NISQ) predicted that, by 2030, we will be able to demonstrate two entangled topological qubits.
Key benefits — These are stable qubits, with a long coherence time, good scalability, and low error correction requirements. Their high gate speed enables the processing of long and complex algorithms.
Key hurdles — No Majorana fermion qubit has yet been demonstrated, making them currently purely theoretical. Topological qubit programming requires an additional software layer compared to alternative approaches. They require low cryogenic temperatures similar to superconducting qubits. Microsoft is the only potential vendor, although IBM is investigating the field.
R&D themes — Fermion particles are still the subject of basic research by various physical laboratories. In 2018, a Microsoft team predicted a fermion-based machine by 2023, but this is now highly unlikely.
Key players — Players include Microsoft as well as physics labs in countries such as China, Denmark, Finland, France, the Netherlands, and the US.
Maturity — TRL 2: No proof of concept so far.
Pace —
Disruption potential —
Appendix Note
[1] Schrödinger, Erwin. “Are There Quantum Jumps? Part II.” British Journal for the Philosophy of Science, Vol. 3, No. 11, 1952.
DOWNLOAD THE FULL REPORT