DOWNLOAD
8 min read • Technology & innovation management
The global AI arms race - How nations can avoid being left behind
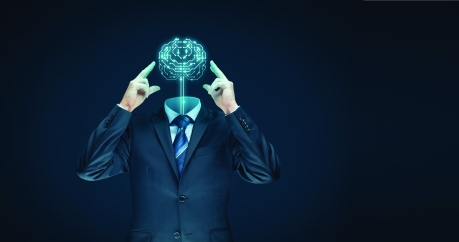
Artificial intelligence promises to have the same disruptive effect on today’s global economy as electricity in the 19th century. This has led governments to focus heavily on how they can unlock AI’s potential within their countries. Based on in-depth research, this article outlines the current winners and losers, exploring what it takes to win in the global AI arms race.
Artificial intelligence (AI) is widely accepted as having the potential to transform virtually every industry and create significant economic value. While businesses play out their AI strategies within their various national and international ecosystems, an often-overlooked dimension is how innovative regulation and governmental support can unlock AI’s full potential. Governments and regulators play a critical role in fostering, or hindering, how technology benefits accrue for their citizens, and the role of academia and research institutes is also crucial for AI technology to develop. In this article we look at the successes and failures of national AI strategies, and identify some key priorities for competing nations, including the role of government as well as private and public stakeholders.
Which are the current leading countries in AI?
There are several country rankings of AI strength across the world. Those that focus on metrics such as patents and research publications tend to list China first, followed by the US, with third place disputed between European and Asian countries including South Korea, Japan and India.
However, taking a broader approach using a composite AI-readiness index (from Oxford Insights) that factors in governance, skills and education, infrastructure and data, and government/public services reveals the top three countries to be Singapore, the UK and Germany. (See Figure 1.)
In order to understand better which national polices and strategies are important for success, on behalf of the government of Singapore we recently conducted a comparative review of 10 of the top 20 countries. These 10 countries were selected on the basis of having comparable GDPs to Singapore (around USD 50–60k per capita) and similar innovation capacities (based on the Global Innovation Index). For these 10 countries, we used our published public reports, global AI indices and our own analysis to cover over 50 AI-related metrics, including enablers and impacts. The impacts were classified across four key dimensions:
- AI start-up activity, including investment in AI startups per capita, number of AI start-ups per capita, and number of AI unicorns per capita
- AI-related jobs, including percentage of AI-related jobs in the economy
- Adoption of AI by private industry, including level of AI adoption among top 10 listed companies, extent of AI government support for small and medium-sized enterprises (SMEs), and funds committed to AI adoption
- AI knowledge/skills capability, including H-index (i.e., quality/quantity) in AI publications, weighted citation index in AI, university-led/-funded incubators in top five universities, and AI patents filed per capita
The study provided valuable insights into what is, and is not, important for countries to compete successfully in AI. A toplevel overview of strengths across the impact dimensions is provided in Figure 2.
- It can be seen that there is quite some difference in strength and focus across the four dimensions. Leading countries have broad coverage across the impact metrics, with a stronger bias towards one or more, based on their context. For example, Germany is the strongest in the sample in private sector adoption of AI, driven by government support of AI-based industrial digitization focused on SMEs and university AI R&D programs that have strong bias for commercialization.
- Canada, the US and Singapore all have strong scores in AI knowledge/skills capability.
- Israel, Singapore and the US have mature and welldefined AI governance frameworks with clear regulatory set-ups, well-established national and regional AI offices, and strong cyber-security and AI risk management practices.
What are the policy levers that have the greatest impact?
Focus on the key enablers
The study analyzed which specific “enablers” correlated highest with each of these impact dimensions. In other words, which are the enabling policies that really make the difference? This is shown in Figure 3.
It can be seen from this analysis that some common assumptions about policy levers are not correct, in particular:
- Growing AI start-up activity: National AI governance is as important as funding
Government funding is part of the solution. However, the support required goes beyond finance, including mentoring, facilitating international collaboration, and a drive for talent development and retention. We found that the most likely contributing factor that encouraged start-ups was the maturity and influence of national AI governance.
- Private sector AI adoption: A triple-helix partnership is more important than having a data-sharing framework and ethical policy
Fostering private participation in AI has little to do with whether data can be obtained from the government or has a strong ethics policy. What private participants are looking for to kick-start the AI journey is the so-called “triple-helix” partnership, with academia, government and the private sector all playing their roles.
- AI knowledge/skills capability: Investment in R&D supported by mature national AI governance is key
Although international collaborations and the triple-helix model play a part in knowledge and skills development, we found that a more direct approach based on government investment in AI R&D and a mature and influential national AI governance approach are even more important.
Build a virtuous AI talent cycle
The analysis showed that ensuring local and global AI talents were developed, attracted, and retained was central to national AI success. We found that:
- The US has almost 40 percent of the global AI talent, while Israel and Singapore have the highest scores for AI workforce and talent development from a tertiary education perspective.
- Countries such as Germany, the US and Canada have been able to attract new AI talent into their economies over the last few years, while China, Israel and Singapore have been unable to retain and grow their talent pools.
A country’s national AI talent strategy should therefore aim to create a virtuous cycle in which an AI ecosystem fosters AI talent concentration, which, in turn, drives private innovation and economic development, boosting the AI ecosystem further. Based on our observations, a coordinated policy effort across academic institutions, public sector support and incentives for private players has the highest likelihood of being successful in creating this virtuous cycle.
Adopt robust methodologies for assessing AI investments
The analysis also showed that the most successful countries had robust methodologies for assessing how much to invest in different aspects of policy. For example, in deciding the size of the incentive required to support increased adoption of AI in the private sector, a business case can be developed studying the economic potential of AI skills by sector and function, netted against potential costs. The approach is illustrated in Figure 4.
Adopt a model across multiple dimensions, anchored in ecosystem thinking
Finally, the study showed that countries with AI strategies across multiple dimensions, including clearly defined national AI visions, investment in AI benefits, and protections for data usage, had higher likelihood of success compared to countries that focused on fewer dimensions.
However, it is essential to realize that the path to success is not the same for all countries. The national context varies tremendously from country to country, and the way in which AI will transform industries and countries is not yet obvious. For example, in the current state of development, AI is at a crossroads, with at least one major dichotomy in terms of how it will progress:
- Global, centralized AI driven by Big Data platforms (e.g., Google and Amazon) that accumulates vast amounts of information about each individual to engineer a digital environment
- Decentralized AI, with significant privacy protections and in which data is controlled by the individual, communities or national governments
This is in many ways analogous to the adoption of electricity in the 19th century, for which progress was neither linear nor predictable, and success depended not just on the technology itself, but also on how it became integrated with broader societal changes. (See Box 1.)
Box 1: AI as the new electricity – A lesson from history
In many ways, artificial intelligence is the new electricity. In the late 19th century, as electricity was being popularized, it became apparent that it required a new framework, as it was different to virtually every other commodity. Whoever mastered the engineering and economics of electricity would indelibly change the course of history. The original model for electrification was limited, as it relied on DC current and local generation. Electrification took off with the switch to the AC current, which allowed long-distance power distribution from centralized generation. This shows that progress with transformative technologies was neither linear nor predictable. The more customers the electricity company had, the more efficiently it could run its generators and the cheaper it was to provide power, and even more people and industries could be served and transformed. The benefits of electrification did not accumulate for early adopters, but for those who had identified how electrification could transform their societies within its existing context.
AI will similarly transform entire industries and countries, and the path will again not be obvious and results will probably not be linear. In its current state of development, AI is at a crossroads similar to electricity in its early years.
This means national policy makers need to adopt AI strategies that are anchored on consideration of the broader local and global ecosystem and tailored to the national context, and that consider multiple actions that may need to take place within different parts of the ecosystem. There is no single path to success
Insights for the executive
Widespread AI-driven disruption is coming, whether we embrace this technology nationally or not. Just like the adoption of electricity, it is enabling winners and losers within the global race for greater productivity. Achieving a competitive edge will have significant consequences, which means governments need to make the right decisions. Essentially, national AI adoption should be designed and rolled out across multiple dimensions with an ecosystem-based philosophy of creating smart people, smart government, enabled industries and companies, and smart technologies. In summary, governments need to consider the following:
- Design and articulate national AI objectives based on their assessment and relevance of likely future scenarios.
- Develop clear policies, regulations and incentive mechanisms that leverage learnings from leaders in the field, backed by objective high-level business cases where applicable.
- Coordinate across national and regional borders when setting up governance models and managing governing entities (such as universities, AI-funding entities and partnerships).
We believe the first steps in this direction have been initiated and driven by a small group of like-minded countries. Given the significant transformation scope of AI, a strong foundation needs to be laid by each country to ensure it is not left behind in this global race.
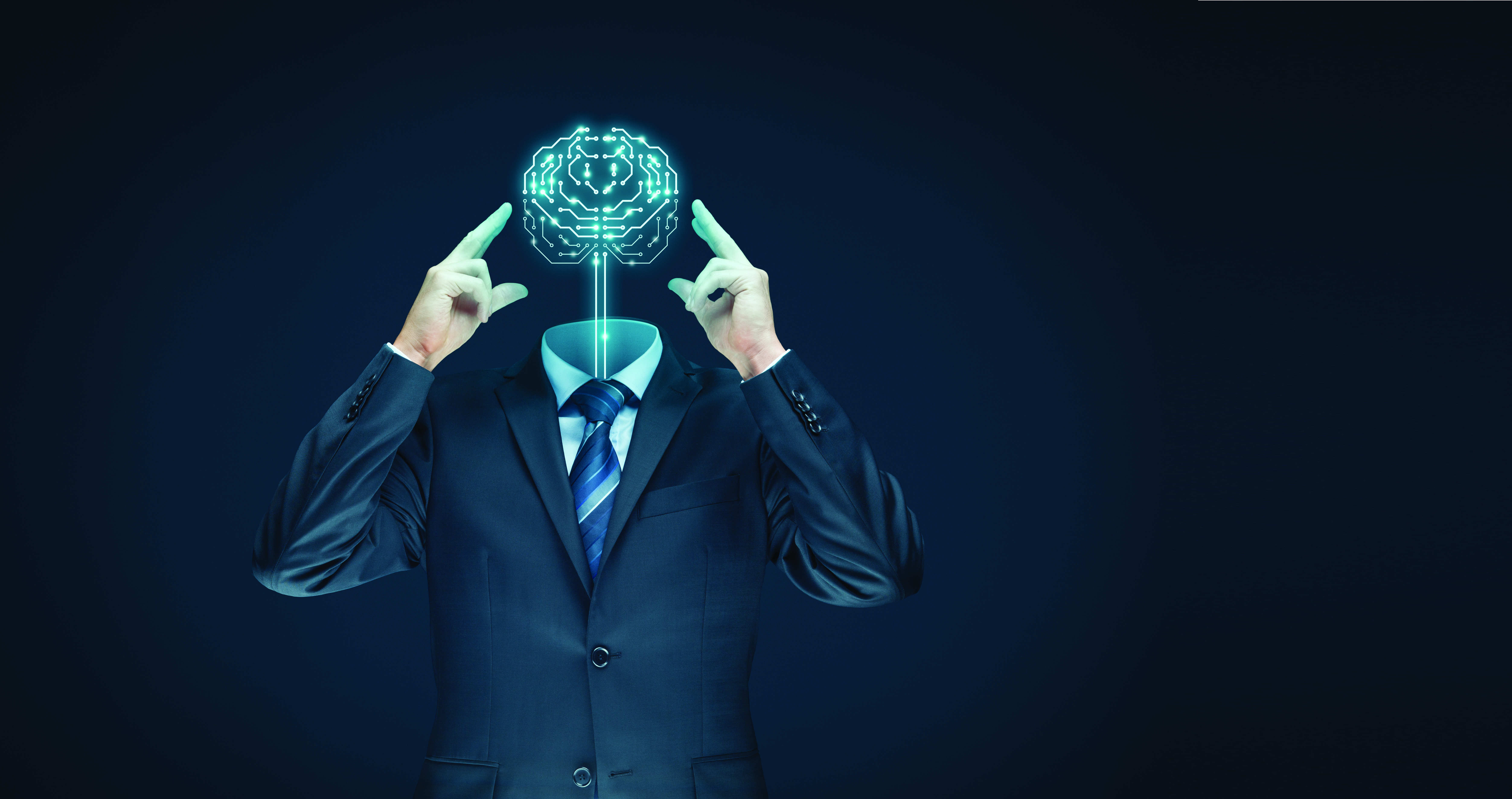
8 min read • Technology & innovation management
The global AI arms race - How nations can avoid being left behind
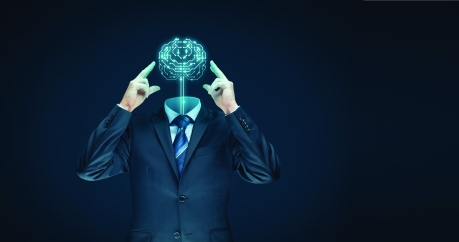
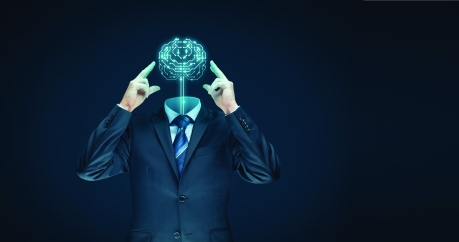
Artificial intelligence promises to have the same disruptive effect on today’s global economy as electricity in the 19th century. This has led governments to focus heavily on how they can unlock AI’s potential within their countries. Based on in-depth research, this article outlines the current winners and losers, exploring what it takes to win in the global AI arms race.
Artificial intelligence (AI) is widely accepted as having the potential to transform virtually every industry and create significant economic value. While businesses play out their AI strategies within their various national and international ecosystems, an often-overlooked dimension is how innovative regulation and governmental support can unlock AI’s full potential. Governments and regulators play a critical role in fostering, or hindering, how technology benefits accrue for their citizens, and the role of academia and research institutes is also crucial for AI technology to develop. In this article we look at the successes and failures of national AI strategies, and identify some key priorities for competing nations, including the role of government as well as private and public stakeholders.
Which are the current leading countries in AI?
There are several country rankings of AI strength across the world. Those that focus on metrics such as patents and research publications tend to list China first, followed by the US, with third place disputed between European and Asian countries including South Korea, Japan and India.
However, taking a broader approach using a composite AI-readiness index (from Oxford Insights) that factors in governance, skills and education, infrastructure and data, and government/public services reveals the top three countries to be Singapore, the UK and Germany. (See Figure 1.)
In order to understand better which national polices and strategies are important for success, on behalf of the government of Singapore we recently conducted a comparative review of 10 of the top 20 countries. These 10 countries were selected on the basis of having comparable GDPs to Singapore (around USD 50–60k per capita) and similar innovation capacities (based on the Global Innovation Index). For these 10 countries, we used our published public reports, global AI indices and our own analysis to cover over 50 AI-related metrics, including enablers and impacts. The impacts were classified across four key dimensions:
- AI start-up activity, including investment in AI startups per capita, number of AI start-ups per capita, and number of AI unicorns per capita
- AI-related jobs, including percentage of AI-related jobs in the economy
- Adoption of AI by private industry, including level of AI adoption among top 10 listed companies, extent of AI government support for small and medium-sized enterprises (SMEs), and funds committed to AI adoption
- AI knowledge/skills capability, including H-index (i.e., quality/quantity) in AI publications, weighted citation index in AI, university-led/-funded incubators in top five universities, and AI patents filed per capita
The study provided valuable insights into what is, and is not, important for countries to compete successfully in AI. A toplevel overview of strengths across the impact dimensions is provided in Figure 2.
- It can be seen that there is quite some difference in strength and focus across the four dimensions. Leading countries have broad coverage across the impact metrics, with a stronger bias towards one or more, based on their context. For example, Germany is the strongest in the sample in private sector adoption of AI, driven by government support of AI-based industrial digitization focused on SMEs and university AI R&D programs that have strong bias for commercialization.
- Canada, the US and Singapore all have strong scores in AI knowledge/skills capability.
- Israel, Singapore and the US have mature and welldefined AI governance frameworks with clear regulatory set-ups, well-established national and regional AI offices, and strong cyber-security and AI risk management practices.
What are the policy levers that have the greatest impact?
Focus on the key enablers
The study analyzed which specific “enablers” correlated highest with each of these impact dimensions. In other words, which are the enabling policies that really make the difference? This is shown in Figure 3.
It can be seen from this analysis that some common assumptions about policy levers are not correct, in particular:
- Growing AI start-up activity: National AI governance is as important as funding
Government funding is part of the solution. However, the support required goes beyond finance, including mentoring, facilitating international collaboration, and a drive for talent development and retention. We found that the most likely contributing factor that encouraged start-ups was the maturity and influence of national AI governance.
- Private sector AI adoption: A triple-helix partnership is more important than having a data-sharing framework and ethical policy
Fostering private participation in AI has little to do with whether data can be obtained from the government or has a strong ethics policy. What private participants are looking for to kick-start the AI journey is the so-called “triple-helix” partnership, with academia, government and the private sector all playing their roles.
- AI knowledge/skills capability: Investment in R&D supported by mature national AI governance is key
Although international collaborations and the triple-helix model play a part in knowledge and skills development, we found that a more direct approach based on government investment in AI R&D and a mature and influential national AI governance approach are even more important.
Build a virtuous AI talent cycle
The analysis showed that ensuring local and global AI talents were developed, attracted, and retained was central to national AI success. We found that:
- The US has almost 40 percent of the global AI talent, while Israel and Singapore have the highest scores for AI workforce and talent development from a tertiary education perspective.
- Countries such as Germany, the US and Canada have been able to attract new AI talent into their economies over the last few years, while China, Israel and Singapore have been unable to retain and grow their talent pools.
A country’s national AI talent strategy should therefore aim to create a virtuous cycle in which an AI ecosystem fosters AI talent concentration, which, in turn, drives private innovation and economic development, boosting the AI ecosystem further. Based on our observations, a coordinated policy effort across academic institutions, public sector support and incentives for private players has the highest likelihood of being successful in creating this virtuous cycle.
Adopt robust methodologies for assessing AI investments
The analysis also showed that the most successful countries had robust methodologies for assessing how much to invest in different aspects of policy. For example, in deciding the size of the incentive required to support increased adoption of AI in the private sector, a business case can be developed studying the economic potential of AI skills by sector and function, netted against potential costs. The approach is illustrated in Figure 4.
Adopt a model across multiple dimensions, anchored in ecosystem thinking
Finally, the study showed that countries with AI strategies across multiple dimensions, including clearly defined national AI visions, investment in AI benefits, and protections for data usage, had higher likelihood of success compared to countries that focused on fewer dimensions.
However, it is essential to realize that the path to success is not the same for all countries. The national context varies tremendously from country to country, and the way in which AI will transform industries and countries is not yet obvious. For example, in the current state of development, AI is at a crossroads, with at least one major dichotomy in terms of how it will progress:
- Global, centralized AI driven by Big Data platforms (e.g., Google and Amazon) that accumulates vast amounts of information about each individual to engineer a digital environment
- Decentralized AI, with significant privacy protections and in which data is controlled by the individual, communities or national governments
This is in many ways analogous to the adoption of electricity in the 19th century, for which progress was neither linear nor predictable, and success depended not just on the technology itself, but also on how it became integrated with broader societal changes. (See Box 1.)
Box 1: AI as the new electricity – A lesson from history
In many ways, artificial intelligence is the new electricity. In the late 19th century, as electricity was being popularized, it became apparent that it required a new framework, as it was different to virtually every other commodity. Whoever mastered the engineering and economics of electricity would indelibly change the course of history. The original model for electrification was limited, as it relied on DC current and local generation. Electrification took off with the switch to the AC current, which allowed long-distance power distribution from centralized generation. This shows that progress with transformative technologies was neither linear nor predictable. The more customers the electricity company had, the more efficiently it could run its generators and the cheaper it was to provide power, and even more people and industries could be served and transformed. The benefits of electrification did not accumulate for early adopters, but for those who had identified how electrification could transform their societies within its existing context.
AI will similarly transform entire industries and countries, and the path will again not be obvious and results will probably not be linear. In its current state of development, AI is at a crossroads similar to electricity in its early years.
This means national policy makers need to adopt AI strategies that are anchored on consideration of the broader local and global ecosystem and tailored to the national context, and that consider multiple actions that may need to take place within different parts of the ecosystem. There is no single path to success
Insights for the executive
Widespread AI-driven disruption is coming, whether we embrace this technology nationally or not. Just like the adoption of electricity, it is enabling winners and losers within the global race for greater productivity. Achieving a competitive edge will have significant consequences, which means governments need to make the right decisions. Essentially, national AI adoption should be designed and rolled out across multiple dimensions with an ecosystem-based philosophy of creating smart people, smart government, enabled industries and companies, and smart technologies. In summary, governments need to consider the following:
- Design and articulate national AI objectives based on their assessment and relevance of likely future scenarios.
- Develop clear policies, regulations and incentive mechanisms that leverage learnings from leaders in the field, backed by objective high-level business cases where applicable.
- Coordinate across national and regional borders when setting up governance models and managing governing entities (such as universities, AI-funding entities and partnerships).
We believe the first steps in this direction have been initiated and driven by a small group of like-minded countries. Given the significant transformation scope of AI, a strong foundation needs to be laid by each country to ensure it is not left behind in this global race.