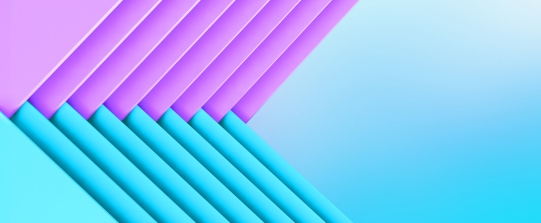
In our evolving digital landscape, artificial intelligence (AI) is driving innovation across multiple sectors. It has now intersected with embedded finance, which weaves financial services into nonfinancial platforms, enhancing user experiences and streamlining processes. AI has the potential to lift embedded finance to its fullest, offering tools to combat fraud, curate personalized experiences, and manage risks. In this Viewpoint, we shed light on the interplay between AI and embedded finance, sharing current applications, future trajectories, and the manifold challenges.
Embedded finance, the seamless integration of financial services into nonfinancial platforms, marks a monumental shift in financial services. Its transformative potential is undeniable. The global embedded finance market is projected to reach US $350 billion by 2025. Its symbiotic integration with AI, another transformative force, is accelerating embedded finance’s momentum. While AI’s historical contributions to finance, such as algorithmic trading and fraud detection, remain foundational, it is poised to support embedded finance’s growth and address some of its challenges.
AI is evolving into the linchpin of the embedded finance revolution, thanks to advancements in generative AI (GenAI), large language models (LLMs), and deep learning. GenAI can create offerings tailored to individual users. LLMs can handle customer queries, manage data, and predict market trends with greater accuracy (see Figure 1).
AI has moved from facilitating to creating, innovating, and shaping finance in real time. Its profound impact on embedded finance is rapidly expanding, and some might argue that we are only beginning this journey.
A plethora of applications already exist, which may predict AI’s ability to drive wider adoption of embedded finance:
-
Square, a financial services platform, leverages machine learning (ML) for underwriting and extending loans to small businesses bypassed by conventional banks.
-
E-commerce platform Shopify embeds the AI prowess of payment service processor Stripe, offering individualized solutions, which amplifies the sales potential for its merchants.
-
Retail behemoth Amazon relies on AI to dissect data and curate personalized loan offers anchored in sales history.
These examples only scratch the surface. Figure 2 reveals the spread of AI’s influence across embedded finance.
AI’s potential to expedite and amplify embedded finance cannot be understated. This synergy is not just beneficial; in many respects, it is indispensable.
The essence of embedded finance is its comprehensive integration with nonfinancial platforms, ensuring that users can access and utilize financial services without disrupting their primary engagements. AI elevates this feature; its advanced personalization capabilities discern user needs before they become apparent. For example, with data analytics, AI could instantly offer a specific financial product, like an installment plan or short-term loan, to a user shopping online for a high-end electronic product.
The world of finance revolves around trust. While traditional financial institutions have built this trust over decades, embedded finance solutions don’t have this luxury of time. This is where AI comes to the fore. Its predictive analytics and fraud-detection capabilities verify that financial transactions are secure, transparent, and in the user’s best interest. An e-commerce platform offering financial services will gain trust faster if its AI-backed systems demonstrate transparency, minimize errors, and preemptively address user concerns.
Moreover, AI’s scalability, especially with advancements like LLMs and GenAI, means it can adapt in tandem as embedded finance grows and diversifies. It can process larger data sets, interact with other systems, handle increasingly complex user queries, and cater to diverse financial needs without needing additional resources.
EMBEDDED FINANCE: BEFORE & AFTER AI
Embedded finance could substantially accelerate its growth through its marriage to AI, whose wide-ranging applications facilitate its adoption and deepen the intimacy of the user experience. Three broad application categories stand out:
-
Smoother customer journeys. AI can go beyond processing to amplify the value proposition for customers. It brings agility to applications, accelerates underwriting, and refines credit assessments, which culminates in swifter, more accurate decisions. This streamlined experience bolsters customer satisfaction and fosters loyalty. Augmented by AI-powered chatbots, customer support becomes more fluid, queries are promptly addressed, and the reliance on human intervention diminishes, creating an efficient customer experience.
-
Improved risk management. AI has elevated the accuracy of risk assessments, which redefines financial inclusivity, making services accessible to those previously marginalized by conventional financial systems — whether due to demographics, unconventional credit histories, or perceived risk. Realizing the untapped potential in these segments translates to substantial revenue opportunities. AI can also enhance portfolio management and optimization on the side of both the provider and the customer.
-
Faster, more accurate fraud detection. AI can dissect vast data sets to unearth anomalies. Continuous scrutiny of transactional data, coupled with behavioral analytics, fortifies the system against fraud. Given that control around customer acquisition often rests with the embedding entity, this vigilant layer becomes indispensable to mitigate provider-side risks.
Table 1 shows some use cases and illustrates improvements made possible through AI. We see the trend of supercharging embedded finance with AI continuing as new and more innovative use cases emerge in the future (see Figure 3). We anticipate developments in all four categories shown in Figure 3, which may include:
-
Biometric authentication (customer journey). A commitment to security is paramount, as financial institutions essentially outsource their customer acquisition to embedders. Advanced biometric authentication, powered by AI, stands at the forefront of the shift toward user acquisition through the embedder. This approach, which incorporates facial, fingerprint, voice, and iris recognition, promises a stronger level of security for all financial transactions.
-
Sentiment analysis in wealthtech (risk management). AI-driven sentiment analysis harnesses natural language processing (NLP) to extract opinions and emotions from social media and news, offering a predictive edge for market trends and investment strategies. Coupled with LLMs that formulate summaries and reports, this technology is set to revolutionize the wealthtech sector, both within the realm of embedded finance and outside of it.
-
Customized financial advice (personalization). The traditional paradigms of robo-advisors and financial advisors are poised for a major shift. AI can tailor financial advice to an individual’s unique circumstances, including behavior, risk tolerance, income, and spending habits. This transcends the limitations of traditional portfolio approaches.
-
Automated debt collection (risk management). AI makes error-prone, human-led debt collection organized and efficient. It uses NLP to communicate with debtors and curate feasible payment plans.
-
Regulatory compliance and blockchain (risk management). AI parses through regulations to pinpoint requirements, ensure adherence, and highlight discrepancies. The increase in blockchain transactions requires scrutiny, which AI can accomplish. Its ability to detect patterns and anomalies plays a pivotal role in combating fraud.
-
Voice-activated transactions (fraud detection). The integration of audio processing and LLMs transforms how we interact with our finances. As audio-activated transactions become a reality, AI can employ voice biometrics to authenticate and securely verify users.
-
Dynamic risk management and behavioral analysis (risk management). Real-time risk management is essential. By processing vast amounts of market data, AI can instantly evaluate and offset risk exposures and issue timely alerts for emerging threats. In tandem, AI’s behavioral analysis capabilities enhance security and user experience by identifying any deviations in typical user patterns.
-
Advanced forecasting for financial planning (personalization). As households grapple with soaring costs, advanced forecasting emerges as a beacon for financial planning. AI offers sophisticated projections for income, expenses, and savings by generating actionable advice. AI’s focus on personal finance management prepares users well for their financial futures.
These advancements paint a promising picture for the financial sector, ensuring that services are more efficient, secure, and deeply attuned to individual needs.
EMBEDDED FINANCE’S LLM DIMENSION
Card-issuing platform Marqeta tapped OpenAI’s LLM to speed up its launch of embedded finance applications. The result, Marqeta Docs AI chatbot, is a GenAI tool that allows customers to quickly navigate the site. Customers can ask questions and collect information and gain more knowledge of the platform’s offerings. The tool also addresses the process of embedding different categories of payment services. Marqeta is an excellent example of how embedded finance and AI are starting to merge and leverage LLMs.
Our exploration of AI’s transformative impact includes another evolving dimension: LLMs such as GPT-4. These models bring the potential to drastically elevate embedded finance through their advanced language-generation and comprehension abilities. Established companies, especially those focused on embedded finance, can gain a competitive edge by adding GenAI capabilities to their operational fabric, including:
-
Automated customer service. LLM-powered chatbots could provide more insightful, contextually aware customer support. Chatbots decode complex user queries with remarkable accuracy, ensuring an efficient and informed transaction. LLMs do more than facilitate transactions: they offer real-time assistance, anticipate user needs, and suggest alternate payment methods.
-
Personalized financial advice. Personalization is at the heart of modern consumer interaction. LLMs, with their ability to process vast amounts of data and generate human-like language, can offer financial advice that feels truly bespoke. These models can sift through purchase histories and preferences to help users confidently navigate products and services. LLMs support embedded lending with insights into optimal repayment strategies and educate users on proactive loan management, giving them a transparent, manageable experience.
-
Regulatory compliance. LLMs can further refine this task, simplifying the regulatory process with their aptitude for understanding detailed documents. While final decisions will invariably involve human discretion, LLMs can make the process faster and more robust. In embedded insurance, which often involves numerous policy details, LLMs, especially when coupled with knowledge graphs, can guide users through the intricate clauses, helping them comprehend coverage details.
It’s evident that adding LLMs is not just an incremental step. It’s a leap into a future where finance isn’t just about numbers, it’s about delivering a thoughtful, personalized user experience. As embedded finance continues its ascent, harnessing the capabilities of LLMs could be its next big opportunity.
CHALLENGES & ETHICAL CONSIDERATIONS
As we continue our journey, it’s crucial to address the challenges and concerns that inevitably accompany groundbreaking innovations. Despite the benefits, there are some real considerations that consumers and providers need to grapple with:
-
Trust and transparency. The nature of embedded finance’s integration into nonfinancial platforms faces skepticism and intensifies concerns about data privacy and security. Furthermore, fraud has become a pervasive theme, making trust even more critical. In a digital economy, as highlighted by the recent surge in AI-enabled frauds, the onus is on businesses to maintain this trust.
-
Data security in the age of AI. AI can strengthen cybersecurity, but its power to process vast streams of data instills apprehension. Financial platforms need to articulate their protective measures, especially given the predicted surge in payment-fraud losses, expected to exceed US $40 billion by 2027.
-
The “black box” dilemma. AI decision-making can jeopardize trust if those decisions lack transparency; fairness and potential biases are significant concerns, especially when financial decisions are involved.
-
Tackling algorithmic bias. Bias in AI reflects societal issues; embedded finance’s mission to democratize access is undermined if biases persist. Efforts like explainable AI (XAI) can offer transparency.
-
Regulatory, compliance, and legal risks. The transition from analog to digital brings regulatory challenges. The trend points toward a localized approach to data storage and sharing, which is hard to reconcile with the global nature of the Internet. The emergence of local privacy regimes poses the risk of increased fraud as an unintended consequence. Moreover, standards like the Payment Card Industry Data Security Standards (PCI DSS) add operational burdens.
-
Operational risk. Operational challenges are exacerbated by the rollout of real-time payments alongside AI tools, which creates an environment conducive to fraud. The continuous evolution of data security demands businesses to adapt, but without clear industry standards, the pathway remains ambiguous.
-
Model risk, including hallucinations. AI, especially deep learning models, might produce unintended outputs or “hallucinations.” Financial decisions are prone to serious risk, as incorrect predictions can lead to significant implications.
-
Reputational risk. Failure to manage the above can harm a company’s image, especially in the sensitive realm of finance. A significant fraud event, caused by AI vulnerabilities, can erode customer trust and impact a company’s standing.
As we reflect on the promise of AI, it’s clear that the path forward will require balancing innovation with ethics, efficiency with transparency, and capability with responsibility. As embedded finance evolves, championing these values will be essential for widespread acceptance and success.
RECOMMENDATIONS FOR FINANCIAL SERVICES PROVIDERS & FINTECHS
Financial services providers and fintechs have pivotal roles that give them unique opportunities. Here are several recommendations based on our exploration of embedded finance and the intertwined roles of AI and LLMs:
-
Integrate, don’t just incorporate, the technology. AI is the new financial reality. Financial services providers and fintechs must integrate it holistically, making AI-driven tools and insights intrinsic to their operations. Goals should include refining user experiences, automating processes, and opening new avenues to personalized services. Providers should collaborate with potential embedders to create a more seamless customer experience.
-
Forge meaningful partnerships with AI pioneers. While financial services expertise is paramount, an in-depth understanding of AI is equally crucial. The realm of AI is vast and complex and relies on the expertise of specialists to support a technically sound and ethically aligned integration, especially given the overall importance of transparency and fairness.
-
Make data security a prime directive. Today, data is often equated with currency. However, it’s more than an asset in the world of embedded finance; it fuels ML models. This data is critical to tailor services and its protection is nonnegotiable. Adequate security thwarts breaches while building trust. Firms must establish robust encryption, stringent access controls, and a commitment to respecting user privacy.
-
Promote transparency and address algorithmic bias. The black box nature of certain AI models and the potential for algorithmic bias make it vital for financial services providers and fintechs to champion transparency. Investing in XAI will show that every financial decision made by an AI model can be understood, justified, and trusted by the end user.
-
Continual learning and adaptation. Finance, technology, and user expectations are in a state of flux. Providers should commit to learn and adapt, regularly updating their models in line with new data, trends, and best practices.
The convergence of AI and embedded finance presents a transformative opportunity for the financial sector. Considering these recommendations can position financial services providers and fintechs at the forefront of this revolution, where they can drive innovation while upholding trust, transparency, and ethical standards.
CONSIDERATIONS FOR EMBEDDERS
It is vital for nonfinancial businesses, or “embedders,” to grasp the nuances and opportunities. These businesses stand at the intersection of traditional industries and innovative financial solutions, ready to redefine customer experiences. Embedders must meticulously select their partners, as each offers distinct advantages:
-
Finance is heavily choreographed by regulations. From data protection to licensing, regulatory norms shape the foundation of financial services. Traditional banks, given their long-standing experience, might appear to have an upper hand. However, innovation and compliance can coexist, provided there’s a deep understanding of the environment.
-
Businesses venturing into sectors like healthcare or employment face intense regulatory challenges. Whether it’s adhering to regulations or ensuring compliance, the journey is complicated. The confluence of legal acumen and industry knowledge becomes crucial. Collaborating with industry stalwarts can demystify regulatory complexities.
-
The brilliance of AI isn’t limited to enhancing user experiences or predicting trends. Its prowess in automating compliance processes demonstrates its versatility. By comparing vast data reservoirs against regulatory standards, AI can make compliance fast, accurate, and efficient. Fintech visionaries Plaid and Forter leverage AI to validate transactions swiftly, simultaneously establishing legitimacy and thwarting potential fraud.
-
Embedded finance is filled with possibilities and challenges. By strategically selecting partners, understanding regulations, and leveraging AI’s multifaceted capabilities, embedders can add trust, innovation, and unparalleled customer experiences to their offerings. As the landscape evolves, the fusion of technology, finance, and traditional business promises a future where boundaries blur and possibilities are limitless.
FUTURE TRENDS & PREDICTIONS
Embedded finance, fueled by AI, has gained enough momentum to place it at the forefront of the next technological revolution. Here’s a closer look at some anticipated trajectories and implications:
-
Peak personalization. AI analyzes user patterns, preferences, and histories and offers financial solutions to meet individual needs. Imagine insurance policies determined by lifestyle choices or investment plans attuned to personal aspirations.
-
Redefined efficiency. Automation will streamline processes, drive efficiency, and reduce costs. Wait times for loan approvals or dispute resolutions will shrink, while precision soars.
-
Financial inclusivity. Individuals historically sidelined by rigid conventional banking criteria will have better access and options. Alternative data metrics and innovative business models will make financial inclusivity a reality.
-
Demystified financial algorithms. XAI will shed light on the intricacies of financial algorithms. Customers will understand the reasons behind the financial decisions they receive, cultivating trust.
-
Future foundations. AI, LLMs, blockchain, and edge computing will augment embedded finance, offering secure, swift, and scalable solutions. The confluence of these technologies will form the backbone of future financial ecosystems.
-
Strategic collaborations. Financial giants will forge alliances with fintech and AI innovators, combining traditional wisdom with modern agility to elevate embedded finance solutions.
-
Market dynamics and maturation. Acquisitions will become commonplace. The race to scale, coupled with access to vast data troves, will determine the victors.
-
Regulatory shifts. Governments will establish frameworks ensuring data sanctity, algorithmic fairness, and consumer protection. These regulations might also trigger industry consolidations.
-
Power-play dynamics. The current ecosystem seems harmonious, but banks, fintechs, or colossal tech corporations may emerge as predominant players.
-
Application programming interfaces (APIs). APIs enable embedded finance’s straightforward integrations. Expect a proliferation of robust, secure, and intuitive plug-and-play APIs, anchoring flawless cross-platform functionalities.
-
Open banking. Embedded finance amplifies open banking, accentuating collaborations, data sharing, and relationships between banks and third-party developers.
-
Sectorial expansion. The ripple effect of embedded finance won’t be confined to traditional sectors. The integration of finance will present myriad opportunities in healthcare, real estate, and education.
The horizon of embedded finance, pushed further by AI, promises a world where finance isn’t just a sector but an integral part of our experiences, incorporated effortlessly into daily lives, decisions, and aspirations. Inclusivity, personalization, and unparalleled user experiences will characterize the future of embedded finance.
Conclusion
SHAPING THE FUTURE OF FINANCE
Connecting AI and embedded finance is not just an enhancement to accelerate growth — it’s a trend poised to redefine the contours of digital financial services. AI complements embedded finance and amplifies its core strengths by:
-
Promising a user-centric environment that emphasizes trust and transparency
-
Contributing to value-focused personalized products and services
-
Automating processes to save time and minimize frustration
-
Building trust swiftly by prioritizing security and derailing fraud
-
Redefining financial inclusion and improving access to products and services
-
Interpreting standards and adhering to regulatory frameworks
AI promises to make embedded finance faster, deeper, and more meaningful. The fusion of these two forces heralds a future where financial services are omnipresent, intuitive, and most importantly, centered around the individual.