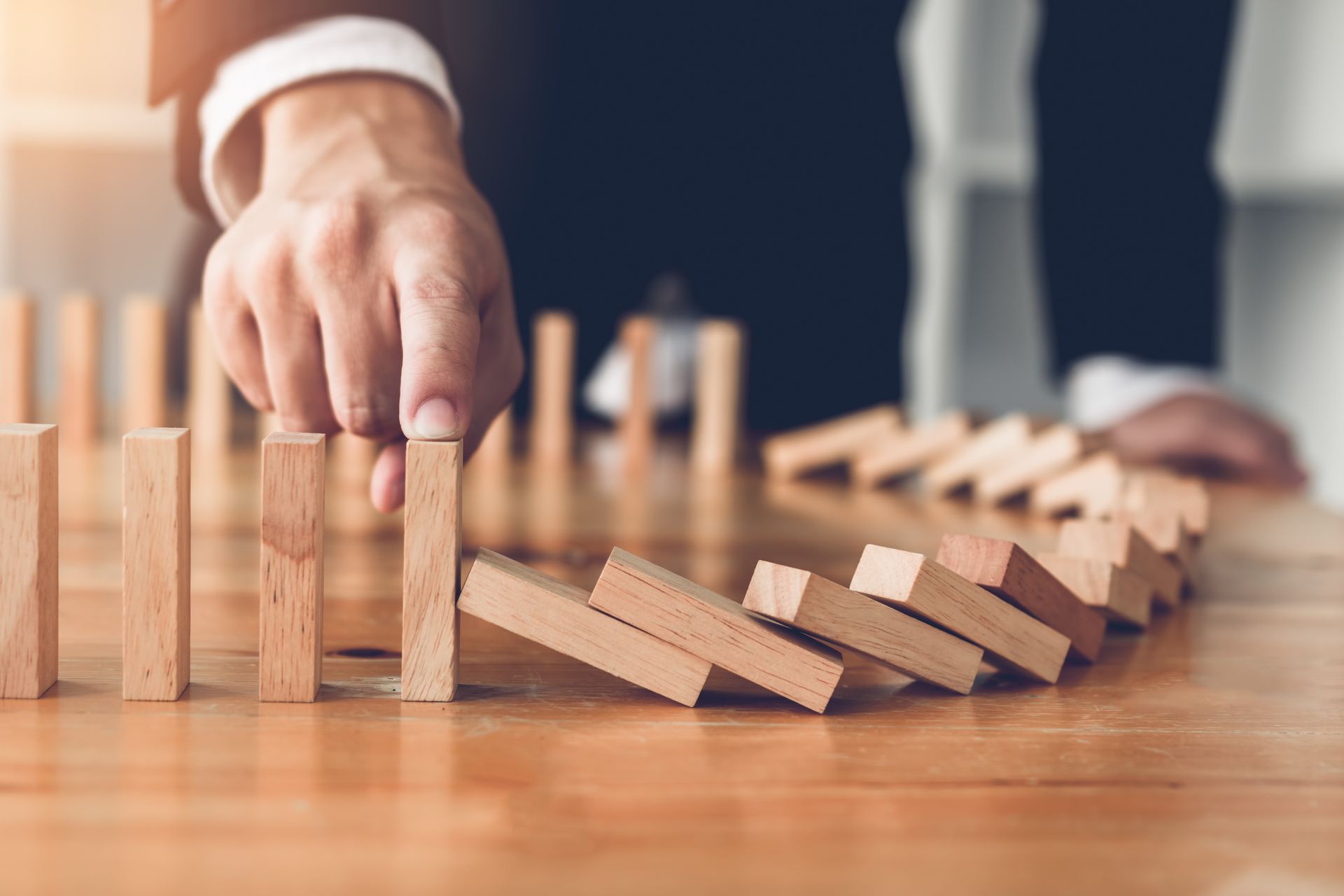
Responses from large companies to the COVID-19 crisis have brought into sharp focus serious shortcomings in current risk and business continuity management approaches, such as lack of suitable data and intelligence, inadequate appreciation of risk velocity, and not enough agility to adapt to rapidly changing situations. Well before the crisis, Arthur D. Little (ADL) pioneered and deployed a new business resilience management approach with, at its core, an innovative predictive analytics tool powered by machine-learning technology to address these shortcomings. With this approach companies can become truly resilient and “anti-fragile”. Business operations with critical infrastructure, such as utilities, transport, telecoms, healthcare and chemicals, will need to move to this “next-generation” business resilience approach for the post-COVID world.
The shortcomings in current approaches to risk and business continuity management
The COVID-19 crisis is proving to be the ultimate test for effectiveness of companies’ crisis management and business continuity systems. Although many enterprise risk management systems already had “pandemic” as one of the major risks on their corporate risk registers before COVID-19, Asian companies that had experienced the SARS outbreak were the most able to respond as effectively as they had planned. Based on the lessons from 25 CEO best practice-sharing meetings, which we held a few weeks into the crisis, we know that there are some common shortcomings:
- Getting reliable intelligence: Difficulties in understanding and aligning all parties around real facts and intelligence.
- Keeping up with the velocity of the crisis: Plans took too long to implement and were constantly being overtaken by events.
- Coping with uncertainty: Companies needed to be much more agile and flexible than anticipated as events unfolded daily, and even hourly.
- Understanding the whole ecosystem: Companies had not sufficiently considered the impact of the crisis on the suppliers and partners in their ecosystems, which then hit their own operations. This is of growing significance as companies’ operating models increasingly adopt an ecosystem-based approach.
The fundamental problem many companies face is that their risk management approaches, and ensuing business continuity plans, are essentially based on a rearward view of risk, rather than one that is forward looking and dynamic. They are based on establishing robust risk processes, assuring best practices are applied and continuously improving in response to measurement and review – a traditional management approach.
However, if we accept that the world is increasingly unpredictable, complex, and therefore uncertain, we see that the traditional approach does little to avoid top leadership from periodically being caught unprepared – as demonstrated with tragic clarity by COVID-19. Even before COVID-19, CEOs in leading organizations expressed concerns over “being caught by surprise” by project/operations failures and wanting to move to forward-looking risk capabilities. If the management approach is not changed, this will happen again and again. To address this requires some fundamental shifts in approach:
- From plan/do/check/act to sense and respond (agile working).
- From a process compliance-driven culture to one in which risk management is embedded into activities.
- From backward-looking review to forward-looking prediction using firm baseline data.
- From oversimplifying complexity to embracing unpredictability.
- From an experience-based to a data-driven approach.
We know from discussions with our clients (including running “blue sky EXCOM workshops”) that many companies have recognized the need to make this shift. However, it is only with recent developments in data analytics and AI/ML technology that we now have the tools to make it a reality. Before and now during COVID-19, ADL has pioneered and applied such an approach and, accordingly, invested in recruiting a world-leading capability in data science and data engineering.
Our next-generation business resilience approach
By combining the expertise of our risk specialists, digital team and industry experts, we have worked with organizations to develop and implement an innovative next-generation business resilience approach that is forward-looking and agile. At its core is a powerful ML-driven data analytics tool that collects and integrates internal and external data to enable real-time monitoring of a set of key risk indicators (KRIs).
Three aspects are fundamental to the approach:
- Forward-facing practices: Setting and monitoring a set of customized KRIs (leading indicators) based on a robust bow-tie analysis of causes and effects, which are calibrated to provide a “red flag” prior to a risk event occurring; the calibration should be directly related to an organization’s risk tolerance. KRI monitoring is informed by ML-driven data analytics.
- Dynamic prioritization: Regularly tuning risk-control priorities to take account of emerging risks. For this, understanding risk velocity – how quickly an organization will feel the impact of a risk event occurring – is key. Our data analytics tool enables potentially high-velocity emerging risks to be identified more easily and monitored in real time through KRIs.
- Adaptive response: Moving away from formulaic management responses based on static risk registers towards an active decision-making regime based on constantly refreshed KRI data. Our data analytics tool provides tailored dashboards designed to support decisionmaking at operational and top leadership levels.
The above approach can sit within an established “three lines of defense” structure, providing insight and assurance at the required levels, from first-line managers with new operational dashboards to the executive level, at which critical information informs strategic, real-time decisions.
How our ML-driven data analytics tool supports the next-generation approach
Our leading-edge data analytics tool is an essential enabler to make the next-generation approach practical and feasible. One of the biggest challenges of KRI-based risk management is that the required input data is rarely complete, often in different forms, and too large and complex to be analyzed manually.
Our use of ML technology allows for greatly enhanced prediction and forecasting. This means the tool can integrate data from a huge number of diverse data sources, combine it with companies’ internal data, and provide context-specific, customized forecasts and predictions to support risk-based decision-making. Predictions are refreshed continuously as new data is ingested , which is essential in situations in which changes are rapid and knowledge is still evolving week by week, or even faster (as is the case with COVID-19). The model is ideally suited to situations in which data is uncertain or incomplete. In geographies for which detailed data is not available, the model can draw inference from similar areas with better data coverage. In one recent use case before the COVID-19 pandemic, we ingested hundreds of gigabytes of hourly global weather-forecasting data and used this with machine learning to model the impact on critical transport infrastructure in real time. This provided a forward-looking, location-specific risk prediction to help drive management intervention.
The tool has a number of key features that enable nextgeneration risk management:
- Ability to ingest both hard data from government, authorities, intelligence agencies and the like (e.g., one recent application used approximately 160 unique sources) and “soft” data from sources such as Google searches and interaction, mobility, and demographic data.
- Combines external data with companies’ internal business and operational data to enable customized, context-specific analysis, aggregation and interpretation.
- Provides understanding of KRIs that need to be monitored, ensuring that alerts are raised in real time as thresholds become at risk of being breached.
- Integrates knowledge from companies’ in-house experts and specialists.
- Allows what-if simulation modeling to assess scenarios and stress-test responses.
- Provides customized, user-driven dashboard graphics that can be tailored to different user personas, including top leadership and operational staff.
We have applied the approach in the transport and pharma industries – see the below case studies.
Case study 1 – Proof of concept (POC) for rail service disruption risk management
For one of the world’s leading railways, ADL has successfully implemented a novel approach for improving risk management of service disruptions caused by tree falls. Tree falls, often resulting from adverse weather conditions, are a significant cause of disruption and delay.
We developed and implemented an ML-based analytical tool to help operations predict where tree falls were most likely to occur. We built a digital model of the railway line and surrounding topography and surface data, including number and proximity of trees and historical data on service interruptions. We used machine learning to understand past weather patterns and their impact on tree falls and service disruption. By continuously ingesting real-time data on weather conditions, including day forecasts, the tool presented a detailed visual map to indicate dynamic risk of where disruption was most likely. This has enabled the company to minimize service disruptions and reduce maintenance costs. The project was delivered in eight weeks and provided a web-based dashboard for the company to use.
Case study 2 – Supporting business continuity for a pharma company during COVID-19
We were engaged by a mid-sized pharma company to rapidly develop an integrated, global approach for managing the continuity of active and planned clinical trials in light of disruption caused by COVID-19.
Working with the company’s in-house team, we developed an approach for collecting and integrating data (internal and external), aggregating and correlating it into focused dashboards, and setting up a framework to make or recommend relevant clinical operations decisions. The model is constantly updated and refreshed as the work continues.
After setting up the initial framework and dashboard within three weeks, we have since supported the company’s operational and leadership teams in making decisions on issues such as home-based infusion, site access, database lock, monitoring and screening. Further applications are being discussed.
Benefits of the next-generation business resilience approach
The next-generation approach unlocks huge potential benefits to companies, especially those with critical infrastructure, such as utilities, transport, telecoms, healthcare and chemicals:
- Enables an integrated business resilience approach to be adopted. By having a powerful data analytics engine at its core, companies can effectively integrate their risk management and business continuity efforts, which leads to better and more dynamic decisions around prioritization and allocation of resources and capital.
- Keeps the executive updated with business-critical information in near-real time: dynamic, forward-looking risk management, “sensing, feeling and reacting” to signals across both internal operations and external supply chains to ensure the business is fully prepared for the threat.
- Helps organizations to move towards becoming “antifragile”: gaining strength as they are exposed to more risks, with ML allowing them to better adapt and build more resilience.
- Stress-tests business continuity plans. This is especially important as plans need to keep up with ongoing changes in operating models.
- Improves the organization’s ability to anticipate and take action through identifying weak signals and early risk indicators of key trends.
- Enables better linkage between operational- and strategiclevel decision-making, using consistent data.
- Helps management teams interpret large and complex data sets more quickly and effectively for management decision-making.
We believe moving to next-generation business resilience will be essential for companies in the post-COVID-19 world.
Implementing the approach
Our approach begins by rapidly solving a specific key risk management problem as a hybrid proof of concept/pilot. We can typically develop and implement a specific solution in a few weeks (the case studies above were delivered in eight and four weeks, respectively). The key to success is providing regular demonstrations of the emerging end product, which we take feedback on and tailor to hand over something that is “end-user ready”. We believe in “show, don’t tell”. Building on the proof of concept, we work with clients to agree priorities for broader application across their enterprises.
If you would like to hear more about next-generation business resilience management, please contact the ADL office near you.