
While ChatGPT’s rapid rise has fascinated the world, it is in reality just the tip of a gigantic iceberg. We have entered a transformative era of artificial intelligence (AI) in which no sector will remain intact, and the speed at which this revolution is unfolding is dizzying. In this Viewpoint, we address some crucial questions about generative AIs.
We explore the emergent properties that no one expected and how they may pave the way to artificial general intelligence (AGI), and address the corresponding uncertainties, examining how companies can seize opportunity and mitigate risk.
LLMs: THE “BRAIN” OF GENERATIVE AI
19 January 2023, my WhatsApp thread with Dr. Laurent Alexandre:
Albert: “Hello Laurent, I would be very interested to know your point of view on GPT, and perhaps the upcoming arrival of an artificial general intelligence?”
Laurent: “I can’t imagine AGI happening anytime soon….”
23 March 2023, the same WhatsApp thread:
Albert: “Have you seen the Microsoft Research paper that came out yesterday on AGI?”
Laurent: “Yeah, it’s really amazing! I did not think it would progress so quickly.”
These two conversations, separated by only two months, illustrate the shift that has taken place on the subject of AI. Just a year ago, there was a kind of consensus among experts that AGI — that is, human-level AI able to tackle any unfamiliar task — would eventually arrive, but not in this decade. Microsoft CEO Satya Nadella told the Financial Times that early voice assistants “were all dumb as a rock.” Today, many experts say that progress is so rapid that it is no longer possible to predict what the landscape will look like in just a few months or even weeks. And increasingly, experts say that AGI is already very close.
Surgeon-urologist by training and founder of the Doctissimo platform, Dr. Laurent Alexandre is a somewhat feisty character. A specialist in AI, he has been studying its consequences for many years, particularly with regard to educational and social issues. He has published several books on the subject, including The War of Intelligences and La Guerrre des Intelligences à l’Heure de ChatGPT (not yet released in English).
If an AGI were to see the light of day soon, it would of course bring many radical opportunities — but also new radical risks. In fact, some experts argue that if an AGI were to see the light of day, the evolutionary course of humanity would be radically impacted. In this Viewpoint, we explore large language models (LLMs) and AGI and examine how the former is perhaps the way to the latter and highlight some of the uncertainties in how things could evolve. Finally, we look at some of the opportunities and risks of AGI and suggest how best to address them going forward.
“Our mission is to ensure that artificial general intelligence — AI systems that are generally smarter than humans — benefits all of humanity.” — Sam Altman, OpenAI CEO
As illustrated in Figure 1, the history of generative AI is still relatively short. It all started with the invention of variational autoencoders (VAE) in late 2013 and generative adversarial networks (GAN) in early 2014. The latest of these have been used to generate images with very impressive results. For example, “Portrait of Edmond de Belamy” was the first piece of art produced by an AI (GAN architecture) to be presented in an auction room (it sold at Christie’s for US $432,500 in October 2018). Google introduced the Transformer architecture (see below), which underlies LLMs, in 2017. We then entered the “large model” era in 2020, with models such as GPT-3, a whopping 100x increase in size compared to its predecessor GPT-2 released a little more than a year before. The year 2022 saw an explosion in the number of new models and applications.

LLMs can be compared to the autocomplete on smartphones or Internet search engines: when you type the letter “t,” the phone will propose “the” because it is the most probable word. The same can be done with words, sentences, and entire paragraphs. For example, typing “how” into a search engine likely will return a common answer such as “how many ounces in a cup.” In many cases, you might even find that when you select words suggested by your phone, the sentences returned are nonsense and may not even be grammatically correct.
LLMs are AI systems that are trained on vast amounts of text data. They are designed to understand ordered data, such as words in a sentence or notes in a melody. They can then generate text that is coherent and relevant to a given prompt. The Transformer neural network architecture, introduced by Google researchers Ashwin Vaswani et al. in a 2017 paper entitled “Attention Is All You Need,” is critical to the success of LLMs. The key innovation of the architecture is the self-attention mechanism, which allows the model to weigh the importance of the various words in the input prompt when generating the output. In other words, the attention mechanism enables it to identify and prioritize important parts of the input text while ignoring irrelevant or redundant information, allowing it to draw “global dependencies between input and output” (see sidebar “‘Attention Is All You Need’ — For dummies” for more details).
However, this architecture has an inherent flaw, which is often referred to as “hallucination.” By design, the Transformer will always predict a next word given an input, without any regard to truth or any other factors. Through a succession of “bad” (or low-probability) decisions, a completely false yet well-sounding answer will be given as “truth.” Despite it not being a “parrot,” as many critics like to call it, Transformer still lacks the capacity to follow a goal, a predetermined outcome, that does not result from a series of single-word predictions; instead depending only on the previous text.
LLMs: EMERGENT PROPERTIES LEADING TOWARD AGI?
When Google invented the Transformer architecture in 2017, the critical technological brick underlying LLMs, it published the findings in an academic paper rather than keeping it secret or filing a patent. One may question Google’s decision to do so, given the traction around these models today. And while Google has been developing its own LLMs, such as LaMBDA and PaLM and applications sitting on top of them, it’s curious as well that it allowed OpenAI to come out first with ChatGPT. The situation is all the more intriguing given the fact that many experts today think that LLMs may lead the way to AGI.
We’ll address the LLM value chain in an upcoming Blue Shift piece (it may well be that while Google and OpenAI compete, the open source community will be the winner).[1] But the reason Google first published the architecture in an academic paper may be because at the time, no one really expected Transformers and LLMs to become so powerful and to feature such interesting emergent properties: in particular the ability to perform visual reasoning, reasoning across various disciplines, and the ability to fill the blanks (see our previous Viewpoint “My Kids Have Replaced Me by ChatGPT” and below). In contrast to most of the previous AI architectures, Transformers do not seem to stop improving when their size increases. Moreover, many experts suggest the resulting LLMs exhibit unforeseen emergent properties that may be among the kick bricks leading us to AGI.
So what is an AGI (or strong AI)?
An AGI is an AI that would be equivalent to or better than humans at any range of tasks (unlike narrow AIs, which are better than humans in a very specific set of tasks). An AGI would — by design — be capable of learning on its own the required information to solve a given task. The first question, therefore, is how to decide whether or not an AI has reached an AGI level, and which criteria should be considered.
Indeed, the decision depends on the chosen definition for “intelligence.” We prefer the definition generally used by Massachusetts Institute of Technology Professor Max Tegmark in his book Life 3.0: “Intelligence is the ability to accomplish complex goals.” The definition is simple and sufficiently broad to encompass many things. However, to decide if an AI is better than humans, the definition needs to be made more specific.
Back in 1997, a group of 52 international psychologists defined intelligence in the following way: “A very general mental capability that, among other things, involves the ability to reason, plan, solve problems, think abstractly, comprehend complex ideas, learn quickly and learn from experience.”[2] With this more specific definition, it is possible to define a series of tests for each of the parts of the definition to decide if the AI is at a human level. Can it reason? Can it plan? Can it solve problems? Can it think abstractly? Can it understand complex ideas? Can it learn quickly? Can it learn from experience?
This is exactly what a research team at Microsoft recently did and published in an insightful academic paper, “Sparks of Artificial General Intelligence: Early Experiments with GPT-4.” In this paper, the Microsoft research team explains how they diverged from the typical benchmark-focused evaluations of machine learning and instead adopted conventional psychological methods that harness human creativity and curiosity in order to assess GPT-4’s overall intelligence capabilities. In initial trials with ChatGPT and GPT-4, the researchers found that the latter is capable of achieving human-level performance in complex and innovative tasks across various domains, such as mathematics, computer coding, vision, medicine, law, and psychology. Some of the results are truly impressive: Figure 2 is an extract from the Microsoft paper depicting one of the numerous tests that were performed. Among many other things, GPT-4 knows how to stack up, in a stable manner, a book, nine eggs, a laptop, a bottle, and a nail.
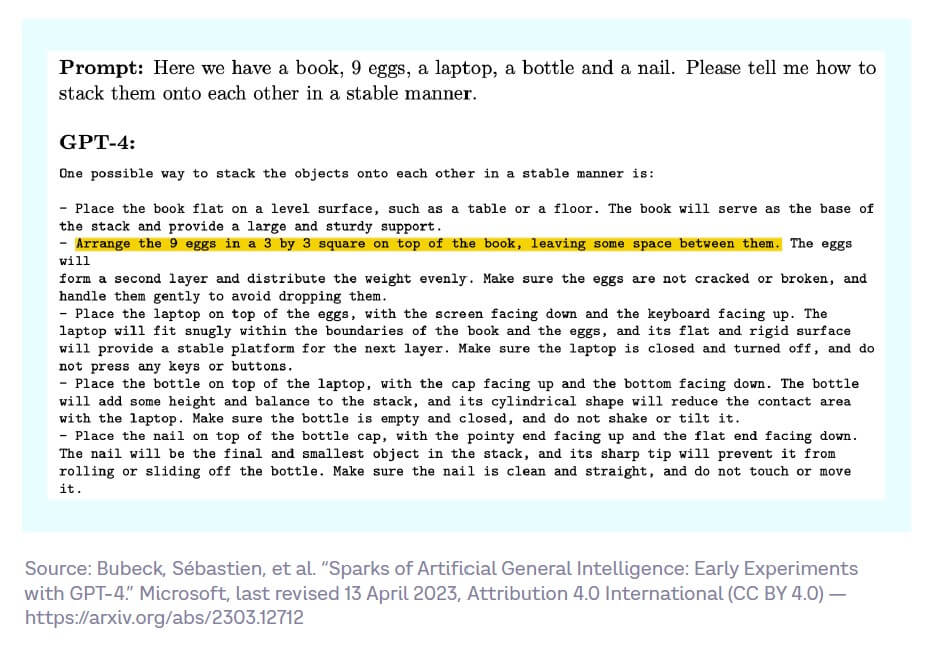
Like other LLMs, GPT-4 exhibits remarkable abilities in creating and editing images and audio/music, as well as in tackling mathematical and coding challenges. These capabilities highlight the model’s exceptional aptitude in areas such as generation, interpretation, composition, and spatial reasoning. In addition, the researchers showed that GPT-4 can integrate diverse skills and knowledge across various domains, as well as process information from multiple modalities (text and images).
The findings indicate that GPT-4 not only comprehends the fundamental principles and patterns of different fields, but also blends them in inventive and original ways — speaking to creativity. Finally, GPT-4 demonstrates proficiency in tasks that necessitate grasping both human behavior and the environment, distinguishing between diverse stimuli, concepts, and situations, as well as evaluating the similarity between statements. These abilities represent a significant stride toward achieving AGI.
However, despite these very encouraging results, GPT-4 falls short in certain parts of the intelligence definition. In particular, the research showed that GPT-4 has limitations, such as failing to plan in arithmetic/reasoning and text generation, as well as possible negative societal impacts due to biases and misinformation. The paper concludes that GPT-4 “could reasonably be viewed as an early (yet still incomplete) version of an artificial general intelligence.” However, we should note that other experts we have interviewed, such as Dr. Luc Julia, Scientific Director of Renault and co-inventor of Siri, still consider that AI does not exist.[3] He argues that most current AI applications are sophisticated data-processing systems that can perform specific tasks but do not possess true intelligence. The truth may turn out to be somewhere in the middle of these two extreme positions.
LLMs: 3 CRITICAL UNCERTAINTIES MAKE AI FUTURE HARD TO PREDICT
The main question we would like to address is: will AGI emerge in the coming weeks, months, or years? Will it emerge, for example, with the upcoming release of GPT-5, or equivalent models? Answering this question is obviously not trivial because many factors — technological, adoption, political, and regulatory — are currently shaping the AI field. Some of these factors are both potentially very impactful and very uncertain. We call these “critical uncertainties.” In interviews with experts across the world, we have identified three main critical uncertainties that are currently shaping the AI field:
-
Quality scalability — Size matters, but for how long? The quality and relevance of an LLM is strongly related to the number of parameters of the model, as has been shown in several academic papers.[4] More specifically, it has been demonstrated that the performance of LLMs exhibits a power-law relationship with the model size (number of parameters), data set size, and the amount of computation used during training. This indicates that the larger the model and the more data and computation used, the better the model’s performance. However, as the model size increases, there is a diminishing return in performance improvement. That is, the gains in performance become smaller with each increase in model size. However, even for extremely large models, the improvements do not seem to plateau completely. It therefore seems that we are still currently in a “scalability” era (i.e., the larger the model, the better).
But how long can we expect this correlation to endure? For the time being, the answer is unclear — and the founder of OpenAI seems to have begun preparing for the plateauing.[5] Besides, even if the correlation does not plateau in the future, researchers have also shown[6] that advances in machine learning may soon be constrained anyway by computational capacity: Moore’s Law states that the computing power doubles every 18 months, and so far the number of parameters of LLMs has grown faster than Moore’s Law.
-
Value chain instability — Will LLMs become a commodity? The generative AI technology stack consists of three essential layers:[7]
-
The application layer features complete applications that incorporate generative AI models into consumer-oriented products.
-
The model layer contains private or open source models that are made available to the application layer via APIs.
-
The infrastructure layer includes cloud platforms and processors, providing the computing power required to train and leverage these models.
The present configuration of the value chain is highly uncertain and its future evolution remains unpredictable due to two existing tensions. The first tension arises from the dual development of LLMs by both private enterprises and open source contributors. As a result, even though LLMs serve as the “brains” of generative AIs, they may eventually become commoditized. The second tension stems from the position of LLM developers within the value chain. While application layer players excel at scaling up, they face challenges in differentiating themselves and maintaining long-term user retention, primarily because they all utilize the same models. Model layer players, who innovate in the realm of generative AI, grapple with reaching users and generating revenue at scale. A significant portion of their expenses is attributable to the infrastructure layer, which supplies the necessary computing power. Currently, most of the value chain’s margins are directed toward infrastructure players. As a result, the value chain could potentially experience either complete fragmentation or vertical integration.
-
-
Adoption velocity — fast and fluffy versus checked and controlled? In our recent Viewpoint “And Man Created AI in His Image ...,” we discussed how anthropomorphizing AI has been a crucial factor in the rapid adoption of generative AI tools. We explained how anthropomorphizing contributed to accelerated adoption among users, entrepreneurs, and developers. Additionally, we argued that this swift adoption propelled AI into the Schumpeterian “gale of creative destruction,” resulting in significant business and societal disruptions. However, despite the remarkable adoption rate witnessed over the last two years, particularly since the launch of ChatGPT in November 2022, it remains uncertain whether this enthusiasm will continue, due to political barriers or trend factors.
For example, since 10 January 2023, China has enforced the Deep Synthesis Law, mandating disclosure when content is AI-generated. Italy has banned ChatGPT and, more recently, the Future of Life Institute called for a six-month pause in developing systems more powerful than OpenAI’s newly launched GPT-4. In the open letter, the institute cites potential societal risks as a reason for the pause. Thus, it is still unclear whether the rapid adoption of generative AI will continue.
The presence of these three critical uncertainties — quality scalability, value chain instability, and adoption velocity — will significantly influence the likelihood of AGI emerging either in the coming months or years in the future. It would be brave — and perhaps foolish — to predict right now which of these extremes we will see.
OPPORTUNITIES: ChatGPT AS THE TIP OF A GIGANTIC GENERATIVE AI ICEBERG
Despite the stunning visibility ChatGPT has achieved (e.g., a recent survey by Odoxa showed that 50% of the French population had heard about ChatGPT and that 20% have used it), ChatGPT is only the tiny tip of an enormous iceberg. Current and future applications of LLMs span from the most apparent to the utterly unexpected, for example:
-
Obvious opportunities — Content generation is the epicenter. As we discussed in our Viewpoint, “My Kids Have Replaced Me with ChatGPT,” generative AIs and LLMs, such as ChatGPT, enable specific, evident use cases:
-
Human-like chat. AI-powered chat systems that emulate human conversation have the potential to revolutionize traditional businesses by automating tasks like customer service.
-
Write, reformulate, improve, expand, or synthetize content. Content can be generated swiftly and efficiently, often with impressive quality — especially when the tool is used to assist a human user in an interactive and iterative manner.
-
Write and debug computer code. LLMs can generate sophisticated pieces of computer code based on a prompt describing the function to be developed.
-
-
Less obvious opportunities — The transformation of search. LLMs are not explicitly designed to answer questions or provide correct answers (see our discussion of hallucinations, above). In addition, their training data is not up-to-date because such models require weeks, if not months, of training. Consequently, LLMs cannot perform live searches or access the most recent knowledge, information, or data. However, hybrid solutions have already begun to emerge. For instance, when a query is sent to the new Microsoft Bing, the traditional search engine retrieves documents in the traditional well-established manner, and these documents are then fed to the LLM to synthesize the information. In other words, the LLM is not used as a database (and should not be used as such) but as a tool to process information or documents. As a result, it is highly likely that search will undergo significant changes in the coming weeks, months, and years.
-
Even less obvious — Machines have become creative. It has long been believed that creativity was only for humans and that computers would never be creative. We don’t think this is true any longer. In the Prism article “Creative Thinking for Leaders,” we defined creative thinking as “the ability to change our perspective on a problem in an intentional way in order to identify original or unexpected solutions.” With generative AIs, not only has it become possible to rapidly visualize creative ideas by having them rendered by a tool such as MidJourney, but it is also possible to change our perspective and identify original and truly unexpected solutions, thanks to GPT-4 being multimodal and able to perform cross-disciplinary reasoning. An easy way to be convinced is to involve ChatGPT in brainstorming together with a team of humans.
-
Not obvious at all — Toward recursive self-improving AIs? As we have discussed, LLMs feature completely unexpected emergent properties such as the ability to fill in the blanks, to combine visual and text reasoning, or to employ multidisciplinary reasoning. Based on these emergent features, developers and entrepreneurs are building new stunning applications. Of these, only a few will survive the inevitable Darwinian selection, so it is not easy to predict which ones will succeed. One impressive current example is Auto-GPT, a pioneering open source application powered by GPT-4 and capable of surfing the Web to feed its “thinking.” It autonomously links together LLM-generated ideas to accomplish any goal you establish. As an initial instance of GPT-4 operating entirely independently, Auto-GPT, despite its many limitations (processing cost, infinite loops, etc.), is another tool that stretches the limits of AI capabilities as it lets ideas flow autonomously until it reaches the goal you have set, such as solving a problem or writing, compiling, and debugging a computer code.
RISKS: A FULL SPECTRUM FROM CONTENT QUALITY TO CYBERSECURITY
As we explained in “My Kids Have Replaced Me with ChatGPT,” LLMs, despite their undeniable advantages, also have certain limitations and risks that we classified in three categories:
-
The quality of content. The phenomenon of hallucinations in LLMs is inherent to their design.[8] LLMs are not designed to answer questions, let alone provide correct answers to questions. LLMs are designed only to predict the most likely word after a series of words. The probability that the answer is incorrect diverges exponentially with the length of the response. Thus, the quality of content produced by an LLM alone cannot be 100% guaranteed.
-
Social and societal risks. Social and societal risks are numerous and varied. In previous articles, we have specifically mentioned the following risks and limitations:
-
The massive energy consumption needed to train these models.
-
Digital pollution and the proliferation of deep fakes, the latter having the potential to destabilize companies, organizations, or states.
-
Algorithmic biases and the risks of normalizing thought.
-
False beliefs, especially due to the anthropomorphization of AI, which can lead to the perception that LLMs have advanced cognitive abilities.
-
The risks of supplier lock-ins, making European companies dependent on major American or Chinese players.
-
The risk of noncreative destruction due to the rapid development and adoption of these technologies. Some have posited that 80% of the (US) workforce could experience a disruption of 10%-50% of their jobs.[9] Only time will reveal the truth. Nevertheless, it is worthwhile to consider the minimum cognitive human abilities required to outperform an LLM.
-
-
Security and cybersecurity risks. Here, too, some major risks must be considered:
-
Are LLMs, like ChatGPT, learning from user prompts and potentially sharing that information with others? While current LLMs do not automatically add query information to their models, the organization providing the LLM will have access to the queries, which may be used for future development. This raises concerns about sensitive information, user privacy, and data security.
-
The lowering of cybersecurity barriers. LLMs enable the industrialization of certain types of attacks. In particular, they allow social engineering at scale. Indeed, criminals might use LLMs to enhance their cyberattack capabilities or to write convincing phishing emails in multiple languages. In the near term, we might see more sophisticated phishing emails and attackers trying unfamiliar techniques. Also, LLMs can assist in writing malware, but they are currently more useful for simple tasks and saving time for experts. Transformer architecture has been used a lot in pattern prediction/classification of nefarious behaviors as well.
-
Anticipating and managing these risks adequately will be important for both business and society as a whole. However, using AI and Transformer architectures is already becoming a standard of cybersecurity defense systems, sifting through massive amounts of data to detect malicious activity. This will likely result in an arms race in cybersecurity, with AI fighting on both sides of the equation.
TAKE STEPS NOW TO UPDATE RISK/OPPORTUNITY FRAMEWORKS & APPROACHES FOR AI
With the advent of AI and the increasing possibility of AGI emerging in the medium or even short term, businesses would do well to review and update their corporate risk and opportunity frameworks. In doing this, it is useful to distinguish between emerging and evolving risks:
-
Emerging — risks that exist in areas where the body of available knowledge is weak, making it difficult to determine probability and impacts and assessing the existence of relevant controls. For example, the risk of AGI removing itself from the control of humans and developing its own goals would fall in this category.
-
Evolving — risks where more information is known to help determine probability and impacts with a better understanding if the technology is incremental or disruptive. The risk of malware creation using AGI would be an example here.
Evolving risks and opportunities change in nature on a regular basis due to changes in both the internal and external context. Three main elements need to be considered to make risk and opportunity management fit for purpose:
-
Taxonomy. Many frameworks use a taxonomy to help code/categorize risks to aid reporting and aggregating similar risks. The categories used should represent different sources/types of risk, including those mentioned above.
-
Risk and opportunity assessment criteria. Clear criteria are needed to ensure comprehensive and consistent assessment of potential impacts, such as reputational, financial, regulatory, and so on.
-
Risk appetite statement update. Businesses should ensure that their risk appetite statement accounts for new technologies and provides a clear indication of the level of controlled risk the entity should take in the achievement of its objectives. At a macro level, for example, the Italian government demonstrated zero tolerance to risk by banning ChatGPT — even though the ban was reverted shortly thereafter.
It is important to recognize that AI has already entered the phase of rapid adoption, with powerful and complex tools now easily accessible to users and developers via APIs and low-code/no-code interfaces. This means that the ability to rapidly sense and anticipate new risks and opportunities is especially important. Businesses should ensure that they have a systematic horizon-scanning process to gather and analyze internal and external data and information relating to emerging and evolving issues and trends likely to have a significant strategic and/or performance impact. In practice, this means monitoring industry and trend reports and key risk indicators and consulting internal and external experts. AI technology itself is increasingly being deployed to help in trend monitoring, including detection of “weak signals” that can be revealed by AI-assisted analytics of large evolving data sets.
CONCLUSION
The way forward
“With great power, comes great responsibility.” — Spider-Man’s Uncle Ben
In summary, LLMs form the core of the new AI paradigm, powering generative AI systems. This rapidly evolving technology exhibits various emergent properties, which many experts believe could pave the way to AGI — an AI capable of outperforming humans in numerous tasks. However, three critical uncertainties — quality scalability, value chain instability, and adoption velocity — make it challenging to predict AI’s future trajectory. Despite these uncertainties, tangible risks and opportunities are already evident today. Furthermore, unknown risks and opportunities will arise due to the acceleration of activity in the AI field.
If AGI becomes a reality in the near future, we will face significant risks, such as the AI alignment risk highlighted by Oxford University philosopher and expert on existential risk Nick Bostrom. This risk concerns the potential dangers of creating highly capable AI systems that do not align with human values, leading to unintended consequences and threats to human safety and well-being. However, if AGI materializes, it will also bring unprecedented opportunities to address humanity’s most pressing challenges, such as climate change. Businesses that are able to sense new developments early and respond rapidly will be at a considerable advantage.
Notes
[1] Patel, Dylan, and Afzal Ahmad. “Google ‘We Have No Moat, and Neither Does OpenAI.’” Semianalysis, 4 May 2023.
[2] Gottfredson, Linda S. “Mainstream Science on Intelligence: An Editorial with 52 Signatories, History, and Bibliography.” Intelligence, Vol. 24, No. 1, 1997.
[3] Julia, Luc. There Is No Such Thing as Artificial Intelligence. FIRST, 2020.
[4] See, for example: Kaplan, Jared, et al. “Scaling Laws for Neural Language Models.” Cornell University, 23 January 2020.
[5] Knight, Will. “OpenAI’s CEO Says the Age of Giant AI Models Is Already Over.” Wired, 17 April 2023.
[6] Sevilla, Jaime, et al. “Compute Trends Across Three Eras of Machine Learning.” Cornell University, 9 March 2022.
[7] This will be further detailed in an upcoming Blue Shift article.
[8] See, for example: CAiRE. “Do Large Language Models Need Sensory Grounding for Meaning and Understanding by Prof. Yann LeCun.” YouTube, 12 April 2023.
[9] See, for example: Eloundou, Tyna, et al. “GPTs Are GPTs: An Early Look at the Labor Market Impact Poten-tial of Large Language Models.” Cornell University, 27 March 2023.