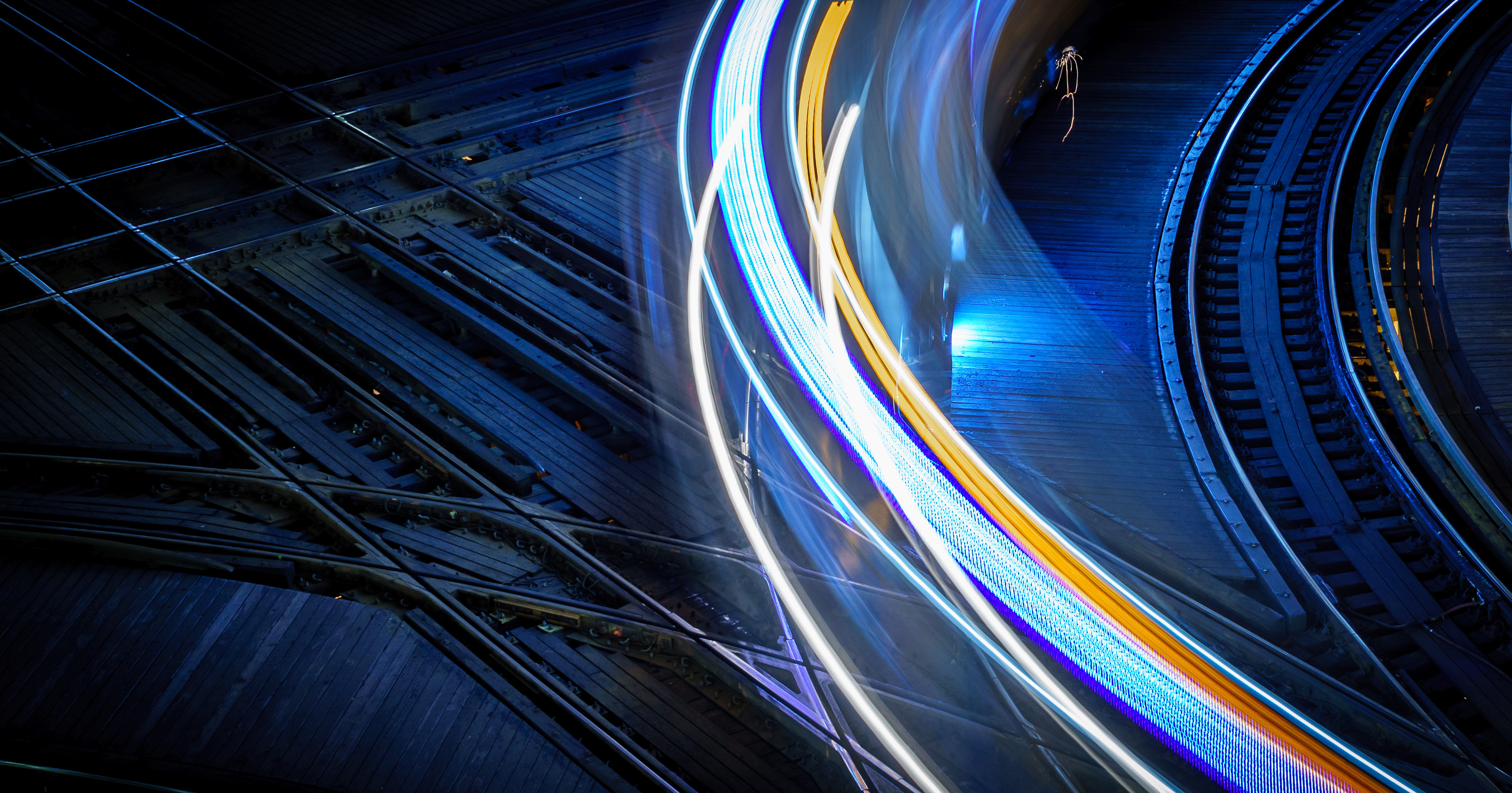
Beyond the hype, where the true value lies
After all the hype, driverless cars guided by artificial intelligence (AI) are still some years away. However, this is far from being the only application of AI in mobility and transport. There is still huge potential for AI to help solve many critical transportation and mobility challenges now and in the future, both in terms of improving effectiveness and efficiency for some modes, such as rail and aviation, as well as in terms of optimizing mobility at the broader system level across modes. So, what are some of the most promising opportunities today and what are the important prerequisites for making progress?
A window of opportunity for radical change in mobility systems
It could be said that AI is now well into the “disillusionment” section of the celebrated hype curve, with previous bullish predictions of transformational impacts in the near term now being revised downwards. Nowhere is this truer than in autonomous vehicles, where safety concerns and negative consumer sentiment have led most observers to predict deployment only in limited and segregated contexts, at least in the short to medium term.
The COVID-19 crisis has certainly not helped – with new trials and launches being postponed or shelved. However, the disruption caused by the pandemic has also had a major upside. It has caused society to realize for the first time that it is possible to drastically change mobility habits, especially in cities.
The pandemic has accelerated many trends that already existed, including more working from home; more use of individual transport modes rather than mass transit; and greater public concerns about health, safety, and environmental sustainability (see our recent major study report, “The Future of Mobility post- COVID-19”).
Because of this acceleration, the next few years offer a unique window of opportunity to make radical change toward the goal of more sustainable, resilient, and human-centric mobility systems.
How AI can help bring about the change
AI has huge potential to help bring about this change, well beyond autonomous vehicles. At a very basic level, AI can do three key things to improve mobility systems (see figure below):
1. Sense – acquiring and interpreting data that can come from the network, such as weather, traffic flows, and crowding.
2. Think – creating insights and predicting future conditions, such as disturbances and congestion.
3. Act – assisting humans in making rapid decisions, such as optimizing routes and adjusting speeds.
Based on these capabilities, let’s look at some opportunities for AI, both in specific applications and to enhance the overall mobility system. AI has the potential to develop increasingly from Sense, toward Think, and eventually to Act.
1. Transport planning & execution – from long-term planning to real-time optimization of flows
AI is already being used to enable much more dynamic and accurate predictions of a territory’s infrastructure needs. One example is the UK’s National Infrastructure Systems Model (NISMOD), which addresses multiple domains, including energy, digital, transportation, and water management. NISMOD deals much better with interdependencies across different types of infrastructure and allows for real-time refreshing of source data. Here, AI is leveraged through the Sense capability using knowledge representation and deep learning.
At the other end of the scale, AI is being used to dynamically optimize transport network control to improve speed and capacity without the need for any physical extensions. One of many examples is Norfolk Southern Corporation in the US, which dynamically optimizes traffic patterns across the rail freight network, enabling train drivers to increase speed by 10%-20%. Leveraging knowledge reasoning through the Think capability allows processing of much higher volumes of real-time data than a human dispatcher could handle.
Another example is Singapore’s Changi Airport, which has a prototype AI-powered system for predicting flight arrival times for long-haul flights with much greater accuracy than was previously obtainable, reaching ~95% of accuracy. Using machine learning and decision making in a Think way enables more responsive and efficient ground-handling operations as well as shorter queues for passengers.
2. Asset lifecycle management – from maintenance to replacement of assets
AI is already being used to diagnose asset condition from automatically captured images and recommend maintenance interventions, improving asset performance and reducing costs and downtime. Deutsche Bahn employs such a system for rolling stock maintenance; there are many applications in other transport modes (e.g., rentals and aircraft maintenance turnarounds).
Leading airports like Seattle-Tacoma International and Gatwick use Assaia’s video recognition and AI in a Think way to improve the security and efficiency of operations. Based on unstructured data from the aircraft, Assaia identifies the tasks to be performed and matches them with maintenance procedures to ensure all tasks are performed in an efficient and secure way across all teams on the tarmac. AI is also being used to augment maintenance operators’ capabilities (e.g., by automatically providing relevant contextual data for a repair or pointing out aspects that may be hard to see with the human eye).
3. Crisis management – from prediction to business recovery
AI’s sensing and predicting capabilities are invaluable for improving system resilience and crisis management. An example is Hong Kong transport operator MTR’s AI-based system that can predict specific types of incidents on tracks up to five days ahead. It draws on weather forecasts, historical incidents, topological data, and current conditions (e.g., leaf color and tree height) to create insights and recommendations to help prevent disruption.
In the automotive sector, Audi cars already collect sensor data to calculate road slipperiness and adapt the suspension to suit. The data is then uploaded to the cloud and broadcast to all Audi cars to warn them of possible hazards ahead. This application of machine learning in a Think way has a major positive impact on road safety.
4. Customer engagement – from communication to personalization
AI is helping to take personalization of the mobility user experience to the next level. For example, in late 2020, GPS navigation app Waze introduced new features to proactively suggest itineraries using personal trip history, localization, and time data. Estimated time of arrival data is now updated also for areas where there are fewer drivers, reflecting the impact that the pandemic has had on historical driving patterns.
Personalization will be increasingly relevant as mobility systems evolve toward greater human centricity and sustainability. Apps such as AxonVibe and MotionTag, which detect and predict real-time traffic patterns, push contextual information only at the right moment and in the right place, which is essential to bring about behavior change.
In airports around the world, AI-powered robots are also being used to improve customer experience. True Act capability is being employed as these robots are continuously trained to perform a wide range of tasks, from cleaning and security to check-in and baggage handling.
5. Autonomous vehicles – from promise to realization
Autonomous vehicles (e.g., in the form of small interconnected pods replacing conventional vehicles) are still the bleeding edge for AI technology. Here, AI is required to continuously and rapidly Act on how vehicles progress along a road. There are several potential benefits, including increased road capacity, lower pollution, less congestion, and improved safety. However, in various trials and pilots over recent years, we have seen the limitations of current technologies and have become more aware of the broader behavioral, societal, and ethical challenges.
Further AI improvements will be needed to better model and predict human movements, and humans also need to better understand how to live with autonomous vehicles.
Moreover, some of the expected benefits, such as reduced congestion and environmental footprint, may not be so easy to achieve. Arthur D. Little recently performed a study on the overall capacity improvement that could be achieved from autonomous vehicles. We concluded that mixed traffic (with 50% non-autonomous conventional vehicles on the road in addition to autonomous vehicles) would actually reduce the overall capacity by around 16% in comparison to a fully conventional situation. The congestion benefits of autonomous driving, a substantial multiplier effect of about 10 times, would be enjoyed only if there was universal deployment of autonomous driving. This is hard to envisage in the foreseeable future and may not be something we would be aiming for. What seems clear is that, whatever the development path will be, autonomous vehicles will need to process even more data to realize their promise in the years ahead. Better AI will be one of the keys to enable this to happen.
The next step for AI – enabling the move toward full multimodal system-level optimization
So, what are the next priorities for AI in mobility? For users, transport authorities, and mobility providers alike, the “holy grail” is to have fully integrated mobility systems with seamless operations and optimization across all transport modes, centered on the needs of the user, resilient to disruption, and environmentally sustainable.
For some years, Mobility-as-a-Service (MaaS), in which users shift from using individual personal transport “by default” in favor of a range of tailored mobility services made accessible by a unified platform and app, has been seen as a key part of this future. Yet virtually all the MaaS pilot schemes tried so far have either failed or fallen short of their objectives. There are several reasons behind these shortcomings, many of which are not related to technology:
- Too much focus on “having a MaaS in place” rather than on improving the travelers’ multimodal experience.
- Development of “generic” MaaS offerings (same value proposition to all, often with low adoption), rather than developing MaaS offerings of most relevance to the specific user groups that would benefit most from them (e.g., young families with children and no car); therefore, driving adoption on the basis of careful user segmentation considering mobility patterns and requirements.
- Insufficient investments in the physical solutions and infrastructure that are critical to effectively improve the experience, beyond the digital components of MaaS.
In our view, one reason behind these shortcomings is that MaaS endeavors have so far mostly focused on either improving the “offer” (through more seamless intermodality) or influencing the demand (through nudging the use of more sustainable modes), but not sufficiently on improving system-level orchestration of the supply and demand.
What would be needed to address this challenge is what we call a Unified Mobility Management Model, in which there is open data sharing and unified governance across all mobility modes (see figure below), allowing for system-level optimization of supply and demand going far beyond “traditional” MaaS solutions.
The model is further supported by a shared public back end, in which common mobility system management, supply, and customer services/experience functionalities are dynamically processed, with decision making informed by suitable algorithms. This covers both goods and people, across all transportation modes. It also includes regulatory enforcement and system-level flow and asset optimization.
At the front end, different mobility service providers continue to interface with the customer, including goods as well as people. The model requires a unified collaborative governance approach to steer the system, involving all system players and user representatives. AI technology is critical in enabling such a model to be deployed, for example:
- To forecast short-term demand and leverage the system digital twin to optimize urban mobility planning.
- To orchestrate demand and supply management measures (e.g., trip-based subsidies), bringing the system closer to its optimum combining dimensions (e.g., system congestion, user adoption, healthy lifestyle, safety, and carbon emissions).
- To suggest, implement, and enforce mobility policies across the system (e.g., dynamically defining no-parking zones based on disappearances of e-scooters, or deriving maximal speeds based on a speed/incident analysis).
- To predict and anticipate user needs and offer a mobility experience tailored to their journey context.
AI has a key role to play but there are important prerequisites for players in the mobility ecosystem
We can conclude that AI certainly has a very promising future in the mobility domain. Already, it is helping to optimize mobility systems, manage and operate assets, improve resilience and crisis management, and personalize mobility services. In time, it has the potential to help realize the promise of autonomous vehicles and enable us to achieve fully integrated, sustainable, resilient, and human-centric mobility systems. However, technology alone will not be enough to bring about the radical changes that are needed. Players in the mobility ecosystem, whether service providers or authorities, public or private, will need to collaborate in new ways. We see three prerequisites for success in AI-based mobility propositions:
1. Choose your battle. Many underlying algorithms and approaches for AI are already available in theory. However, translating these into viable business propositions is not straightforward. Players must carefully select and analyze the business cases they want to implement, otherwise a lot of money can be spent for little impact. They should focus not just on the technology itself, but on the valuable outcomes in terms of Sense, Think, and Act, and whether the various use cases and their benefits can be clearly explained and easily understood by users.
2. Manage your data. For many AI use cases, data is required that may currently be unavailable or not clean. For example, Uber prevents outside APIs to function, restricting access only to those incoming from its own app. Players must ensure that their proposition includes a data supply chain delivering usable data at scale and at speed. Moreover, more personal data being shared and a larger dependency on data, cybersecurity must become a core business. The privacy of data owners is very important and should be guaranteed to achieve high rates of adoption.
3. Govern your ecosystem. Finally, players must collaborate to enable unified governance across the complete ecosystem. In the coming years, we expect city governments and authorities to increasingly stand up and take a more active role in framing and enabling more integrated and virtuous mobility systems. Standards like the Mobility Data Specification for collecting and managing data must be defined, agreed, and enforced. Regulations and circumstances differ by region and even by city, and require a high degree of adaptability. Safeguards will be needed to enable human intervention and control when necessary.
We are still at the foundational level in what AI can mean for mobility and the transport industry. The examples shown here demonstrate that major benefits are achievable, provided that the ecosystem players can work together to overcome the challenges.