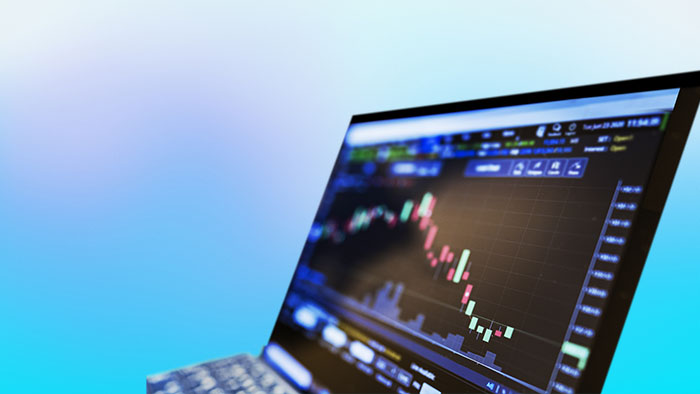
This is commonly due to a mismatch between technology and business needs, along with a failure to focus on culture and governance in data management projects. Closed company cultures, in particular, make interaction between departments difficult, which often prevents the collection, sharing, and effective use of comprehensive data. This Viewpoint outlines how smart data management and a business-focused approach deliver proven, immediate benefits while unlocking future opportunities for growth.
Transforming data into a strategic asset
Attempts to harness data across organizations and then turn it into a business asset are not new. However, data often remains stuck in application silos, stored across the business with no transparency on information availability and little sharing. Often, data is being generated, but not stored or used. Learning from failed projects, there is a growing realization of the importance of a structured approach to data management, moving away from the dispersed, siloed, and departmental methods in place.
Effective data management enables better decision making, delivering clear benefits in four key areas:
- Internal efficiencies – driving process improvements, operational excellence, and cost savings.
- External customer excellence – ensuring a greater customer focus and the delivery of digital, innovative, and personalized service offerings and solutions for customers.
- Driving innovation and new opportunities – underpinning the development of new services, new revenue streams, and the effective introduction of artificial intelligence (AI). Without accurate, high-quality data, AI projects fail to deliver.
- Enabling digital transformation – providing a foundation for successful digital transformation.
Organizations can learn from their experience of introducing process management and the benefits this delivers. Enforcing standard processes across the entire company and within departments transforms performance and builds a scalable platform for both efficiency and innovation. Smart data management delivers a similar step change. It drives both agility and operational improvements, helping create ambidextrous organizations that can thrive in today’s competitive markets and transform themselves going forward (see Prism article, “Ambidextrous organizations”).
The barriers to smart data management
Given the clear benefits, why is the potential of data not being unlocked? For years organizations have invested heavily in data management but have yet to realize value from their projects. There are five common reasons for failure:
- Business models. Most data-driven business models die in the embryonic phase, as they do not deliver sufficient value in the eyes of stakeholders.
- Data collection. A technical focus on creating a single, company-wide data lake increases costs and is too complex to meet business needs.
- Inability to scale. Initial successes and quick wins may deliver results but the approach does not scale across the business.
- Data quality issues. Access to data is more difficult than planned, and the data itself is not high enough quality for successful usage.
- Cultural issues. People and culture are not considered as a key part to data management projects; this prevents data sharing or cross-departmental projects.
The data maturity curve
Before implementing a data management strategy, it is essential that organizations understand their level of data maturity (see Figure 1). Carrying out a maturity assessment of strategy, governance, and execution provides a starting point on the data journey and focuses activities where they will deliver best value. Today, most companies are at Step 2 (Supportive). They know that data is important and will help their businesses thrive, but they lack the vision, roadmap, or management expertise needed to successfully manage and benefit from it.
While moving forward to the Prescriptive stage cannot be realized overnight, companies typically can achieve this level within two to four years, depending on company culture and readiness for change. However, organizations don’t need to be fully mature to begin to get value from their data — success comes from moving forward on a clearly mapped program of smart data management.
Adopting a smart data management approach
Overcoming the challenges to data management and increasing data maturity requires organizations to adopt a more holistic approach. That means treating data management as a business and cultural program, not just focusing on the technology that handles the data. Businesses need to put in place a clear scaling process that enables them to learn from proofs of concept and drive wider adoption. Thus, overcoming data quality challenges requires the implementation and enforcement of global data governance concepts, including clearly setting respective roles and responsibilities.
Essentially, smart data management involves taking a full lifecycle approach (see Figure 2) covering both upstream access to data and downstream generation of insights and value.
Upstream
−Create and collect – identify sources and gather data.
−Validate and clean – manage and monitor data quality through agreed-upon, company-wide standards.
−Store and share – make data available in the right formats to the right people.
Downstream
−Define – enhance data and identify business needs and use cases.
−Develop – provide data science capabilities and build skills.
−Scale – go beyond single use cases to integrate data into business operations.
Turning strategy into results
To unlock the value in data across the company, organizations should put in place a framework with concrete steps that starts with the business and its needs.
1. Business needs
Begin by defining how data can support corporate strategy. What are the use cases, including AI-enabled use cases, for creating new, digital products and services (generating new revenues) and improving existing operations (enabling cost savings)?
2. Strategy
Build on these needs to create the data management vision, addressing potential challenges such as data sharing up front. Translate this to the target picture, providing an ambitious description of the desired impact of the data management strategy, including people and culture as well as technology. Set clear, straightforward data management principles to guide all activities.
3. Governance
Create defined managerial processes, focused on strategic, governance, and operational results. This should include roles and responsibilities, quality standards, a common glossary of terms, and reporting frameworks.
4. Execution
Put governance and strategy into action. In addition to building a data map and management capabilities, focus on data security and compliance. Ensure you have the data science and analytics capabilities to support your use cases. Protecting the data and interests of customers and employees is not just a regulatory imperative but builds trust and can unlock opportunities for differentiation against rivals. Only in this phase should organizations choose and deploy the IT architecture, systems, and tools.
CONCLUSION
DRIVING SMART DATA SUCCESS
Harnessing data and turning it into a strategic asset is essential for organizations in an increasingly digitized world. Success involves moving beyond technology —taking a business-centric approach built on concrete use cases (including AI-driven projects) that allows for centrally governed data to avoid duplication or data silos. Organizations need to move beyond “big bang” projects to focus on smaller, more modular data services —effectively creating data pools rather than expensive and complex data lakes. Above all, the approach needs to involve the entire company —sharing responsibility, breaking down silos between departments, and encouraging a data culture across the organization. This will deliver the benefits of effective data management both internally and externally, enabling innovation, greater efficiency, and digital transformation.